NHR Compute Time Projects
Current NHR compute time projects on Alex and Fritz at NHR@FAU
Humanities and Social Sciences | Life Sciences | Natural Sciences | Engineering Sciences | Completed NHR compute time projects
Humanities and Social Sciences
Linguistics
Abstract
This NHR project is tied to an existing DFG/AHRC-funded joint project by FAU Erlangen-Nürnberg and the University of Oxford studying the mechanisms used for disinformation, in particular viewpoint manipulation, by Russian state-sponsored media. The purpose of this sub-project is to use the dataset created in the first stage of the project (NHR-project Pose-22) to build a multimodal transformer that allows us to improve performance of downstream tasks such as gesture detection, segmentation and annotation.
Scientific field: 104-04 Applied Linguistics, Experimental Linguistics, Computational Linguistics
Principal Investigator: Prof. Dr. Peter Uhrig, Friedrich-Alexander-Universität Erlangen-Nürnberg
Target systems: parallel computer Fritz & GPGPU cluster Alex
Literary Studies
Abstract
This project is a collaboration between computer science and computational literary studies (CLS), an emergent field which analyses larger collections of literary texts using a wide set of tools from computational linguistics, computer science and its own tradition. In our project, we focus on the computational literary analysis of character as one of the most important descriptors of narrative and dramatic texts. Our project will investigate the textual description of character’s internal and external features, actions and further character specific information using knowledge induced language models. We aim to create a character knowledge graph through extracting character information from text, find different character types through data-driven clustering, and leverage this information to develop a character-attentive, “literary” language model (“LitBERT”) for automatic literary analysis. The project will significantly advance the state of the art in the combination of language models and knowledge graphs, showing how to improve the performance of language models for the analysis of entities and their attributes by (a) integrating knowledge graphs and (b) enriching domain specific knowledge graphs based on text analysis using language models. Additionally, we want to improve the handling of longer texts like novels by advancing the capabilities of language models to represent knowledge, like representation and types of characters in the text world (i.e., the world described in the text).
Scientific fields: 409 Computer Science; 105 Literary Studies
Principal Investigator: Prof. Dr. Fotis Jannidis, Julius-Maximilians-Universität Würzburg
Target systems: GPGPU cluster Alex
Life Sciences
Basic Research in Biology and Medicine
Abstract
Proteins offer an exciting path to address a multitude of biotechnological challenges. Functionally encoded by amino acid sequences and capable of working under non-toxic, mild conditions, their controllable design has been pursued for decades. Even though Artificial Intelligence (AI)-based models have recently made giant strides in computational protein design, it is evident that we cannot yet design proteins as efficient as those present in nature. To gain a technological advantage in a world with pressing demands in sustainability and healthcare, we must improve and accelerate the development of tailored, proficient proteins.
In this project, I aim to develop a multi-task intelligent agent capable of efficiently engineering functional proteins tailored to user-defined specifications. To achieve this goal, the agent will be trained using available sequence, structural, functional, and dynamic data to perform multiple protein engineering tasks. The model will be freely accessible through user-friendly interfaces, empowering researchers worldwide with an easy-to-use tool for tailored protein design.
Scientific fields: 201-07 Bioinformatics and Theoretical Biology; 409-05 Interactive and Intelligent Systems, Image and Language Processing, Computer Graphics and Visualisation
Principal Investigator: Dr. Noelia Ferruz, Universität Bayreuth
Target system: GPGPU cluster Alex
Abstract
Inflammation plays a crucial role in fighting infections, but its magnitude and duration must be tightly controlled to prevent tissue damage. Immunoglobulin G (IgG) antibodies have both pro-inflammatory and anti-inflammatory effects. Their interaction with cellular Fcγ-receptors (FcγRs) and activation of the complement pathway play a critical role in immune cell activation. Recent studies have shown that IgG can also suppress inflammation through interactions with C-type lectin receptors. The macrophage inducible C-type lectin (Mincle) receptor is induced on macrophages upon activation via microbial infections or tissue damage. Mincle is involved in immunity to mycobacteria, it is upregulated in patients with rheumatoid arthritis, and Mincle sig- naling has been connected to cancer progression. Similar to activating Fcγ-receptors, Mincle signals through binding to the common signaling adaptor molecule, the FcRγ-chain. Binding of Mincle and activating FcγRs to FcRγ-chain is mediated by their transmembrane domains, and competition of Mincle and FcγRs for FcRγ could regulate immune cell activation. Importantly, the interaction between Mincle, FcγRs and the FcRγ-chain depends on receptor localization within the plasma membrane. Our research aims to investigate the molecular crosstalk be- tween Mincle, activating FcγRs, and the FcRγ-chain within the heterogenic environment of the plasma membrane of immune cells. We will explore the competitive binding of activating FcγRs and of the C-type lectin receptor Mincle to the common FcRγ-chain, characterize the receptor-specific lipid environment and their impact on receptor dimerization, receptor cluster- ing and signaling, and the modulation of autoantibody-driven inflammation. By understanding these mechanisms, we aim to enhance our understanding of the complex mechanisms under- lying Mincle-dependent modulation of autoantibody-driven inflammation on different immune cell subtypes.
Scientific fields: 201-02 Biophysics; 204-05 Immunology
Principal Investigator: Prof. Dr. Rainer Böckmann, Friedrich-Alexander-Universität Erlangen-Nürnberg
Target system: GPGPU cluster Alex & parallel computer Fritz
Abstract
The ATPase p97 is a hexameric protein complex that pulls out other proteins from complexes or membranes for further processing, e.g. degredation. Several cryo-EM structures of p97 in different states of function have been published in the last decades, providing a basic understanding of its mechanism. During pulling, the substrate is pushed through a pore in the center of p97 by moving its subunits in a ladder motion using the energy released from ATP hydrolysis. This coordinated motion requires sophisticated information transfer within p97. This allosteric signaling is not yet understand and the aim of this project is to formulate a hypothesis through studying the collective coupled motions with molecular dynamics simulations.
Scientific field: 201-01 Biochemistry
Principal Investigator: Dr. Martin Kulke, Technical University of Munich
Target system: GPGPU cluster Alex
Abstract
Efficiently inducing tailored responses to specific stimuli is pivotal in the development of protein-based nanoswitches for diverse medical and biotechnological applications. This study aims to elucidate the conformational dynamics underlying protein switching behavior upon interaction with target molecules, thereby advancing our ability to engineer functional nanoswitches. Leveraging the periplasmic binding protein (PBP) superfamily as a well-established model system due to its innate ability to bind diverse small molecules, we employ cutting-edge global and local stability design algorithms. Through a combination of biophysical techniques and computational simulations, we investigate the dynamics of various PBP designs. This integrated approach offers insights into the mechanisms governing protein conformational rearrangements, facilitating the rational design of responsive biosensors and other innovative nanotechnologies.
Scientific fields: 201-01 Biochemistry; 201-07 Bioinformatics and Theoretical Biology
Principal Investigator: Prof. Dr. Birte Höcker, Universität Bayreuth
Target system: GPGPU cluster Alex & parallel computer Fritz
Abstract
Metastasis, the spread of cancer cells from a primary tumor to distant sites, is a major cause of cancer-related mortality. A crucial process in metastasis is the disruption of cell adhesion, enabling cancer cells to detach and invade surrounding tissues. This transition involves the upregulation of N-cadherin, enhancing cell motility and invasiveness. On a molecular level, cadherins are calcium-dependent adhesion proteins with five extracellular (EC) domains, facilitating adhesion through homotypic trans and cis interactions. Glycosylation of cadherins modulates these interactions, impacting protein folding, trafficking, and dynamic shielding. In cancer, dysregulated glycosylation networks contribute to altered adhesive behaviors, promoting metastasis by affecting cadherin-mediated cell-cell adhesion and increasing cell migration.
Scientific fields: 201-02 Biophysics; 201-04 Structural Biology
Principal Investigator: Dr. Isabell Louise Grothaus, Universität Bremen
Target system: parallel computer Fritz & GPGPU cluster Alex
Abstract
Bacterial antimicrobial resistance (AMR) is a major public health threat, calling for new therapy concepts. A requirement for effective antibiotics is the accessibility of the target structure. To do this, the antibiotics usually must pass through the bacterial envelope, often consisting of two membranes. The aim of the proposed project is to use membrane-active peptides to influence bacterial membranes such that (i) classical antibiotics become more effective, and (ii) substances with a high affinity for the target structure but a low or even non-existent membrane permeability can efficiently reach their target. This interaction between peptides and bacterial membranes will be characterized using molecular dynamics (MD) simulations.
Scientific field: 201-02 Biophysics
Principal Investigator: Prof. Dr. Rainer Böckmann, Friedrich-Alexander-Universität Erlangen-Nürnberg
Target system: parallel computer Fritz & GPGPU cluster Alex
Abstract
Bitter and sweet taste modalities are mediated by chemosensory receptors, which constitute interesting subgroups within the superfamily of G protein-coupled receptors (GPCRs). Bitter taste receptors are 25 in human and are classified as class A GPCRs for their architecture, while a heterodimer of two class C GPCRs, TAS1R2 and TAS1R3, specifically recognize sweet taste molecules. Sweet and bitter receptors recognize chemically diverse molecules. The proposed project focuses on peptide and protein modulators. Particularly, we aim to investigate the dynamics of sweet and bitter taste receptors in complex with peptide modulators.
Scientific field: 201-02 Biophysics
Principal Investigator: Prof. Dr. Antonella Di Pizio, Technical University of Munich
Target system: GPGPU cluster Alex
Abstract
The Calcium sensing receptor (CaSR) is a class C GPCR, a homodimeric heptahelical membrane protein with am extracellular venus-fly-trap (VFT) domain and a cystein-rich region (CRR). CaSR senses calcium, physiologically present in millimolar concentration range. However, how the extracellular region senses concentration differences in these high ranges and it appropriately mediates receptor activation remains unknown. In light of new Cryo-EM structures of the CaSR, we intend to explore VFT/CRR activation dynamics by permutating occupancy of binding sites, and further simulate the full-length and G-protein bound CaSR dimer. Additional analysis by e.g. allosteric network analysis of this multi-regulated system featuring various allosteric modulators, helps to uncover the activation dynamics of the CaSR.
Scientific fields: 201-02 Biophysics; 201-04 Structural Biology
Principal Investigator: Prof. Dr. Peter W. Hildebrand, Freie Universität Berlin
Target system: parallel computer Fritz & GPGPU cluster Alex
Abstract
ORmd aims to explore the dynamics of all the odorant receptors (ORs). To achieve this, we will employ cutting-edge High-Throughput Molecular Dynamics simulations starting from receptor models in the active and inactive states. This work will allow us to generate for the first time a database of OR structures and molecular dynamics, shedding light on this large but unexplored GPCR subfamily. The analyses of the trajectories will allow us to outline structural features relevant to the activation, which will be common to all ORs or specific for some subgroups.
Scientific field: 201-02 Biophysics
Principal Investigator: Prof. Dr. Antonella Di Pizio, Technical University of Munich
Target system: GPGPU cluster Alex
Abstract
This project focuses on investigating the dynamics of proton-coupled cation permeation in ion channels, with a specific emphasis on comprehending how pH influences the channel’s cation conduction and selectivity. Our research falls under the framework of CRC1078, which aims to enhance our understanding of protonation dynamics in protein function by fostering collaboration among various experimental and computational groups. Within this project, we have set two primary objectives:
(i) To study the Influenza A M2 proton channel, a pH-activated viroporin known for exhibiting both proton and cation transport activities.
(ii) To investigate CNG channels, which are non-selective cation channels that play a pivotal role in the signal transduction pathways of vision and olfaction.
Our approach involves the utilization of classical molecular dynamics simulations and constant-pH simulations to model the protonation patterns of titratable residues within the channel pore and the cation conductance across various protonation states. We have used similar approach before to study AMPA receptor and K2P channels.
Scientific field: 201-02 Biophysics
Principal Investigator: Prof. Dr. Han Sun, Freie Universität Berlin
Target system: GPGPU cluster Alex
Abstract
DNA damages are a constant threat to the integrity of the genome. Damages of the DNA bases can arise from oxidation or aberrant methylation. Deamination of cytosine bases, for example, results in U:G mismatches which ultimately lead to mutations in the encoded protein. The Base excision repair system (BER) is a machinery of enzymes, recognising and removing damaged/wrong bases and replacing them with the correct one. While most base excision repair glycosylases usually only excise the base, it was shown that some also have lyase activity and thereby circumvent AP endonuclease which is otherwise responsible for this second step of the repair mechanism. This project aims for revealing the mechanism of this strand incision, how this relates to the rather narrow specificity of glycosylases with dual activity compared to the broader set of substrates in mono-functional glycosylases.
Scientific field: 201-02 Biophysics
Principal Investigator: Prof. Dr. Petra Imhof, Friedrich-Alexander-Universität Erlangen-Nürnberg
Target system: GPGPU cluster Alex
Abstract
The AmPeL project seeks to advance our understanding of the novel antimicrobial peptide lugdunin by characterizing its interaction with various bacterial and eukaryotic membrane models. Specifically, the project aims to elucidate the mechanisms of action underlying lugdunin’s antimicrobial properties against bacteria, as well as investigate the reasons for its lack of activity against erythrocytes. By shedding light on the precise molecular interactions between lugdunin and different membrane types, this research could ultimately facilitate the development of new antimicrobial therapies that are both effective and safe for human use.
Scientific field: 201-02 Biophysics
Principal Investigator: Prof. Dr. Rainer Böckmann, Friedrich-Alexander-Universität Erlangen-Nürnberg
Target system: parallel computer Fritz & GPGPU cluster Alex
Abstract
The function of proteins is determined by their amino acid sequence and tertiary structure, but nevertheless the particular function of most proteins is unknown. In the Dynasome project we explore to what extent protein function can be predicted by protein dynamics, and explore the space of protein dynamics in general. To this aim, we perform molecular dynamics simulations for a large set of 200 proteins. We analyze these simulations, using e.g. Markov state models, to capture a ‘dynamics fingerprint’ of the studied proteins. We suggest these dynamics fingerprints as a new tool for protein function prediction and for quantitative comparison of protein dynamics.
Scientific field: 201-02 Biophysics
Principal Investigator: Prof. Dr. Helmut Grubmüller, Georg-August-Universität Göttingen
Target system: parallel computer Fritz
Project Report (06/2023)
In the Dynasome3 project, we studied a set of 50 different proteins, all of which perform different specific motions that we probed using molecular dynamics simulations. Some of these specific motions are similar, whereas others are very different. Unfortunately, these similarities and dissimilarities are not obvious from looking at the simulations directly, so instead we use this set of simulations to develop methods that accurately capture the protein-specific dynamics and allow for quantitative comparison between them. Ultimately, by using additional simulations and refined analysis methods, we can gain insight into the world of protein dynamics and, because protein dynamics are generally related to their function, improve protein function prediction.
Abstract
Using Molecular Dynamics (MD) simulations, it is possible to follow conformational changes in proteins at atomic resolution and at high time resolution. MD simulation studies can supplement experimental approaches that typically allow only the structural characterization of initial or final or average structures. Especially in case of conformational switching processes such as binding induced folding an understanding of the process requires the analysis of intermediate states and driving forces for conformational changes. We apply MD-simulations and advanced sampling techniques to understand the molecular details of conformational switching processes within the SFB1035 (“Control of protein function by conformational switching”). One focus is on the transition of a DNA binding domain from a partially unfolded state to a folded state upon binding to DNA. The simulations will be used to define arrangements at which the partners start to influence the conformational switching process and to identify associated energy barriers. As a second system we study the process of collagen folding which is process that involves the coupled folding of three peptide strands and association to form a stable triple helix conformation. The general goal of our simulation studies is to understand at atomic detail how a protein or DNA surface or a specific modification of a protein can help to promote transitions towards a folded conformation of a binding partner or protein segment.
Scientific field: 201-02 Biophysics
Principal Investigator: Prof. Dr. Martin Zacharias, Technical University of Munich
Target system: GPGPU cluster Alex
Abstract
With the incredible growth in RNA therapeutics, lipid nanoparticles (LNPs) have become an indispensable tool to deliver RNA to target cells thereby providing promising perspectives to combat life-threatening diseases such as Amyloidosis or COVID-19. We use all-atom molecular dynamics simulations to resolve the structure of these clinically relevant particles and proved molecular insights how the RNA cargo is distributed inside the LNPs. The structural information gained from our simulations will help to guide the design of LNPs with improved properties.
Scientific field: 201-02 Biophysics
Principal Investigator: Prof. Dr. Nadine Schwierz, Augsburg University
Target system: parallel computer Fritz & GPGPU cluster Alex
Abstract
GPCRs constitute the largest protein family in the human genome. This genome-encoded protein repertoire of about 1000 receptors is expressed in a tissue- and organ-specific manner and transduces a large variety of extracellular signals into the cell. Whilst key residues determining coupling specificity of G proteins have been localized at the Gα-C terminus (GαCT), e.g. of Gs or Gi or at the finger loop of arrestin, a common sequence motif of GPCRs responsible for specific recognition of different GαCT or finger loops has not been detected yet. The R*-Gs/i/o arrestin complexes resolved so far do not provide a clear explanation for G protein coupling specificity. Evidence from several sources suggests the existence of transient complexes between the R* and GTP- bound G protein that may represent several novel intermediates on the way to the formation of GαsGTP and may contribute to coupling specificity.
Scientific fields: 201-07 Bioinformatics and Theoretical Biology; 201-02 Biophysics
Principal Investigator: Prof. Dr. Peter W. Hildebrand, Universität Leipzig
Target systems: parallel computer Fritz & GPGPU cluster Alex
Project Report (02/2023)
Heterotrimeric Gαβγ proteins are guanine nucleotide-binding proteins consisting of alpha (α), beta (β), and gamma (γ) subunits. Gαβγ proteins are activated by G protein-coupled receptors (GPCRs), the largest family of membrane receptors sharing a common seven transmembrane helix (TM) structure. Essential fundamentals of GPCR signaling at the molecular level are now considered to be well understood: Upon binding of extracellular ligands, the receptor undergoes a conformational change that opens up an intracellular pocket to which the G protein can bind. Formation of the ternary ligand-receptor-G protein complex goes along with a conformational change in the Gα subunit that ultimately triggers the exchange of GDP for GTP. For over a decade GPCRs signaling through G-prortein were over simplified looking only at the nucleotide free state. To extend our knowledge on the structural events of GPCR signaling at atomic-level details, we have used all-atom molecular dynamics (MD) simulations combined with time-resolved cryo-EM to explore dynamics of the agonist bounds GPCRs complexed with G-protein in either nucleotide bound (GTP or GDP) or nucleotide free state.
Project Report (09/2023)
Heterotrimeric Gαβγ proteins, comprising alpha (α), beta (β), and gamma (γ) subunits, are key players in G protein-coupled receptor (GPCR) signaling. GPCRs represent the largest family of membrane receptors, characterized by a common seven-transmembrane helix (TM) structure. The basic principles of GPCR signaling at the molecular level have long been established: extracellular ligand binding induces a conformational change in the receptor, creating an intracellular pocket for G protein binding. This leads to the formation of a ternary ligand-receptor-G protein complex, accompanied by a conformational change in the Gα subunit, ultimately promoting the exchange of GDP for GTP. However, this over-simplified view of GPCR signaling has evolved in light of current research endeavors. Our approach combines all-atom molecular dynamics (MD) simulations with innovative experimental techniques such as time-resolved cryo-electron microscopy (cryo-EM) to explore the intricate dynamics of GPCRs complexed with G-proteins. Our investigations encompass agonist-bound GPCRs in conjunction with G-proteins, considering both nucleotide-bound (GTP or GDP) and nucleotide-free states. Our research seeks to extend the boundaries of GPCR signaling by providing atomic-level insights into the dynamic interplay between GPCRs and G-proteins across various nucleotide states. Through the integration of computational and experimental methodologies, we aim to unravel the conformational changes and molecular events governing these interactions. This approach promises to refine our comprehension of GPCR signaling and its intricacies, potentially offering novel avenues for drug discovery and therapeutic intervention.
Abstract
Thymine DNA glycosylase (TDG) is an important enzyme involved in DNA repair, which removes mispaired or modified DNA bases and thus ensures genetic integrity. We have investigated possible reasons for its substrate specificity in our previous work and we now intend to extend the range of possible forms of the substrates to achieve a deeper understanding of the situation in the protein substrate complex prior to the chemical reaction. For this reason, we will investigate the possible role of imino-tautomeric forms of the damaged DNA bases flipped out into the enzyme active site as well as the effect of different protonation states of the substrate bases and an important histidine residue in the binding pocket.
Scientific field: 201-02 Biophysics
Principal Investigator: Dr. Frank Beierlein, Friedrich-Alexander-Universität Erlangen-Nürnberg
Target system: GPGPU cluster Alex
Project Report (07/24)
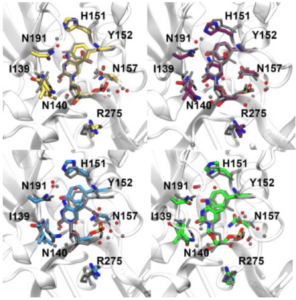
Thymine DNA glycosylase (TDG) is an important enzyme involved in DNA repair, which removes mispaired or modified DNA bases and thus ensures genetic integrity. We previously investigated possible reasons for its substrate specificity, both in the intrahelical (flipped-in) and in the extrahelical (flipped- out) state of the damaged bases 5-carboxylcytosine, 5-formylcytosine, 5-hydroxymethylcytosine and 5-methylcytosine. We showed that imino tautomers probably do not contribute to recognition in the intrahelical state, and that recognition most likely takes place once the bases are flipped out into the TDG binding pocket (extrahelical complexes). Here, only amino tautomers of the bases of interest were investigated.
In the current funding period we extended the range of possible forms of the substrates to achieve a deeper understanding of the situation in the protein substrate complex prior to the chemical reaction. For this reason, we investigated the role of imino-tautomeric forms of the damaged DNA bases flipped out into the enzyme active site as well as the effect of different protonation states of the substrate bases and an important histidine residue in the binding pocket.
The results obtained in the current funding period agree well with our previous work and the experimental data, and also indicate that imino tautomers might play a role in extrahelical recognition and as a starting point in the actual chemical reaction, and will be published shortly.
To complete the picture of the extrahelical recognition in TDG-substrate complexes, it would be highly desirable to obtain more insight into the role of the individual residues in the TDG binding pocket. A series of mutations are known that render the enzyme active/inactive for certain substrates. We plan to investigate this in more detail in a follow-up proposal which is currently being prepared by us.
Abstract
Broadly neutralizing antibodies that bind to viral fusion proteins represent a promising strategy for protection from viral infections. Such antibodies can be used for passive immunization and are currently tested in clinical trials, but they are expensive and difficult to produce. As an alternative, antibody-derived peptides may be used for this purpose. In the present project, the complexes between antibodies and the viral fusion proteins from HIV-1 and CoV-2 are analyzed to identify energetic hot-spots of the interaction. This information will be used for the design of antibody-derived peptides that bind to viral fusion proteins thereby blocking viral infection. For that purpose, a computational pipeline is developed that uses molecular dynamics (MD) simulations to identify the most promising peptides for further experimental testing.
Scientific fields: 201-02 Biophysics; 204-04 Virology
Principal Investigator: Prof. Dr. Heinrich Sticht, Friedrich-Alexander-Universität Erlangen-Nürnberg
Target system: GPGPU cluster Alex
Project Report (04/2024)
Neutralizing antibodies that bind to viral fusion proteins represent a promising strategy for protection from viral infections. Such antibodies can serve as templates for the generation of peptides, which retain the ability to bind to viral proteins. We have screened more than 1300 antibody-antigen complexes from HIV-1 and SARS CoV-2 proteins to identify energetic hot-spots of the interaction. The energetically most favorable peptide stretches were selected for further computational characterization and experimental binding studies. Molecular dynamics simulations allowed to identify structural features that differ between binding and non-binding peptides. In particular, the formation of rather stable binding-incompetent conformations seems to be a major reason for the inability to bind. This information will now be used for an improved identification of peptides, which retain antibody-like binding properties.
Publications
M. Deubler, L. Weißenborn, S. Leukel, A. H. C. Horn, J. Eichler, and H. Sticht: Computational Characterization of the Binding Properties of the HIV1-Neutralizing Antibody PG16 and Design of PG16-Derived CDRH3 Peptides. Biology 12(6), 2023. DOI: 10.3390/biology12060824.
Microbiology, Virology and Immunology
Medicine
Abstract
The DFG research group FOR2886 aims to unravel the causes of rheumatoid arthritis (RA) and seeks to understand early events contributing to the onset of inflammation. The main focus here is to understand the influence of the natural bacterial flora or the microbiome as well as a number of environmental factors (including nutrition and salt consumption) on our immune system and in particular their impact on immune tolerance. In subproject B5 cutting-edge intravital microscopy and 3D volume imaging are combined with state-of-the-art deep learning algorithms to map the cellular and molecular landscape of the synovial tissue during onset of inflammation.
Scientific fields: 205-18 Rheumatology; 409 Computer Science
Principal Investigator: Prof. Dr.-Ing. Katharina Breininger, Julius-Maximilians-Universität Würzburg
Target system: GPGPU cluster Alex
Abstract
As one of the most important aspects of diagnosis, treatment planning, treatment delivery, and follow-up, medical imaging provides an unmatched ability to identify disease with high accuracy. As a result of its success, referrals for imaging examinations have increased significantly. However, medical imaging depends on interpretation by highly specialized clinical experts and is thus rarely available at the front- line-of-care, for patient triage, or for frequent follow-ups. Very often, excluding certain conditions or confirming physiological normality would be essential at many stages of the patient journey, to streamline referrals and relieve pressure on human experts who have limited capacity. Hence, there is a strong need for increased imaging with automated diagnostic support for clinicians, healthcare professionals, and caregivers.
Machine learning is expected to be an algorithmic panacea for diagnostic automation. However, despite significant advances such as Deep Learning with notable impact on real-world applications, robust confirmation of normality is still an unsolved problem, which cannot be addressed with established approaches.
Like clinical experts, machines should also be able to verify the absence of pathology by contrasting new images with their knowledge about healthy anatomy and expected physiological variability. Thus, the aim of this proposal is to develop normative representation learning as a new machine learning paradigm for medical imaging, providing patient-specific computational tools for robust confirmation of normality, image quality control, health screening, and prevention of disease before onset. We will do this by developing novel Deep Learning approaches that can learn without manual labels from healthy patient data only, applicable to cross-sectional, sequential, and multi-modal data. Resulting models will be able to extract clinically useful and actionable information as early and frequent as possible during patient journeys.
Scientific fields: 205-30 Radiology, Nuclear Medicine, Radiotherapy, Radiobiology; 205-12 Cardiology, Angiology; 409 Computer Science
Principal Investigator: Prof. Dr. Bernhard Kainz, Friedrich-Alexander-Universität Erlangen-Nürnberg
Target system: GPGPU cluster Alex
Project Report (05/2024)
This project explores new methods for the urgent need for computational tools based on machine intelligence in the field of medical imaging until 2028/2029. Due to the rising demand for medical imaging examinations and the subsequent strain on healthcare workers, tools that can exceed human performance and reduce unnecessary referrals could greatly improve healthcare outcomes.
Although Deep Learning (DL) and Machine Learning (ML) have made significant progress in solving challenging problems, they often perform well only for specific diseases or under specific conditions. Current ML approaches, mainly based on fully supervised learning, have limitations, such as the inability to detect diseases they haven’t been trained for, and a lack of adaptability in early diagnostic pathways.
The proposal presents a different approach, Normative Representation Learning (NRL), aiming to replicate the human ability to detect anomalies based on a sense of normality. By providing models that define what healthy anatomy and physiological dynamics should look like, the proposal envisions aiding healthcare professionals, patients, and expert clinicians with automated image interpretation capabilities.
The proposal’s main hypothesis is that confirming normality is an essential part of diagnosis and that ML methods can be developed to confirm normality and identify deviations from the norm. The PI advocates research on NRL as an unsupervised learning variant focusing on out-of-distribution detection and open-world problems, with the goal of early disease detection and second reader capabilities in diagnostic workflows.
The proposal seeks GPU compute time to develop and implement these machine learning methods, arguing that they could chanve medical imaging and healthcare more broadly.
Abstract
The establishment of suitable molecular models, based on experimental target structures, is a prerequisite for the successful design of multifunctional drugs. In all approaches of the proposed CRC (TRR 351), such models help to understand relationships of structure and function, including the interactions of the individual modules. Modeling will also guide ligand optimization. Retrospectively, molecular models can support the rationalization of the observed biological responses and integrate novel structural information obtained by biophysical methods.
Our molecular models will be important
- to understand the origins of a drug’s pharmacological effect at the atomic level. Based on existing X-ray crystallography or cryo-EM structures, our multifunctional ligands bound to their target will be investigated by long-timescale molecular dynamics (MD) simulations.
- to guide the design and development of multifunctional drugs in all project areas of the CRC. The interaction quality and stability of envisaged ligand-target complexes will be evaluated by means of docking, energy minimization and MD simulations.
- to identify new chemotypes by virtual screening. Filtering ultra-large libraries (in the order of 109 compounds) with pharmacophore models and molecular docking paves the way to novel multifunctional ligands with non-canonical receptor-ligand interactions.
- to decipher the relationships between structure and function. The elucidation of multidimensional SAR analyses allows us to predict useful modifications of lead compounds to improve affinity, selectivity and functional properties.
- to predict potential cooperativity between the modules. MD simulations of target-bound multifunctional ligands compared to their target-bound modules will guide compound development.
- to establish design principles of multifunctional biopharmaceuticals. Simulations will evaluate their stability and structure and, thus, guide their design.
Scientific field: 205-8 Pharmacy
Principal Investigator: Prof. Dr. Peter Gmeiner, Friedrich-Alexander-Universität Erlangen-Nürnberg
Target system: parallel computer Fritz
Project Report (03/2024)
β-Adrenergic receptors (βARs) induce a beneficial increase in inotropy of the heart in acute heart failure, strengthening myocardial contraction and, thus, heart function. β1AR-Gs and β2AR-Gi biased ligands with functional selectivity over β-arrestin recruitment to prevent receptor desensitization will be useful for the treatment of pathological hypertrophy and heart failure. Bitopic ligands are drugs or lead compounds comprising a pharmacophore for the orthosteric site and a second moiety specifically addressing an allosteric site. The design of our bitopic ligands will be assisted by computational methods. Whereas the choice of the orthosterically-binding modules will rely on recently described lead structures, the allosteric modules will be identified by docking studies in combination with simulation analyses. In particular, methods for the design of covalent ligands, including covalent docking, will allow us to find new chemotypes with a point of attachment that can be used to connect such fragments to orthosteric moieties via a suitable linker unit. For this purpose, both the non-covalent association complex and the covalent bond formation must be considered in the rational development and fine-tuning of covalent ligands. This strategy is being adopted for bitopic βAR ligands targeting nucleophilic residues at allosteric sites of the receptor. The particular challenge here is based on less nucleophilic and more surface-exposed residues. The warheads must be reactive enough to compensate for this problem without increasing the risk for off-target effects. This requires ligands that bind with good affinity and also enable optimal and stable orientation of the warhead. The simulations carried out so far helped to prioritize the addressable nucleophilic resdiues as well as the electrophilic warheads that can be suitably positioned for a covalent reaction. The calculations and experiments are ongoing.
5-HT1A receptor—The overuse of hydrocodone and inadequate pain treatment have contributed to a severe opioid epidemic in the United States, affecting millions of people across all socio-economic classes. The 5 HT1A receptor is a key target for pain suppression, but previous attempts faced challenges due to unwanted side effects.
In our recent study, we utilized cryo-EM to examine the structure of the 5 HT1A receptor bound to befiradol, a drug candidate with limiting side effects. We also introduced our novel ligand, ST171, which exhibited promising properties for clinical use as an analgesic. Molecular dynamics simulations revealed persistent macroscopic differences in the helical structure of the receptors, confirming that these changes were induced by the binding of different ligands.
Comparing the signaling profiles of ST171, befiradol, and the endogenous ligand serotonin yielded intriguing results. While befiradol and serotonin recruited Gi protein at low and moderate concentrations, both also recruited Gs protein at high concentrations, along with the effector protein β-arrestin. In contrast, ST171 exclusively recruited the Gi protein, even at high concentrations, suggesting a potentially superior safety profile. Molecular dynamics simulations indicated that amino acids Q972.65 and W3877.40 contributed to this difference in signaling, with stable interactions in the ST171-bound complex compared to significant fluctuations in the other complexes.
Finally, our investigation into the befiradol-bound complex revealed that the side chain of F1123.28 needed to adopt an unfavorable conformation to open a lipophilic pocket for befiradol binding. This pocket remained closed in both our ST171-bound complex and all other previously reported 5-HT1A structures. This led us to question whether befiradol binding is based on a conformational-selection or an induced-fit mechanism. Using the enhanced sampling method metadynamics we clarified that a spontaneous opening of this pocket is energetically unfavorable, suggesting that only an induced-fit mechanism is feasible.
GPR3—With the rise in life expectancy, the prevalence of Alzheimer’s disease has consistently increased in recent decades. While existing treatments slow down the disease’s progression, they fall short of providing a cure. In many Alzheimer’s patients, the GPR3 receptor is overexpressed in neurons, making it a valuable target for treatment. Furthermore, the absence of GPR3 receptors has been linked to obesity, underscoring the pharmacological importance of this receptor.
In a collaborative initiative with colleagues from the Chinese University of Hong Kong, we successfully determined the cryo-EM structure of the GPR3 receptor. However, the electron density didn’t allow for a clear identification of the bound ligand. MD simulations played a crucial role in resolving this uncertainty, demonstrating that the ligand OEA adopted a more stable binding pose compared to oleamide, the other potential candidate. This finding conclusively answers the question regarding ligand binding.
Moreover, the GPR3 receptor displays an increased basal activity. To delve into these findings, we employed our well-established metadynamics protocol to investigate the activation dynamics of the GPR3 receptor. Our simulations revealed that even in the absence of a ligand, the receptor stabilizes in a conformation that lies between a typical active and inactive state. This phenomenon elucidates the favorable basal coupling of the Gs protein.
Publications
A. Ullrich, J. Schneider, J. M. Braz, E. Neu, N. Staffen, M. Stanek, J. Bláhová, T. Hove, T. Albert, A. Allikalt, S. Löber, K. Bhardwaj, S. Rodriguez-Rosado, E. Fink, T. Rasmussen, H. Hübner, A. Inoue, B. K. Shoichet, A. J. Basbaum, B. Böttcher, D. Weikert D, and P. Gmeiner: Discovery of a functionally selective serotonin 5-HT1A receptor agonist for the treatment of pain. bioRxiv, 2023. DOI: 10.1101/2023.09.11.557127.
G. Chen, N. Staffen, Z. Wu, X. Xu, J. Pan, A. Inoue, T. Shi, P. Gmeiner, Y. Du, and J. Xu: Structural and functional characterization of the endogenous agonist for orphan receptor GPR3. Cell Research 34, 2024. DOI: 10.1038/s41422-023-00919-8.
Abstract
Medical imaging, particularly Magnetic Resonance Imaging (MRI), plays a pivotal role in contemporary healthcare, offering noninvasive diagnostic tools, guidance, and exclusive treatment monitoring and disease understanding options. The standard diagnostic medical imaging pipeline involves collecting raw data via scanner hardware, processing this data through image reconstruction algorithms, and subsequently analyzing it for pathology by radiology specialists. While this procedure has traditionally been optimized to produce visually interpretable images for human experts, it may inadvertently lead to the loss of essential patient-specific diagnostic information contained in raw sensor data.
This issue has been further compounded by recent advancements in machine learning for MRI reconstruction. Machine learning has the capacity to generate aesthetically pleasing images from minimal sensor data, which is beneficial for speedy imaging protocols that prioritize patient comfort. However, this reduction in data acquisition could cause generic image reconstruction techniques to obscure disease markers, replacing pathological features with typical healthy image features derived from the training data.
Machine learning has also shown remarkable proficiency in autonomously analyzing medical images and robustly identifying disease patterns across various modalities. However, these tools are generally detached from the image acquisition process, and are only applied to the reconstructed image data.
In this project, we aspire to amalgamate machine learning for image reconstruction and disease localization based on the image, thereby crafting an end-to-end learnable solution for image reconstruction and joint pathology detection that functions directly on raw measurement data. We predict this combination will amplify diagnostic accuracy, producing images that are ideally suited for both human experts and diagnostic machine learning models.
Our approach will lean on the capabilities of foundation models, with a particular focus on state-of-the-art diffusion models for image synthesis. This choice is motivated by their leading performance, but they require substantial GPU time for training, hence our request for more GPU compute time.
We’ve outlined three primary objectives for our project:
1. Developing a machine learning reconstruction method for accelerated MRI that withstands variations in scanners, sequences, and anatomy.
2. Designing disease detection techniques that can learn from human-generated annotations in image space while concurrently extracting information straight from accelerated raw measurement data.
3. Combining these developments to introduce end-to-end training of image reconstruction and image analysis.
We hypothesize that such an approach will prevent machine learning-based MRI interpretation from missing crucial disease indicators and offer tools to express interpretation uncertainties in image regions with limited sensor data. To this end, we will utilize our fastMRI+ dataset, which comprises fully sampled raw measurement data, images, and 20,000 specialist expert bounding box annotations for more than 50 pathology categories from over 8,000 patients.
Scientific fields: 409 Computer Science; 205-30 Radiology, Nuclear Medicine, Radiotherapy, Radiobiology; 205-12 Cardiology, Angiology
Principal Investigator: Prof. Dr. Bernhard Kainz, Friedrich-Alexander-Universität Erlangen-Nürnberg
Target system: GPGPU cluster Alex
Project Report (06/2023)
We have successful trained foundation models for ultrasound video data, anomaly detection in MRI images, and for large-scale histopathology images. We train these models in a way so that they develop a sense for “normality” and can then be refined to specific downstream tasks like MRI image reconstruction with inherent awareness of reconstruction errors.
Our team has made significant strides across six distinct sub-projects leveraging GPU resources for deep learning and imaging tasks.
Project 1 involves the use of deep adversarial super-resolution (SR) techniques to improve the quality of abdominal MRI images, particularly for patients with Inflammatory Bowel Disease (IBD). GPU resources have been used for developing and evaluating the model that corrects motion and improves image resolution.
In Project 2, we created UTAR, a framework for unsupervised test-time domain adaptation for SR in MRI. Our GPU utilization was critical in refining the pre-trained SR model and testing its efficiency in predicting high-resolution images from unseen low-resolution ones.
Project 3 aims to generate plausible video sequences from single images in echocardiograms using diffusion models. GPU resources were crucial in running experiments on the EchoNet-Dynamic dataset and developing the image-to-sequence approach, which currently shows a highly promising $R^2$ score.
Project 4 focuses on single-class modeling and out-of-distribution detection. GPU resources facilitated the development of a self-supervised learning method that makes use of multiple visually-distinct synthetic anomaly learning tasks for training and validation, offering robust results in brain MRI and chest X-rays.
In Project 5, we developed an improved self-supervised single-class training strategy for universal anomaly detection. GPU resources were instrumental in integrating our method into various out-of- distribution detection models and comparing their performance on various benchmark datasets.
Lastly, Project 6 explores a trade-off between image fidelity and interpretability in generative diffusion models. We employed GPU resources to fine-tune text-to-image models and demonstrate the interpretability of diffusion models on multi-label segmentation tasks.
Continued access to GPU resources will be instrumental in further refining these projects and their potential contributions to the field of medical imaging.
Two of this works received early accepts from the 26th International Conference on Medical Image Computing and Computer Assisted Intervention, MICCAI 2023 (acceptance rate 13%), four more are still in a rebuttal phase.
Project Report (07/2024)
Medical imaging, especially magnetic Resonance Imaging (MRI), is essential for modern healthcare, providing tools for noninvasive diagnostics, guidance, and unique monitoring options for treatment and understanding of disease.
The majority of diagnostic medical imaging pipelines follow the same principles: raw measurement data is acquired by scanner hardware, processed by image reconstruction algorithms, and then evaluated for pathology by human radiology experts.
Under this paradigm, every step has traditionally been optimized to generate images that are visually pleasing and easy to interpret for human experts. However, raw sensor information that could maximize patient-specific diagnostic information may get lost in this process.
This problem is amplified by recent developments in MRI reconstruction with machine learning (ML). ML methods allow to generate visually pleasing images from very limited sensor data. Reduced measurement data is often desired for accelerated imaging protocols, providing more patient comfort through reducing scan times from hours to minutes. Though, the less data is acquired the more generic image reconstruction methods will hide signs of disease, replacing pathology-defining features with more likely healthy image features from the training data.
ML has also been tremendously successful for analyzing medical images automatically and to detect patterns of disease robustly across modalities. So far, these tools have been disjointed from the image acquisition process and are usually only applied to reconstructed image data. In this project we would like to seize the opportunity to fuse ML for image reconstruction and ML for image-based disease localization, thus providing an end-to-end learnable image reconstruction and joint pathology detection approach that operates directly on raw measurement data. We expect that this combination can maximize diagnostic accuracy while providing optimal images for both human experts and diagnostic ML models.
To achieve this, our project includes three goals and three corresponding work packages.
(1) we will develop a ML reconstruction approach for accelerated MRI that is robust towards different scanners, sequences, and anatomy.
(2) we will develop disease detection methods that can learn from human-generated annotations in image space and at the same time extract information directly from accelerated raw measurement data.
(3) we will bring these developments together and establish end-to-end training of image reconstruction and image analysis. Our hypothesis is that such an approach will prevent ML-based MRI interpretation from missing important signs of disease and provide tools to communicate interpretation uncertainties in image regions with limited sensor data. We will use our fastMRI+ dataset that contains fully sampled raw measurement data, images and 20.000 specialist expert bounding box annotations for more than 50 pathology categories from more than 8.000 patients.
Publications
M. Baugh, J. Tan, J. Müller, M. Dombrowski, J. Batten, and B. Kainz: Many tasks make light work: Learning to localise medical anomalies from multiple synthetic tasks. Medical Image Computing and Computer Assisted Intervention–MICCAI 2023: 26th International Conference, Vancouver, Canada, 2023. DOI: 10.1007/978-3-031-43907-0_16.
H. Reynaud, M. Qiao, M. Dombrowski, T. Day, R. Razavi, A. Gomez, P. Leeson, and B. Kainz: Feature-conditioned cascaded video diffusion models for precise echocardiogram synthesis. Medical Image Computing and Computer Assisted Intervention–MICCAI 2023: 26th International Conference, Vancouver, Canada, 2023. DOI: 10.1007/978-3-031-43999-5_14.
W. Zhang, B. Basaran, Q. Meng, M. Baugh, J. Stelter, P. Lung, U. Patel, W. Bai, D. Karampinos, and B. Kainz: MoCoSR: Respiratory Motion Correction and Super-Resolution for 3D Abdominal MRI. Medical Image Computing and Computer Assisted Intervention–MICCAI 2023: 26th International Conference, Vancouver, Canada, 2023. DOI: 10.1007/978-3-031-43999-5_12.
M. Dombrowski and B. Kainz: Quantifying Sample Anonymity in Score-Based Generative Models with Adversarial Fingerprinting. arXiv, 2023. DOI: 10.48550/arXiv.2306.01363.
J. P. Müller, M. Baugh, J. Tan, M. Dombrowski, and B. Kainz: Confidence-Aware and Self-Supervised Image Anomaly Localisation. arXiv, 2023. DOI: 10.48550/arXiv.2303.13227.
M. Dombrowski, H. Reynaud, J. P. Müller, M. Baugh, and B. Kainz: Pay Attention: Accuracy Versus Interpretability Trade-off in Fine-tuned Diffusion Models. arXiv, 2023. DOI: 10.48550/arXiv.2303.17908.
S. Cechnicka, J. Ball, H. Reynaud, C. Arthurs, C. Roufosse, and B. Kainz: Realistic Data Enrichment for Robust Image Segmentation in Kidney Transplant Pathology. arXiv, 2023. DOI: 10.48550/arXiv.2304.09534.
G. Shkëmbi, J. P. Müller, Z. Li, K. Breininger, P. Schüffler, and B. Kainz: Whole Slide Multiple Instance Learning for Predicting Axillary Lymph Node Metastasis. Data Engineering in Medical Imaging–First MICCAI Workshop, DEMI 2023, Held in Conjunction with MICCAI 2023: 26th International Conference, Vancouver, Canada, 2023. DOI: 10.1007/978-3-031-44992-5_2.
Abstract
Our project includes unbiased and biased molecular dynamics simulations of the FPR receptor class and its vast array of ligands that include modified peptides as well as non-modified peptides and small molecules like Lipoxin A4 or the circular peptide Ciclosporin A. A standardized metadynamics protocol is applied to study the binding/unbinding events of the ligands to these receptors and enhance sampling. High parallel performance is achieved using multiple-walker simulations. The ligands will include agonists, partial agonist and antagonist to cover as many different binding routes as possible.
Scientific field: 205-08 Pharmacy
Principal Investigator: Prof. Dr. Oliver Koch, Universität Münster
Target system: parallel computer Fritz & GPGPU cluster Alex
Project Report (10/2023)
The Formyl Peptide Receptors (FPRs) belong to class A of G-protein coupled receptors (GPCRs); the family has three members: FPR1, FPR2 and FPR3. FPRs play a key role in the host defense against microbes because they are located on immune cells like phagocytes that belong to the innate immune system. Thus, they are also involved in inflammatory diseases like Alzheimer’s disease or cancer and are novel targets for the treatment of those diseases. Several small molecules and peptides are known which either act as agonists, partial agonists or antagonists. We selected some of the small molecules that represent these classes for further computational analyses including binding-mode identification, binding-pathway analysis and free energy of binding calculations using metadynamics simulations. First, the molecules were docked into the orthosteric binding site of FPR2 of a cryo-EM structure in complex with a known peptide agonist (PDB 7WVW). The resulting FPR2 complex structures were used for μs-time scale metadynamics simulations conducted mainly on the Alex cluster of NHR@FAU in Erlangen using Gromacs and Plumed. The simulations were run following a protocol that was already described earlier by Saleh et al.. With this approach, it was possible to simulate the binding/unbinding of the ligands and to determine the free energies of binding with sufficient accuracy. The global free energy minimum obtained corresponded well to the known binding poses and gave new insight into the binding mode of small-molecule ligands. This knowledge will be used to design novel ligands for FPR2. The results highlight the importance of a deep, hydrophobic pocket at the bottom of the orthosteric site and of three polar residues right above it. Furthermore, these data suggest comparable binding modes of small molecules and peptides, contrary to earlier results.
Abstract
We aim to provide high-quality body models given medical imaging data by the segmentation of relevant structures. Fast 3D modelling is relevant in clinical-radiological everyday routine. In the automatic modeling part of a DFG project, current machine learning methods and atlas-based methods are to be compared for their segmentation proposals and combined to their strengths. A major goal of this project is the development of novel, fully automatic, group-oriented deep-learning and multi-atlas segmentation estimators for the highly efficient multi-organ and simultaneous tumor segmentation in medical 3D-CT image data sets.
Scientific fields: 205-01 Epidemiology, Medical Biometry, Medical Informatics; 409-05 Interactive and Intelligent Systems, Image and Language Processing, Computer Graphics and Visualisation
Principal Investigator: Prof. Dr. Andre Mastmeyer, Aalen University
Target systems: parallel computer Fritz & GPGPU cluster Alex
Neurosciences
Abstract
This project aims to align the preprocessing of two large MRI datasets from the UK Biobank and NAKO Gesundheitsstudie, predominantly comprising healthy individuals. Subsequently, the preprocessed data will be utilized for predicting various phenotypes from neuroimaging data modalities and a comparative analysis of the results between the two datasets will be conducted based on robustness and reproducibility criteria.
Scientific field: 206-04 Systemic Neuroscience, Computational Neuroscience, Behavior
Principal Investigator: Dr. Marc-André Schulz, Charité, Universitätsmedizin Berlin
Target systems: parallel computer Fritz
Abstract
The CRC1540 EBM project explores mechanical influences on the central nervous system (CNS), of which many processes are not yet fully understood. Mechanical signals have shown to impact CNS cell function, emphasizing mechanics’ crucial role. EBM aims to enhance CNS understanding for improved neurological disorder diagnosis and treatment. Subproject X02 focuses on leveraging the diverse data collected within EBM and employs advanced machine learning for domain adaptation and generalization.
Scientific fields: 206-04 Systemic Neuroscience, Computational Neuroscience, Behavior; 409 Computer Science
Principal Investigator: Prof. Dr.-Ing. Katharina Breininger, Julius-Maximilians-Universität Würzburg
Target systems: GPGPU cluster Alex
Abstract
In this project, we want to explore the computational power of HPC-Cluster for modelling single-channel patch-clamp data with Markov models. The 2D-Dwell-Time fit with simulations of time series captures gating kinetics with a high background of noise and can extract rate constants beyond the recording bandwidth. That makes the 2D-Fit exceptionally valuable for relating ion-channel kinetics with data from simulations of single molecules. In addition, 2D-distributions preserve the coherency of connected states. Thereby the algorithm can extract the full complexity of underlying models and distinguish different models.
Scientific field: 206-04 Systemic Neuroscience, Computational Neuroscience, Behavior
Principal Investigator: PD Dr. Dr. Tobias Huth, Friedrich-Alexander-Universität Erlangen-Nürnberg
Target systems: parallel computer Fritz & GPGPU cluster Alex
Project Report (02/2024)
Ion channel proteins utilize the electrochemical gradients across cell membranes to govern important func- tions of life such as the transport of molecules across membranes or the processing and flow of information in organisms. The patch-clamp method allows investigating single ion-channels during their work in real time with high temporal resolution. Since the invention of this technique by the German Nobel laureates, Erwin Neher and Bert Sakmann, scientist strive to extract the underlying kinetics of ion channels in health and disease in the form of Markov models. The extraction of such models from time series proved to be notoriously difficult because of the tiny currents in the range of pA to fA, the huge noise amplitude and the related limited temporal resolution.
In this project, we made a big step forward by implementing the 2D-Fit algorithm on HPC-cluster. The program simulates time series with Markov models and adjusts the model parameters until matching experimental time series. The cluster enables us to obtain results within a reasonable time frame and provided the opportunity to evaluate the results by statistical means. Currently we are in the process of shifting the analysis towards Deep Learning. The simulation of synthetic time series serves as training data. If properly implemented, this approach should enable us to conduct kinetic model analysis online during a patch-clamp experiment.
Publications
E. Oikonomou, T. Gruber, A. R. Chandra, S. Höller, C. Alzheimer, G. Wellein, und T. Huth: 2D-dwell- time analysis with simulations of ion-channel gating using high-performance computing. Biophysical Journal 122(7), 2023. DOI: 10.1016/j.bpj.2023.02.023.
Natural Sciences
Molecular Chemistry
Abstract
The isolation of multiple bonded late transition metal complexes is a challenging task. Nevertheless, they are alleged key intermediates for important “every day” catalysis processes such as the oxidation of ammonia to nitric acid by O2 (Ostwald process) or the catalytic depletion of toxic exhaust gases. Our calculations predict how to tame these elusive molecules to study them in the laboratory. Our work focused so far on terminal imido complexes of the late transition metals (Mn, Fe, Co, Ni, Ir, Pd, Pt) and has not only allowed to understand their intriguing electronic structure, but furthermore to demonstrate first applications in small molecular activation, energy conversion, catalysis, and photochemistry.
Similarly, we use computational methods to study organic redox systems and diradicals. We have predicted how to harness the peculiar properties of carbene decorated diradicals in solar cells and demonstrated their use as singlet fission molecules. Thus, our calculations helped to discover a new class of molecules of use for solar energy conversion, quantum computing, or organic light emitting diodes (OLEDs).
Scientific fields: 321-01 Inorganic Molecular Chemistry; 321-02 Organic Molecular Chemistry; 323-01 Physical Chemistry of Molecules, Liquids and Interphases, Biophysical Chemistry
Principal Investigator: Prof. Dr. Dominik Munz, Saarland University
Target system: parallel computer Fritz
Project Report (12/2022)
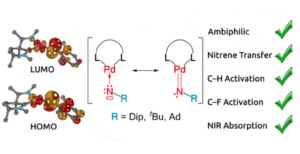
Our project deals with molecules with unusual electronic structures. We aim to harness these electronic structures in green catalysis as well as for material design in organic electronics such as photovoltaics. For bringing these molecules to application, a profound understanding of their complicated electronic structures is required.
As such, we perform synthetic, computational- and spectroscopic work hand-in-hand for optimal synergy. So far, we have focused on late transition metal complexes for catalysis and carbene-derived organic materials. A recent breakthrough was the isolation of the first example of a terminal nitrene of palladium.
Another landmark finding was, how carbenes stabilize radicals for organic electronics. In subsequent steps, we wish to extend our studies to other earth-abundant elements for enhanced sustainability.
Abstract
Nanoporous materials are of interest in many applications due to their high surface area and physicochemical properties. The interconnected channels can be used for “flow-through” applications such as purification of drinking water or nanoseparation of proteins or organic solvents. Here, we will evaluate the impact of (i) nanomaterial kind, (ii) pore size, (iii) pore shape, and (iv) solvent polarity on the material’s permeability using sequential multiscaling molecular dynamics (MD). We will establish molecular models of 3D structures of amorph carbonaceous materials and of diverse metal-organic frameworks. Next, variation of the pore diameter, surface functionalization and solvent polarity will enable us to systematically evaluate the impact of the individual material properties on e.g. molecular transport, solvent competition, and nanoseparation.
Scientific fields: 301 Molecular Chemistry; 302-03 Theory and Modelling
Principal Investigator: Dr. Kristyna Pluhackova, University of Stuttgart
Target system: parallel computer Fritz & GPGPU cluster Alex
Publications
J. Wachlmayr, G. Fläschner, K. Pluhackova, W. Sandtner, C. Siligan, and A. Horner: Entropic barrier of water permeation through single-file channels. Communications Chemistry 6(135), 2023. DOI: 10.1038/s42004-023-00919-0.
Chemical Solid State and Surface Research
Abstract
This project seeks computational support to investigate the potential of NaxV2(PO4)2F3, a polyanionic compound, as a promising material for sodium-ion batteries. With geopolitical challenges surrounding lithium-ion batteries, there is a growing interest in alternative, cost-effective systems. Na3V2(PO4)2F3 exhibits stable capacity and commendable rate capability over 4000 cycles, making it a compelling candidate.
The primary research objective is to understand the mechanisms limiting sodium extraction in fluorophosphate cathodes, with a focus on expanding the compositional range and introducing transition metals for enhanced performance. Utilizing Density Functional Theory (DFT) using the Vienna Ab initio Software Package and in combination with nudged elastic band (NEB) method, we aim to explore the stability and electrochemical proprieties of these materials.
Scientific field: 302 Chemical Solid State and Surface Research
Principal Investigator: Prof. Dr. Matteo Bianchini, Universität Bayreuth
Target system: parallel computer Fritz
Abstract
Ferroelectrics are insulators with a spontaneous electrical polarization that can be reversed by an external electric field. Their properties make them sought-after functional materials with a wide range of potential applications. A basic requirement for the occurrence of ferroelectricity is that the material crystallizes in a polar space group.
In this work we want to predict new ferroelectric metal fluorides. To this end, we will compile an overview of polar metal fluorides, which we will investigate using crystallographic and quantum chemical methods. The starting point is a database screening that yields fluorides in 105 structure types that potentially crystallize in polar space groups. We will perform DFT calculations on individual representatives of the structure types to confirm their polar structure.
We will perform structural optimizations and calculate vibrational frequencies at the Γ-point in the harmonic approximation to verify that our candidates are dynamically stable at 0 K. If the DFT structural optimization converges to a nonpolar structure, we will classify the structural model of this compound as potentially erroneous and suggest a redetermination of the crystal structure. We will perform the DFT calculations with the program CRYSTAL23 using the hybrid functional PBE0 and atom-centered basis functions. We will adopt technical parameters and convergence criteria from our previous theoretical studies on fluorides. All structure types of our screening have less than 200 atoms per unit cell. From our preliminary investigations and the system sizes, we extrapolate the required computing time to about 500,000 CPU hours. For all structural optimizations, we estimate the requirement at 100,000 CPU hours, for the frequency calculations at 400,000 CPU hours. Crystal23 is effectively parallelized via OpenMP and MPI. For our system sizes, we use 16 to 64 cores and 4 GB to 32 GB RAM as standard.
Scientific field: 302 Chemical Solid State and Surface Research
Principal Investigator: Prof. Dr. Florian Kraus, Philipps-Universität Marburg
Target system: parallel computer Fritz
Abstract
Catalysis at liquid interfaces (CLINT) provides a fascinating new research area with great potential to explore more efficient and sustainable catalytic processes. Since such kind of catalysis, especially those on supported catalytically active liquid metal solutions (SCALMS) and surface catalysis with ionic liquid layers (SCILL), is still quite new, much more understanding need to be gained on how exactly the mechanistic processes are taking place, leading to know-how accelerating the development of targeted systems for several reactions. Periodic DFT simulations are able to shed a light on the exact processes taking place at the catalyst. Recently, a new machine-learning force-field (ML-FF) was developed which is able to efficiently learn on the fly from DFT data, leading to a high-level FF for metal surfaces in contact with other phases, which are very complicated to describe with FFs so far. By parametrizing these ML-FFs for SCALMS and SCILL systems, we are able to explore new time- and length scales.
Scientific field: 302-03 Theory and Modeling
Principal Investigator: Prof. Dr. Andreas Görling, Friedrich-Alexander-Universität Erlangen-Nürnberg
Target system: parallel computer Fritz
Project Report (10/2023)
Our calculations focus on the atom-resolved understanding of novel catalyst systems, namely catalysis on liquid interfaces (CLINT). In contrast to usual heterogeneous catalysis taking place on metal surfaces, the novel systems have the advantage of better control of the catalytic processes and being less prone to unwanted side-effects like poisoning and coking. Two different catalytic concepts are mainly studied: Supported Catalytically Active Liquid Metal Solutions (SCALMS) and Surface Catalysis with Ionic Liquid Layers (SCILL). Within SCALMS, active atoms like Pt are distributed into a liquid solvent metal like Ga. The Pt atoms move around in the liquid and occasionally reach the surface, where they are available to the catalysis. Our simulations focus on how this motion actually takes place on an atomistic level. Since liquid metals are studied rarely by theory on larger scales, we set up novel machine learning force fields (ML-FF) for a cheap but nevertheless accurate simulation of them, enabling averaging of the surface properties. We further consider in our ML-FFs the interaction of the metal solvents with the support material, on which droplets of them are placed in reality and the formation of oxide layers on the surface and intermetallic phases in the liquid, thus being able to study a large part of the real-world complexity of SCALMS. Electronic properties of the systems can be calculated by periodic DFT, using snapshots of the ML-FF simulations. Within SCILL, the catalytically active metal surface is coated with a thin ionic liquid (IL) film. Depending on the IL species present, the interactions with the reactive species can be fine-tuned, further, unwanted side-reactions are suppressed by the coating. Our simulations aim at a detailed description of the interactions of IL molecules with the metal surface. We optimize IL adsorption patterns by DFT and simulate scanning tunneling microscope (STM) images of them, we further study the electronic properties of the IL/metal interface by applying electric fields to model systems with CO molecules adsorbed on the surface, acting as probing species. In close collaboration with the Zahn group, we are able to link the Coulomb interactions of explicit IL molecules obtained from traditional force field simulations to effective applied electric fields in model DFT surfaces. Besides these two main branches, we use our calculation time for several smaller projects, all covering novel surface systems, like the interaction of hexagonal bornitride (h-BN) sheats on a Rh surface with Br molecules. Due to fascinating nanomesh (moire) patterns arising from a unit cell mismatch of the Rh surface and the h-BN sheet, the interaction with gaseous Br is versatile and complicated. Besides thorough DFT calculations, we are able to simulate the real-time dynamics of such a system using a ML-FF trained for that purpose.
Physical and Theoretical Chemistry
Abstract
This project aims at designing tailor-made functionalization of magnetite nanoparticles to bind heavy metal ions and related organometallic compounds by means of molecular modelling and simulation. While at this stage reflecting a pure theory project, we aim at developing a model-based search strategy for identifying suitable constituents and structures as guides to syntheses. To achieve this, we build on a recently established concept for the removal of oil pollution from water that takes use of super-paramagnetic nanoparticles functionalized by self-assembled monolayers (SAMs) of n-alkyl phosphonic acids. While the association of hydrocarbons to the alkyl chains of such SAMs is driven by a simple hydrophobic segregation mechanism, well-chosen SAMs of tailor-made chelating residues are needed for efficiently binding and removing heavy metal pollutants from water. This calls for in-depth understanding of molecular recognition and the tuning of structural motifs – which shall both be achieved from molecular simulations.
Scientific fields: 303-02 Theoretical Chemistry; 406 Materials Science
Principal Investigator: Prof. Dr. Dirk Zahn, Friedrich-Alexander-Universität Erlangen-Nürnberg
Target systems: parallel computer Fritz & GPGPU cluster Alex
Condensed Matter Physics
Abstract
The quantum world fundamentally differs from the classical world, that we intuitively understand through our daily experience. The mathematical description of quantum systems with many degrees of freedom suffers from a curse of dimensionality and predictions of physical theories are fundamentally restricted to be probabilistic. While these properties form the basis for the potential utility of quantum computers, they are also the cause of the greatest obstacles when creating such devices. In our Helmholtz Young Investigator Group, we aim to exploit the known strengths of machine learning approaches in dimensional reduction and pattern recognition for the advancement of near-term quantum technology. In particular, we will push the boundaries of classical simulations for the certification of quantum simulators, which will become a valuable extension of our scientific toolbox to study complex quantum systems. The basis of this research is a representation of many-body quantum states as artificial neural networks. The goal of this project is on the one hand to improve our understanding of this novel approach, and, on the other hand, to exploit the new capabilities in order to investigate otherwise inaccessible terrain in the realm of correlated matter.
Scientific field: 307-02 Theoretical Condensed Matter Physics
Principal Investigator: Dr. Markus Schmitt, University of Regensburg
Target system: GPGPU cluster Alex
Abstract
The possibility to precisely manipulate and control artificial quantum systems constitutes the foundation of emerging quantum technologies. Of particular interest for the field of correlated quantum matter is the possibility to emulate theoretical models using quantum mechanical degrees of freedom. However, our understanding of how to manipulate such systems using external drives is in its infancy, especially in the presence of strong interactions. We propose to overcome some of the current limitations by combining techniques from machine learning with ideas from quantum many-body physics. Within this project, we will develop simulation methods for periodically driven systems based on neural quantum states (NQS), and a new interpretable framework for tensor-networks- based reinforcement learning (RL) to optimize control of quantum matter.
Scientific field: 307-02 Theoretical Condensed Matter Physics
Principal Investigator: Dr. Markus Schmitt, University of Regensburg
Target system: GPGPU cluster Alex
Abstract
The urgent need to decarbonise our society leads to major materials-science challenges in the transition from fossil to hydrogen-based fuels. It is becoming increasingly critical that H is integrated into materials design principles and in lifetime predictions of engineering parts. This calls for a thorough understanding of the interplay between H atoms and the local geometric and chemical details of the microstructure. This project addresses the impact of H on single-crystal Ni-based superalloys and on grain boundaries in ferritic alloys. The atomistic simulations involve high-throughput ab-initio calculations and the development of machine-learning potentials based on the atomic cluster expansion for large-scale atomistic simulations.
Scientific fields: 307-02 Theoretical Condensed Matter Physics; 406-03 Microstructural Mechanical Properties of Materials
Principal Investigator: PD Dr. habil. Thomas Hammerschmidt, Ruhr-Universität Bochum
Target system: parallel computer Fritz
Abstract
Molten salts electrolysis is a well-known technique to extract metals from ores. Nevertheless, the optimization of cell design and associated processes are limited by incomplete thermodynamic databases. When available, the data can be employed to devise corrosion phase diagrams that are crucial to draw next generation electrochemical reactors. The key quantity in such diagrams is the formation energy of the metal ions to extract and that would dissolve in the electrolyte, but it’s evaluation from experiments is challenging and expensive. An alternative solution is to calculate such quantities by first-principles simulations. While the latter are still challenging, the computational effort is eased by leveraging advanced computational techniques such as active learning density-functional theory molecular dynamics, machine learning neural networks, and reproducible high-throughput calculations. In particular, we focus on the computation and prediction of ion formation energies in molten chloride electrolytes, such as LiCl, KCl, NaCl, CaCl2, and MgCl2. This work founds the base to enable selective metal extraction by providing theoretical guidance that complements experimental efforts.
Scientific fields: 307 Condensed Matter Physics; 406-01 Thermodynamics and Kinetics of Materials
Principal Investigator: Prof. Dr.-Ing. Lucio Colombi Ciacchi, Universität Bremen
Target system: GPGPU cluster Alex
Abstract
We will explore in a spatially and time-resolved manner charge carrier dynamics on three-dimensional TI surfaces subject to time-dependent laser pulses or radiative driving. We will in particular explore the high-harmonic generation for pulse-type of applied external fields, a timely subject of much conceptual, experimental and technological interest. To this end we will employ complementary quantum mechanical wave packet-based techniques and semiclassical path integral methods, with emphasis on reaching the regimes of parameters where the well-established so-called three-step-method may break down and non-classical mechanisms are responsible for the emission process.
Scientific field: 307-02 Theoretical Condensed Matter Physics
Principal Investigator: apl. Prof. Dr. Juan Diego Urbina, University of Regensburg
Target system: GPGPU cluster Alex
Project Report (07/2024)
This test/porting project was used to determine the viability of parameter exploration on GPUs using in-house software on the NHR GPU cluster Alex. We were able to show a perfectly linear scaling of runtime with problem size and establish that the runtime is roughly an order of magnitude faster on an A100 than on a typical 64-core CPU node.
Furthermore, we began a two-dimensional parameter space exploration of a massive Dirac model driven by a linearly polarized laser pulse. These time-resolved simulations can be used e.g. to calculate high-harmonic spectra. It was not possible to obtain converged results within the computation time of the test/porting project, but it allowed an estimation of the runtime required for the follow-up project.
Abstract
Long-range interactions in unconventional superconductors are of high importance as they can give rise to topologically nontrivial phases of matter within the mean-field approximation. In this work, we use the T-matrix approach to derive a generalized gap equation that goes beyond the mean-field approximation. We subsequently investigate how the phase diagram of a 2D superconductor changes as higher-order quantum corrections are included. Going further, we explore whether the Higgs mode, which can be stabilized due to long-range interactions in the mean field limit, remains stable in the T-matrix approach.
Scientific field: 307-02 Theoretical Condensed Matter Physics
Principal Investigator: Dr. Andreas Buchheit, Saarland University
Target system: parallel computer Fritz
Publications
A. A. Buchheit, T. Keßler, P. K. Schuhmacher, and B. Fauseweh: Exact continuum representation of long-range interacting systems and emerging exotic phases in unconventional superconductors. Physical Review Research 5, 2023. DOI: 10.1103/PhysRevResearch.5.043065
Abstract
Planar carbon lattices (PCLs), such as graphene nanoribbons, patterned graphene, 2D metal- organic/covalent-organic frameworks (MOFs/COFs), 2D polymers and their nanoribbons, combine the versatility of carbon-based molecular building blocks with the long-range order and symmetry-imposed properties of a 2D lattice. In recent years, precision synthesis methods have made tremendous progress in creating predefined PCLs on surfaces or at liquid interfaces. In this project, we will investigate the physical properties of such PCLs by numerical methods and predict new PCL structures with intriguing topological and correlated electronic properties.
Scientific field: 307-02 Theoretical Condensed Matter Physics
Principal Investigator: Prof. Dr. Janina Maultzsch, Friedrich-Alexander-Universität Erlangen-Nürnberg
Target system: parallel computer Fritz
Publications
S. Wolff, R. Gillen, and J. Maultzsch: Electronic Properties and Interlayer Interactions in Antimony Oxide Homo- and Heterobilayers. Phys. Status Solidi B 260, 2023. DOI: 10.1002/pssb.20
Abstract
FRASCAL improves understanding of fracture in brittle heterogeneous materials by developing simulation methods able to capture the multiscale nature of failure. With i) its rooting in different scientific disciplines, ii) its focus on the influence of heterogeneities on fracture at different length and time scales as well as iii) its integration of highly specialized approaches into a “holistic” concept, FRASCAL addresses a truly challenging cross-sectional topic in mechanics of materials.
In particular, sub-project P12 (Quantum to Continuum Model of Thermoset Fracture), will develop a concurrent multiscale modelling approach to study the interplay and coupling of process on different length scales (e.g. breaking of covalent bonds, chain relaxation processes, fibril formation and crazing at heterogeneities,…) during the fracture of an exemplary thermoset and its dependence on the (local) degree of cross-linking.
Scientific field: 307-02 Theoretical Condensed Matter Physics
Principal Investigator: Prof. Dr. Ana-Suncana Smith, Friedrich-Alexander-Universität Erlangen-Nürnberg
Target system: parallel computer Fritz & GPGPU cluster Alex
Abstract
We investigate the quantum cooperativity of strongly correlated light-matter systems in the presence of spatially competing matter-matter interactions. We aim at a deeper understanding of quantum phases and quantum phase transitions, where competing long-range interactions are expected to result in unconventional correlations and interesting entanglement properties. To this end we employ linked- cluster expansions on white graphs in combination with classical Monte Carlo integration to determine relevant physical quantities in the thermodynamic limit. This allows us to determine the notoriously complicated quantum-critical properties in quantum many-body systems with long-range interactions.
Scientific field: 307-02 Theoretical Condensed Matter Physics
Principal Investigator: Prof. Dr. Kai Phillip Schmidt, Friedrich-Alexander-Universität Erlangen-Nürnberg
Target system: parallel computer Fritz
Abstract
This project focuses on simulating and understanding topological materials and their excitations under ultrafast laser driving; it is motivated by experimental activities towards “lightwave spintronics” in Dirac systems. The main diagnostic observable will be the optical and magnetic responses, in particular the high harmonic regime and Kerr rotations.
Scientific field: 307-02 Theoretical Condensed Matter Physics
Principal Investigator: Dr. Jan Wilhelm, University of Regensburg
Target system: parallel computer Fritz
Abstract
Twisted bilayer graphene (TBLG) has recently captivated the interest of the condensed matter community, for its capability of hosting a wide variety of peculiar phenomena such as superconductive and correlated insulating phases, as well as topological features. The aim of this project is to apply Dynamical Mean-Field Theory (DMFT) to a recently developed heavy-fermion model for TBLG, investigating the onset of correlated insulating phases for different occupations and temperatures, as well as dynamical screening effects.
Scientific field: 307 Condensed Matter Physics
Principal Investigator: Dr. Lorenzo Crippa, Julius-Maximilians-Universität Würzburg
Target system: parallel computer Fritz
Project Report (03/2024)
The first stage of the DMFT2TBLG project has focused both on Twisted Bilayer Graphene proper and on an equivalently interesting bilayer setup, the 1T-1H TaS2 heterostructure.
Regarding bilayer graphene, we have elucidated the behavior of the local moment fluctuations across a wide range of temperatures and fillings, determining how the heavy electrons in the flat bands are not efficiently screened up to very low values of temperature. We have further analyzed the charge compressibility curve of the Song-Bernevig model for MATBLG, highlighting a number of negative compressibility branches in accordance with recent experiments.
On the topic of TaS2, we have described the physics governing its peculiar spectral properties, showing a narrow peak near zero bias in various experimental realizations: we have determined it to be due not to heavy-fermion physics, but to a doped Mott scenario, by which the Mott insulating 1T layer is depleted by a work function gradient with respect to the metallic 1T layer, acting as an electron reservoir. We have supported our claim with a DFT+DMFT study, analyzing the properties of the Fermi surface and the local spin susceptibility.
Publications
L. Crippa, H. Bae, P. Wunderlich, I. I. Mazin, B. Yan, G. Sangiovanni, T. Wehling, and R. Valentí: Heavy fermions vs doped Mott physics in heterogeneous Ta-dichalcogenide bilayers. Nature Communications 15, 2024. DOI: 10.1038/s41467-024-45392-y.
Abstract
The efficient numerical simulation of quantum systems constitutes a key challenge for current computational methods. In this project, we employ a modern method named neural quantum states which has shown great potential in studying various quantum problems. We plan to target both ground states as well as the time evolution of cutting-edge quantum models to gain new insights on novel quantum phenomena.
Scientific field: 307-02 Theoretical Condensed Matter Physics
Principal Investigator: Prof. Dr. Markus Heyl, Augsburg University
Target system: GPGPU cluster Alex
Project Report (09/2024)
In this project, we focus on the neural quantum state method which is a compressed representation of quantum many-body systems. Previously, the neural networks used in this method are usually shallow with only around 10 layers and thounsands of parameters due to the difficulty of optimization. We develop a new optimization method named MinSR that allows us to implement deep neural quantum states with up to 64 layers and 1 million parameters, which are significantly larger than previous neural quantum state practices. This allows us to obtain higher variational accuracy in several quantum systems and more reliable properties of the underlying quantum spin liquids. The deep neural quantum state with MinSR will open a new route for the numerical studies of strongly correlated many-body quantum systems. Apart from the current applications on quantum spin liquids, it can also be applied to fermionic systems or quantum dynamics for a better understanding on these systems.
Project Report (07/2023)
In this project, we utilize so-called neural quantum state method, which provide a compressed representation of the quantum many-body state into artificial neural networks. Up to now this method has been limited shallow networks with only around thousands of parameters, which has been caused by the computational complexity of optimization.
We have developed a new optimization method named MinSR that allows us to train deep neural quantum states of unprecedented size with up to 64 layers and 1 million parameters. This has allowed us to significantly improve the variational accuracy of the ground state for several paradigmatic quantum systems.
We expect that the deep neural quantum state trainable with MinSR will open up a new route for the numerical studies of strongly correlated many-body quantum systems. Apart from the current applications on quantum spin liquids, it can also be applied to fermionic systems or quantum dynamics in the future.
Abstract
Current ab-initio theory for solid state materials excels in the prediction of electronic band structures. The secondary part of any full description – the interaction between electrons – is beyond the scope of those methods. To remedy this omission the functional renormalization group (FRG) has the expressed goal of deriving effective low-energy interaction models. This method has successfully been used to calculate some simple systems, but the goal of this project is to widen the scope of possible applications and include ever expanding classes of materials. We hope to uncover new effects such as pair-density waves, nematic instabilities and thus extend the predictive power of FRG.
Scientific field: 307-2 Theoretical Condensed Matter Physics
Principal Investigator: Prof. Dr. Carsten Honerkamp, RWTH Aachen University
Target system: parallel computer Fritz
Project Report (01/2024)
Within this project, we have investigated models for common material classes such as square, triangular, and hexagonal lattice structures. Already for this seemingly simple model systems, the effective low-energy theory revealed a broad range of highly unconventional phases in certain parameter regimes. The close competition and cooperation between different symmetry breaking propensities often omits a direct intuitive understanding of the phase diagram.
Our calculations utilized the functional renormalization group, an iterative approach to the prediction of quantum mechanical effects in many-electron systems. With this numerical method, it is possible to successively incorporate higher order screening processes for the electron-electron repulsion provided by the crystallographic environment in a controlled way and monitor the emergence of different ordering tendencies when approaching lower energy scales. Within each step of the iterative procedure, electrons of a distinct energy scale are included resulting in feasible calculation times.
This profile promotes the functional renormalization group as a ideal tool to provide accurate numerical estimates for the parameter dependence of the emergent low temperature phases and simultaneously a detailed understanding of the physical origin governing the encountered phase transitions. This allowed us to uncover guiding principles for the emergence of long sought after pair density waves in the Kagome materials, a new route toward correlated topological physics in graphene and establish a direct connection between spin-orbit coupling and geometrical frustration governing the phase diagram of the triangular lattice Hubbard model.
Abstract
Correlation effects in the quantum mechanical many body problem are fascinating since there seems to be an unlimited richness in emergent phenomena. The common feature of all the subjects presented in this grant proposal is a model Hamiltonian that describes the physics at high energy. The aim is to understand the emergent low-lying and critical phenomena. Generically since the many body quantum mechanical problem does not allow for an exact analytical solution, there is no way to unambiguously elucidate the nature of the low lying excitations. Hence numerical simulations. The numerical simulations that we will carry out here are exact: for a given dimension of the Hilbert space (i.e. volume V ) and inverse temperature, β, we can carry out an unbiased calculation. However, since critical and emergent phenomena is very often a property of the thermodynamic limit, the bigger the system size the more conclusive and impactful our results. This essentially explains why we are dependent on supercomputing resources. In fact without access to supercomputing resources, we would not be able to address the questions posed in this proposal.
Our tool is a general implementation of the so called auxiliary field quantum Monte Carlo algorithm. This approach is triggered at solving systems of correlated electrons that couple to bosonic modes such as lattice vibrations. For a class of models that do not suffer from the so called negative sign problem, this approach allows us to compute properties of systems in thermodynamic equilibrium at polynomial cost. Generically, for short ranged interactions, the computation time scales as βV3.
Scientific field: 307-02 Theoretical Condensed Matter Physics
Principal Investigator: Prof. Dr. Fakher Assaad, Julius-Maximilians-Universität Würzburg
Target system: parallel computer Fritz
Project Report (10/2023)
The ALF library implementation of the auxiliary field quantum Monte Carlo method allows for simulations of lattice fermions. Using this approach we have investigated emergent and topological phenomena in quantum matter. Highlights include a novel formulation for the simulation of the canonical Su-Schrieffer-Heeger model of lattice vibrations coupled to electrons on a square lattice. This model shows emergent Dirac fermions as well as Landau forbidden continuous transitions between different broken symmetry states. We have equally pursued our work in the domain of magnetic impurities in metals. By adopting a setup where there is a dimensional mismatch between the magnetic impurities and conduction electrons we can realize metallic magnetic phase transitions and analyze the critical behavior.
Publications
B. Frank, Z. H. Liu, F. F. Assaad, M. Vojta, and L. Janssen: Marginal Fermi liquid at magnetic quantum criticality from dimensional confinement. Phys. Rev. B 108, 2023. DOI: 10.1103/PhysRevB.108.L100405.
J. Schwab, F. P. Toldin, and F. F. Assaad: Phase diagram of the SU(N) antiferromagnet of spin S on a square lattice. Phys. Rev. B 108, 2023. DOI: 10.1103/PhysRevB.108.115151.
Z. H. Liu, Y. D. Liao, G. Pan, M. Song, J. Zhao, W. Jiang, C.-M. Jian, Y.-Z. You, F. F. Assaad, Z. Y. Meng, and C. Xu: Disorder Operator and Rényi Entanglement Entropy of Symmetric Mass Generation. Phys. Rev. Lett. 132, 2024. DOI: 10.1103/PhysRevLett.132.156503.
A. Götz, M. Hohenadler, and F. F. Assaad: Phases and Exotic Phase Transitions of a Two-Dimensional Su-Schrieffer-Heeger Model. Phys. Rev. B 109, 2024. DOI: 10.1103/PhysRevB.109.195154.
Optics, Quantum Optics and Physics of Atoms, Molecules and Plasmas
Abstract
Accurate observation of two or more particles within a very narrow time window has always been a challenge in modern physics. It creates the possibility of correlation experiments, such as the
ground-breaking Hanbury Brown-Twiss experiment, leading to new physical insights. For low-energy electrons, one possibility is to use a microchannel plate with subsequent delay lines for the readout of the incident particle hits, a setup called a Delay Line Detector. The spatial and temporal coordinates of more than one particle can be fully reconstructed outside a region called the dead radius. For interesting events, where two electrons are close in space and time, the determination of the individual positions of the electrons requires elaborate peak finding algorithms. While classical methods work well with single particle hits, they fail to identify and reconstruct events caused by multiple nearby particles. To address this challenge, we present a new spatiotemporal machine learning model to identify and reconstruct the position and time of such multi-hit particle signals. This model achieves a much better resolution for nearby particle hits compared to the classical approach, removing some of the artifacts and reducing the dead radius by half. We show that machine learning models can be effective in improving the spatiotemporal performance of delay line detectors.
Scientific field: 308 Optics, Quantum Optics and Physics of Atoms, Molecules and Plasmas
Principal Investigator: Dr. Marco Knipfer, Friedrich-Alexander-Universität Erlangen-Nürnberg
Target system: GPGPU cluster Alex
Abstract
For the efficient usage of HPC applications in the exascale era, we need to improve the scalability on large and heterogeneous systems. This requires a variety of components, such as efficient processing, data storage, software, and algorithms. The goal of this BMBF-funded project is to develop new methods for reducing data traffic between compute nodes with distributed memory and storage in file systems on supercomputers. For this purpose, a co-design approach will be used to develop solutions for variable-precision computation, data compression and novel data formats. These solutions will be used to improve GENE, a program used worldwide for the simulation of plasma turbulence, and will be validated using GENE.
Scientific field: 308-01 Optics, Quantum Optics, Atoms, Molecules, Plasmas
Principal Investigator: Prof. Dr. Gerhard Wellein, Friedrich-Alexander-Universität Erlangen-Nürnberg
Target system: parallel computer Fritz & GPGPU cluster Alex
Particles, Nuclei and Fields
Abstract
We plan to study the thermalization of SU(2) gauge theory using techniques developed for quantum computing but on a classical computer, namely “Fritz”. More precisely we want to find out whether SU(2) (as prototype for all SU(N) gauge theories) fulfills the Eigenstate Thermalization Hypothesis (ETH). This is a highly relevant problem for many subfields of physics. It can be addressed on small lattice volumes and thus is one of the best candidates for an early demonstration of quantum supremacy. For this reason ingenious techniques have been developed (Natalie Klco, Duke University, is one of our collaborators) which allow us to check the validity of ETH for SU(2) on Fritz.
Scientific field: 309 Particles, Nuclei and Fields
Principal Investigator: Prof. Dr. Andreas Schäfer, University of Regensburg
Target system: parallel computer Fritz
Abstract
The High Energy Stereoscopic System (H.E.S.S.) telescopes in Namibia observe the very-high-energy gamma-ray sky from 20 GeV to 10s of TeV. H.E.S.S. operates by observing the Cherenkov light emitted when such a gamma-ray creates a particle cascade in the atmosphere; the nature, origin, and energy of the incident particle can be determined using image processing techniques. State-of-the-art deep learning methods allow for high-sensitivity image analysis at high-speed, by exploiting patterns in the data which are currently not being exploited, making them an attractive analysis prospect for such gamma-ray telescopes (DFG FU 1093/13-1).
The future Cherenkov Telescope Array (CTA) and Southern Wide-Field Gamma-Ray Observatories (SWGO) will also greatly benefit from these new analysis techniques. With this project, we aim to develop a variety of new analysis methods for the gamma-ray observatories H.E.S.S., CTA, and SWGO to improve event classification, reconstruction, and simulation.
Scientific fields: 309 Particles, Nuclei and Fields; 311 Astrophysics and Astronomy
Principal Investigator: Dr. Jonas Glombitza, Friedrich-Alexander-Universität Erlangen-Nürnberg
Target system: GPGPU cluster Alex
Abstract
In current and upcoming experiments (like the Electron Ion Collider) nucleon structure is extensively studied. Within the framework of lattice QuantumChromoDynamics (QCD) nucleon structure can be investigated from first principles utilizing large computational resources. Using this method we propose to compute (and improve on) the matrix elements related to Beyond-the-Standard-Model (BSM) interactions (which are not accessible experimentally) and (lower) moments of the parton distribution functions.
Scientific field: 309 Particles, Nuclei and Fields
Principal Investigator: PD Dr. Wolfgang Söldner, University of Regensburg
Target system: parallel computer Fritz
Project Report (01/2024)
Within the past NHR project we have made another great step forward in our large scale project of com- puting important matrix elements related to Beyond-the-Standard-Model (BSM) interactions (which are not accessible experimentally) and (lower) moments of the parton distribution functions. These computations are of great interest for current and upcoming experiments (like the Electron Ion Collider) with respect to nucleon structure. Utilizing the granted NHR resources we could improve on our statistical and systematic uncertainties in a particular relevant region in the parameter space that enters our fits from which we extract the final (physical) matrix elements.
Abstract
A better understanding of Double-Parton Distributions (DPDs) in the proton is vital to make full usage of the discovery potential of the LHC (CERN). We have pioneered corresponding lattice simulations with quite encouraging results, and we want to perform additional simulations with different lattice constants, which will allow for a continuum extrapolation. Without a reliable continuum extrapolation lattice results have, strictly speaking, no physical relevance.
Scientific field: 309-01 Nuclear and Elementary Particle Physics, Quantum Mechanics, Relativity, Fields
Principal Investigator: Prof. Dr. Andreas Schäfer, University of Regensburg
Target system: parallel computer Fritz
Project Report (03/2024)
The Large Hadron Collider at CERN, Geneva has so far failed to find “Beyond the Standard Model” physics (BSM). Therefore, its luminosity (basically its collision rate) is in the process of being much increased, to substantially enhance its sensitivity to BSM physics. As so far, one has not identified BSM physics, its effects, if they exist, must be very small. Consequently, it is of crucial importance to understand all normal, Standard Model, backgrounds to ever higher precision. In this context, Multi-Parton Interactions and in particular Double Parton Interactions are a major concern. These are processes in which two hard QCD reactions take place in the same proton-proton collision, such that (yet unknown) wave function effects become relevant. These are parameterized by Double-Parton Distributions (DPDs) and what we do is to calculate so-called invariant functions which in turn parameterize these DPDs. Because each quark can have 3 flavors and 3 spin orientations and one has two such quarks taking part in a double hard interaction there are many different DPDs and invariant functions, each of them being a function of several variables. Thus, DPDs contain very much information on the quark-gluon structure of a nucleon, of which one cannot predict which will become important for BSM searches but which makes them very interesting in their own right. We have pioneered the theoretical formalism which allows to calculate properties of DPDs using lattice QCD, which is the standard numerical method to calculate properties of specific hadrons from first principle Quantum Chromo Dynamics (QCD). As very little is known so far about DPDs, we focus for the time being on their general properties.
Fig.1 shows typical results for spin-independent invariant functions for the flavors down-down (dd), up-up (uu) and up-down (ud) combinations of quark flavors, as well as one interference DPD (yellow). Fig.2 shows the same when one of the quarks is spin polarized transversely to the proton momentum. The message of both figures is that we can determine all of these leading invariant functions of DPDs rather precisely. A proton has two valence up quarks but only one valence down quark, which explains why the up-up DPD is largest. Note that the interference DPD is not smaller in size than the down-down DPD. Fig.3 compares results for one specific invariant function for two lattices with different lattice constants. Its message is that the dependence on the lattice constant is very weak. Fig.4 compares the results for different quark masses.
The message here is that it is important to extrapolate the quark masses to their physical values what we will focus on next. In the long term the task would then shift to calculating the invariant functions of higher Mellin moments of DPDs, but this is not part of the present project.
Abstract
Experiments at particle accelerators have discovered new particles called XYZ states, which do not fit into the spectrum of conventional mesons made of a charm and an anti-charm quark (charmonium). Many of the new states are close to thresholds for strong decays. States made of gluons only, so called glueballs, are predicted by the theory of strong interactions (Quantum ChromoDynamics) but their existence still awaits an experimental confirmation. In this project we plan to study charmonium and glueballs by simulations of QCD on a lattice. The novelty of our study is the inclusion of light hadrons into which these states can decay. Our software has excellent scaling behavior on HPC systems.
Scientific field: 309-01 Particles, Nuclei and Fields
Principal Investigator: Prof. Dr. Francesco Knechtli, Bergische Universität Wuppertal
Target system: parallel computer Fritz
Project Report (04/2023)
The charmonium spectrum is of great importance to both particle physics and astrophysics, as it provides crucial information about the properties of the strong force and the nature of quarks. In particular, the hyperfine splitting in charmonium has long been a challenge for theoretical calculations, and accurate predictions are essential for interpreting experimental data and testing the standard model. By using cutting-edge techniques and state-of-the-art supercomputers, we aim to obtain results that are much more accurate than previous calculations and include effects that were so far neglected like mixing with light hadrons. Our results will provide new insights into the fundamental properties of the strong force and the nature of quarks, and will enable more accurate interpretation of experimental data from particle accelerators.
Statistical Physics, Soft Matter, Biological Physics, Nonlinear Dynamics
Abstract
Sudden Cardiac Death caused by, for example, malignant ventricular arrhythmias, results in an estimated 600.000 deaths per year in the European Community alone. However, the chaotic electrical excitation wave dynamics underlying such arrhythmias as ventricular fibrillation is not fully understood. It is known that fragments of (unstable) spiral or scroll waves drive the spatiotemporal dynamics during ventricular fibrillation and prevent coherent mechanical contraction of the heart.
Current clinical treatment consists of the application of a high-energy defibrillation shock delivered either from an external device, or from implantable cardioverter defibrillators (ICDs). In both cases, patients suffer from significant side effects due to this treatment, including additional tissue damage and post-traumatic stress.
In the last decades, several groups have been working on the development of alternative control strategies, with the aim to significantly reduce these side effects while reliably terminating the arrhythmia and restoring sinus rhythm. Many of these approaches use sequences of electrical stimulations of lower amplitude, in contrast to the conventional treatment consisting of a single high energy defibrillation shock. For example, pulse trains of electric low intensity stimuli with a specific frequency were used to terminate atrial fibrillation and ventricular fibrillation. Also several stages of differently timed pulse sequences were combined or feedback strategies were used.
Scientific field: 310 Statistical Physics, Soft Matter, Biological Physics, Nonlinear Dynamics
Principal Investigator: Prof. Dr. Thomas Lilienkamp, Technische Hochschule Nürnberg
Target system: GPGPU cluster Alex
Abstract
Project M02 focuses on multi-scale simulations of Interface-enhanced SILP and Advanced SCILL catalysis of hydrogenation reactions, to allow for intelligent design and optimisation of these technological systems. To this end, we will combine hybrid quantum mechanics calculations (chemical transformations), and molecular dynamics simulations (structuring & diffusivity), each thoroughly validated against the wealth of experiments performed in CLINT, to tune the functionality of the catalytic complex, the IL, and the support material. The aim is to identify, characterise and enhance effects, occurring on a multitude of molecular time and length scales, which favourably affect the overall reaction’s turn over.
Structuring of the IL and gas/liquid and liquid/solid interfaces creates a unique dynamic environment for the catalyst. We will therefore focus on developing an understanding of the structural and transport properties of functionalised ILs and reactant molecules within the film using molecular dynamics simulations. This will permit us to boost the spatial coordination of the reactants with the solid and the gas interfaces, and address issues of solubility, viscosity as well as wetting. Building on this understanding of the interface environment, we will devise a hybrid quantum mechanics/molecular mechanics (QM/MM) approach to embed local quantum characterisation of the reactive centre into inhomogeneous IL films.
Scientific field: 310 Statistical Physics, Soft Matter, Biological Physics, Nonlinear Dynamics
Principal Investigator: Prof. Dr. Ana-Suncana Smith, Friedrich-Alexander-Universität Erlangen-Nürnberg
Target system: GPGPU cluster Alex
Abstract
Robust self-assembly of nanoparticles can create nanostructures that have applications as structural color pigments and scaffolds for heterogeneous catalysis. This project develops and applies new methods to study not only the self-assembly process itself but also the properties of the self-assembled nanostructures. The computations in this proposal closely interact with experimental work that is conducted in the framework of the Collaborative Research Centre Design of Particulate Products.
Scientific fields: 310 Statistical Physics, Soft Matter, Biological Physics, Nonlinear Dynamics; 403/01 Chemical and Thermal Process Engineering
Principal Investigator: Prof. Dr. Michael Engel, Friedrich-Alexander-Universität Erlangen-Nürnberg
Target system: parallel computer Fritz & GPGPU cluster Alex
Project Report (06/2024)
This NHR@FAU project developed new advanced mesoscale particle models and applied them in molecular dynamics and Monte Carlo simulations to study the self-assembly of nanoparticles as well as the properties of the resulting nanostructures. Potential applications are in structural color pigments and heterogeneous catalysis. The project, involving four PhD students and one Master student, integrated computational research and experimental approaches of our collaborators in Germany and in the USA. PhD Student 1 discovered unique aperiodic crystals, including a six-fold symmetric chiral quasicrystal. PhD Student 2 enhanced powder diffraction techniques, improving structural analysis through advanced computational models. PhD Student 3 investigated the self-assembly of nanoparticles, developing methods to model interactions of anisotropic particles. PhD Student 4 studied the self-assembly of molecular monolayers on nanoparticles, focusing on how ligand density and arrangement affect interactions. Master Student 1 simulated the spray drying process to optimize the formation of nanoparticle powders. This comprehensive project, conducted within the Collaborative Research Centre Design of Particulate Products, significantly advanced our understanding of nanoparticle behavior and material properties.
Abstract
The computing time allocation supports two ongoing third-party funded projects (ERC & DFG) in our group, in which we investigate the statistical properties of fully developed turbulence. In the ERC project, we aim at developing new theoretical and computational approaches to better understand and model fully developed turbulence. The goal of the DFG project is to capture the large-scale dynamics of turbulent flows. Both projects use our code TurTLE, a pseudo-spectral solver of the Navier-Stokes equations which also features particle tracking capabilities.
Scientific fields: 310 Statistical Physics; 404-03 Fluid Mechanics
Principal Investigator: Prof. Dr. Michael Wilczek, Universität Bayreuth
Target system: parallel computer Fritz & GPGPU cluster Alex
Astrophysics and Astronomy
Abstract
Our project aims to address the computational challenges in simulating the response of neutrino telescopes, such as IceCube and KM3NeT, by developing a neural-network-based surrogate model. Traditional Monte Carlo simulations, particularly the simulation of Cherenkov light from high-energy neutrino interactions, are computationally intensive, often requiring extensive GPU resources. Our surrogate model will learn to parametrize detector responses, enabling more efficient event simulations. Additionally, it will facilitate design studies for next-generation detectors like P-ONE and IceCube-Gen2. By leveraging statistical tools, our framework will optimize detector design without the need for limiting the design parameter space or developing event selection and reconstruction algorithms.
Scientific field: 311 Astrophysics and Astronomy
Principal Investigator: Prof. Dr. Claudio Kopper, Friedrich-Alexander-Universität Erlangen-Nürnberg
Target system: GPGPU cluster Alex
Abstract
Red supergiant stars mark the late phases of massive stars. They are also the direct progenitors of Type II supernovae and gravitational wave sources. However, their mass loss mechanism remains elusive, largely due to the poorly-understood variable nature of their envelopes where convection interacts with large- amplitude pulsation. Such convective motions, driven by internal heating and external cooling, operate at a length scale comparable to the stellar radius. Therefore, global 3D simulations involving accurate radiation hydrodynamics are needed to model the inner structure and outer atmosphere of red supergiants. However, a grid of global red supergiant simulations that covers realistic stellar parameter space has never been computed before. Using the moving-mesh magnetohydrodynamic code AREPO, recently extended with the state-of-the-art radiation transport module, we perform a suite of global 3D radiation hydrodynamic simulations of red supergiant envelopes with updated physics. This suite will serve as the most up-to-date models to be compared with observations of red supergiants. It will also enable potential follow-up simulations of wind launching, shock breakout, common envelope, and binary mass transfer, which pose major uncertainties in our predictions of some transient events and gravitational wave sources.
Scientific field: 311 Astrophysics and Astronomy
Principal Investigator: Prof. Dr. Selma E. de Mink, Ludwig-Maximilians-Universität München
Target system: parallel computer Fritz
Abstract
Does a young planetary system carry the imprint of its birthplace in the diversity of our Galactic ecosystem? How does a specific environment or location in the Milky Way determines the properties of our Sun and solar system? ECOGAL will create the essential synergic framework where the extensive observational datasets available for our Galaxy can be analyzed back-to-back with state-of-the-art theoretical simulations of all Galactic environments, also using innovative machine-learning and decision-making tools. ECOGAL has the ambitious goal to build a unifying predictive model of the Milky Way ecosystem able to trace the properties of planet-forming disks back to the environmental conditions active in their birthplace. This project interweaves the unique expertise of four European research groups at the forefront of science in the four pillars of astronomy and astrophysics: observations, theoretical simulations, instrumentation and data analysis.
Scientific field: 311 Astrophysics and Astronomy
Principal Investigator: Dr. Noé Brucy, Heidelberg University
Target system: parallel computer Fritz
Project Report (04/2024)
The Milky is a complex system, with several components (gas, stars, dark matter) interacting together. In particular, the gaseous disk is undergoing gravitational forces for the stellar components. To better understand this interaction, we ran simulations of hydrodynamical and magneto-hydrodymical simulations of a Milky-way like galaxies, imposing two different external gravitational potentials to the gas. We found that the details of the potential made little difference in the global star formation rate of the galaxies, but are crucial to explain where the star formation take place. In the future, we aim to better understand how the potential influence the kinematic of the gas, as well as how the orbit of the gas and the stars are affected.
Publications
G. H. Hunter, M. C. Sormani, J. P. Beckmann, E. Vasiliev, S. C. O. Glover, R. S. Klessen, J. D. Soler, N. Brucy, P. Girichidis, J. Goller, L. Ohlin, R. Tress, S. Molinari, O. Gerhard, M. Benedettini, R. Smith, P. Hennebelle, and L. Test. Testing kinematic distances under a realistic Galactic potential. Investigating systematic errors in the kinematic distance method arising from a non-axisymmetric potential. Astronomy & Astrophysics. DOI: 10.1051/0004-6361/202450000.
Mathematics
Abstract
This compute time project delivers the necessary amount of core hours needed to provide realistic results of finite element simulations of a laser beam welding process.
In our project we consider a laser beam welding process. Short cycle times and small heat-affected zones have made this noncontact joining process a very viable option in the course of the increasing degree of automation in industrial production. However, due to the high cooling rate inherent in the process solidification cracks can occur. A quantitative understanding of the development mechanisms of these fractures and their correlation to process parameters is thus indispensable for the development and improvement of this process. The final goal of the project is to provide a complete simulation of this process that will gives us indicators under which conditions a crack can appear. A multiscale approach is needed to represent the properties and the behavior of the material and moreover efficient solver strategies are indispensable.
The development of efficient and robust numerical solver strategies, the parallel implementation and integration thereof into the software libraries FE2TI and PETSc is essential. In this computing time period, first large scale production simulations of laser beam welding processes using the FE2TI software are planned as well as optimizations and scalability tests of the integrated Schwarz domain decomposition preconditioners/solvers.
Scientific fields: 312 Mathematik; 402 Mechanics and Constructive Mechanical Engineering
Principal Investigator: Prof. Dr. Axel Klawonn, University of Cologne
Target system: parallel computer Fritz
Abstract
The goal of the project is to perform scale-resolving simulations of turbulent flow in wall-bounded geometries to gain new understanding of the flow behavior near boundaries and support future method development. The project PDExa-CFD uses high-order discontinuous Galerkin finite element discretizations in space and splitting implicit/explicit methods in time to discretize the incompressible Navier-Stokes equations. As test case, direct numerical simulation of the flow over a periodic hill will be computed at a Reynolds number Re=19,000 as well as limit studies towards even higher Reynolds numbers.
Scientific fields: 312 Mathematik; 404-3 Fluid Mechanics
Principal Investigator: Prof. Dr. Martin Kronbichler, Ruhr-Universität Bochum
Target system: parallel computer Fritz & GPGPU cluster Alex
Abstract
This project delivers the necessary computing time for two different research projects, both concerned with modelling and simulation of different aspects of arteries and blood flow using the same software framework.
In the first project, we aim at the robust computational modeling of coupling fluid-structure interaction with pharmacological effects towards an enhanced treatment of cardiovascular diseases. In the future, this could lead to a virtual laboratory to assist medical doctors and patients in their decisions. The main goal is to develop a robust numerical framework including suitable models for the computational simulation of the effects of drugs on the complex bio-chemo-mechanical processes in arterial walls.
In the second project, we aim to develop an efficient multiscale model for blood flow simulation. The main focus is on implementing a computational fluid model combining two different scales: a macroscopic scale which models blood as a continuous fluid, and a mesoscopic/microscopic scale capturing the behavior of red blood cells (RBCs) within the flow. We aim to improve computational efficiency of the multiscale model by identifying and implementing areas where advanced machine learning techniques can be applied.
The development of robust numerical coupling schemes and solver strategies and the parallel implementation and integration thereof into the software libraries FEDDLib, FROSch, and Trilinos is essential for both projects.
Scientific fields: 402 Mechanik; 312 Mathematik
Principal Investigator: Prof. Dr. Axel Klawonn, University of Cologne
Target system: parallel computer Fritz & GPGPU cluster Alex
Project Report (06/2024)
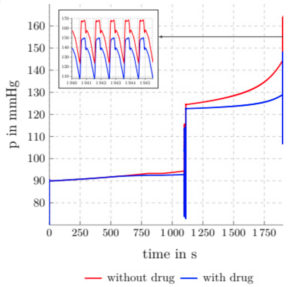
1) Phamaco-mechanical FSI
In “A computational framework for pharmaco-mechanical interactions in arterial walls using parallel monolithic domain decomposition methods, 2023” we presented our framework for the coupling between an antihypertensive drug modeled by a diffusion process and the mechanical response of an arterial wall modeled by a sophisticated smooth muscle model. The project can be divided into two parts. Part 1 being the realistic modeling of the arterial wall and accurately representing the effects certain drugs can have on the wall’s properties. Here, antihypertensive drugs were considered, which have an effect on the contractile behavior of the arterial wall, which in turn can lower the blood pressure. Consequently, a full pharmaco-mechanical fluid structure interaction (FSCI) framework was developed. With the previously granted compute time, numerous studies with respect to the mechanical properties were performed to analyze the influence of antihypertensive drugs on arterial wall. Figure 1 displays the blood pressure measured in the artery in a comparison with and without antihypertensive drug in our full FSCI framework. Furthermore, deeper numerical analysis especially with respect to more complex and realistic problem setting was carried out.
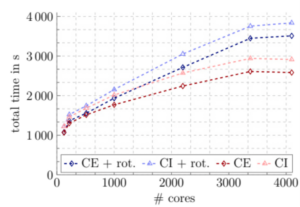
As such computations for full FSCI contain tens of thousands of time steps for modeling different stress and heart beat levels, scalable and efficient algorithms and solver strategies are necessary. This leads to Part 2 which focuses on developing such strategies. The previously mentioned, parallel scalable two-level overlapping Schwarz preconditioners with GDSW-type coarse spaces are implemented in the Trilinos package FROSch. The fully coupled FSCI system is solved iteratively via GMRES. As we can apply a block factorization to the system, we can precondition each arising sub problem with a different strategy. Consequently, with the previously granted compute time each subproblem was investigated separately to obtain the optimal solver configuration.
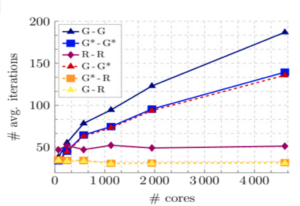
The monolithic pharmaco-mechanical system (SCI) was solved and investigated with respect to weak and strong scaling. The above mentioned paper contains the detailed description of the modeling of the arterial wall, the numerical treatment and solver strategies. Based on the results and the granted compute time the weak scaling for a test problem could be achieved (see Figure 2).
With respect to the fluid sub problem, the scaling properties were also analyzed. Here, the incompressible (generalized) Newtonian Navier-Stokes equations were considered. The weak scaling properties for the stationary case were shown for the backward-facing step example and a deeper analysis of the monolithic preconditioner was performed (see Figure 3; article is in preparation).
For the full FSCI problem, aside from the already presented qualitative results, further scaling tests combined with the results for the SCI and fluid subproblem will be performed.
2) Multiscale Model for Blood Flow
The current state of the research was partly presented at ECCOMAS in June 2024 with the title “Efficient Parallel Non-Newtonian Blood Flow Simulations in Trilinos – Towards Incorporating Microscopic Information with a Data-Driven Approach.” Generally the research can be subdivided into two main parts:
Incorporating Mescoscopic Information into Macroscopic Simulations for Blood Flow Predictions
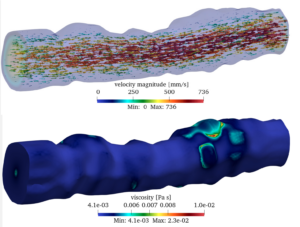
The first part involves simulating blood flow on a macroscopic scale to efficiently and accurately estimate field variables by incorporating mesoscopic information. Our initial approach involves a shear-rate dependent generalized-Newtonian fluid model for blood to simulate its shear-thinning behavior. The needed viscosity functions were obtained from our partner group at Forschungszentrum Jülich, who developed a virtual rheometer based on their mesoscopic solver that captures red blood cell dynamics. There are different viscosity functions for blood, as the viscosity depends not only on shear rate but also on factors such as hematocrit and the specific mechanics of healthy or diseased cells, all of which can be modeled by our partner group. But as a first step these were kept constant. Using the shear-rate dependent viscosity functions and our efficient macroscopic solver, we are able to run blood flow simulations capturing the shear thinning behavior.
As supported by literature, we conclude that for larger vessels, the shear-thinning effect is relatively small and the limiting viscosity has the most dominant effect on flow features. However, as the vessel size decreases or in pathological cases such as severe stenosis with recirculation zones, the non-Newtonian effects become increasingly significant. In the future, we aim to investigate smaller vessels in the regime of hundreds of micrometers. For these vessels, the non-homogeneous distribution of red blood cells and their effects become more important and should be incorporated into the model by simulating a non-constant hematocrit profile.
Numerical Aspects of Simulating Non-Newtonian fluid flow
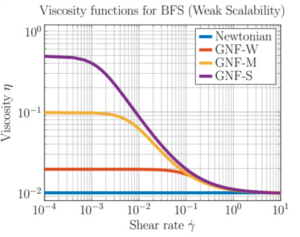
The second part of our research focuses on efficient simulation using scalable solvers. For this, we employ monolithic overlapping Schwarz preconditioners from the FROSCH package to solve the saddle point problem resulting from the discretization of incompressible, stationary flow of generalized-Newtonian fluids. For the nonlinear problem, we use the (globalized) Newton’s method from the NOX package in Trilinos.
Building on the results of previous projects by Lea Saßmannshausen et al., we used different coarse basis combinations to solve a backward-facing step flow case. These combinations, implemented in FROSCH, were applied to generalized-Newtonian fluid flow using viscosity functions of varying strengths (see Figure 5). Compared to Newtonian flow, we observed that reusing the coarse basis becomes increasingly inefficient, making it beneficial to compute the coarse basis based on the updated linearization after each Newton iteration.
In Figure 6, only the best combination, GDSW* for velocity and RGDSW for pressure, is indicated but all possible combinations were computed. We conclude that a combination of a large coarse space for velocity and a smaller for pressure is most efficient, as stated in the results of Lea Saßmannshausen et al. In future work, we aim to investigate the convergence of the nonlinear solver, as we have observed that for the strongest nonlinearity in viscosity, Newton’s method does not converge. Additionally, we will examine how a restart affects the reuse of the coarse basis.
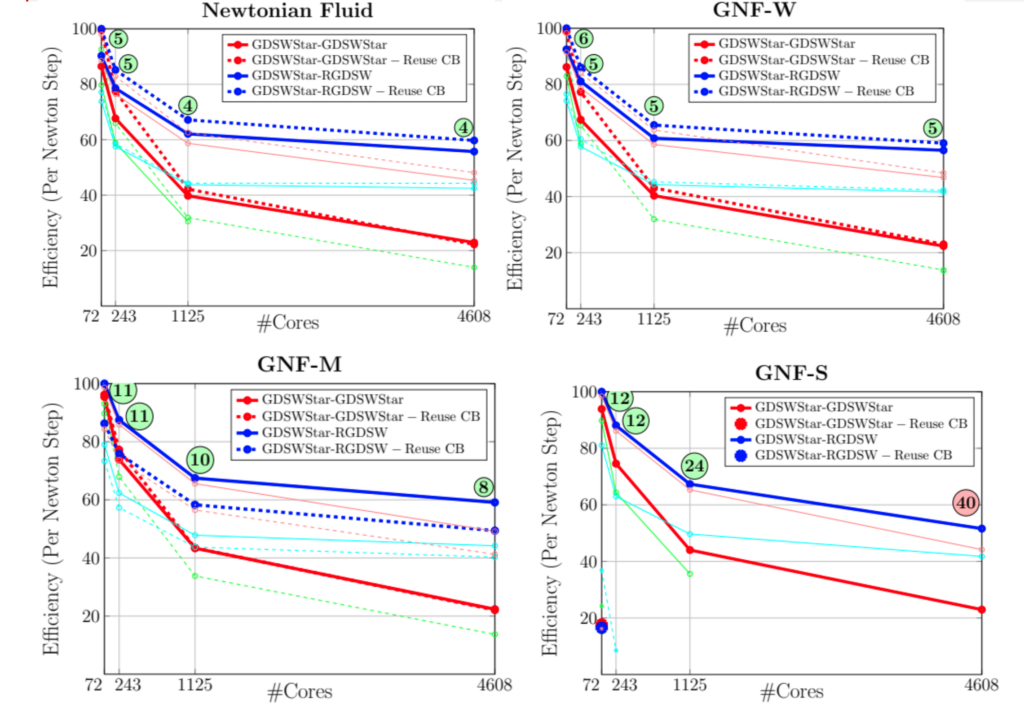
Abstract
Future Exascale computing architectures will feature a high number of heterogeneous hardware components that are comprised of special-purpose processors or accelerators. The corresponding realization of Computational Fluid Dynamics (CFD) application software as a central core component of nowaday’s CFD simulations in industrial context requires high-scaling methods. These methods solve high-dimensional and unsteady (non)linear systems of equations and need to be integrated into application software such that they can be used by non-HPC experts from the industry. Especially the open-source software FEATFLOW, is a central component of the StroemungsRaum platform that is successfully used by the industrial partner of the project IANUS for years. In the context of the whole project, FEATFLOW will be extended methodologically and by parallel near-hardware implementations.
Scientific fields: 404-03 Fluid Mechanics; 312 Mathematics
Principal Investigator: Prof. Dr. Stefan Turek, TU Dortmund University
Target system: parallel computer Fritz & GPGPU cluster Alex
Atmospheric Science, Oceanography and Climate Research
Abstract
The BigData@Geo 2.0 project aims to significantly increase the resilience of farms in the northern Bavarian agricultural sector through customized and spatially detailed adaptation measures to regional climate change. This requires the development of new modules of scientifically and methodologically sound transfer functions that translate changing climate indicators such as heat stress, UV exposure and late frost into operationally relevant metrics such as crop stress factors, risk exposure and crop yields. For this purpose, we develop data-driven climate models with Deep Learning and increase their spatial resolution for specific regions to field level with statistical downscaling. This enables the adaptation of the trained climate models to forecast crucial performance indicators for agricultural enterprises in the northern Bavarian region.
Scientific fields: 313 Atmospheric Science, Oceanography and Climate Research; 409 Computer Science
Principal Investigator: Prof. Dr. Andreas Hotho, Julius-Maximilians-Universität Würzburg
Target system: GPGPU cluster Alex
Abstract
The project “Detection and Attribution of climate change for the glaciers on Kilimanjaro: Targeting the processes at regional and local scales” is attempting to break down the concept of Detection & Attribution to the local scale, by using the case study of glacier change on Kilimanjaro. This is because (a) the long research record for this place and (b) the unique climate indicator potential of these glaciers on a freestanding peak in the tropical mid troposphere. Results will reveal how human influence on the climate is transferred to the site-specific level.
The project “Exploring the potential of coralline algae as climate proxy and for climate model evaluation: a Southern Hemisphere case study of New Zealand” aims to explore a novel climatic indicator, namely crustose coralline algae that grow in shallow ocean waters (CCA), for the purpose of improved global climate model (GCM) evaluation. In particular, we will target the improvement potential with regard to sea surface temperatures in the Southern Ocean and the effect of these ocean conditions on the regional high-mountain climate of New Zealand.
Scientific field: 313-01 Atmospheric Science
Principal Investigator: Prof. Dr. Thomas Mölg, Friedrich-Alexander-Universität Erlangen-Nürnberg
Target system: parallel computer Fritz
Project Report (03/2024)
In the first 1.5 years, several successful simulations were conducted in the project ATMOS. These were mostly based on the numerical atmospheric model WRF, a well-known community model. The main progress has comprised (i) the development of new modules for WRF to implement important (yet thus far, neglected) processes, especially with regard to cold climates; (ii) the creation of a new regional climate data set for New Zealand over the period 2005-2020 with a focus on the Southern Alps; and (iii) insights into cloud processes that are tied to radiation effects and the isotopic composition of water.
Publications
M. Andernach, J. Turton, and T. Mölg: Modeling cloud properties over the 79N Glacier (Nioghalvfjerdsfjorden, NE Greenland) for an intense summer melt period in 2019. Quarterly Journal of the Royal Meteorological Society 148, 2022. DOI: 10.1002/qj.4374.
E. Kropač, T. Mölg, and N. J. Cullen: A new, high-resolution atmospheric dataset for southern New Zealand, 2005–2020. Geoscience Data Journal 11(4), 2024. DOI: 10.1002/gdj3.263.
M. Saigger, T. Sauter, C. Schmid, E. Collier, B. Goger, G. Kaser, R. Prinz, A. Voordendag, and T. Mölg: A Drifting and Blowing Snow Scheme in the Weather Research and Forecasting model. Journal of Advances in Modeling Earth Systems 16(6), 2024. DOI: 10.1029/2023MS004007.
N. Landshuter, F. Aemisegger, T. Mölg: Stable Water Isotope Signals and Their Relation to Stratiform and Convective Precipitation in the Tropical Andes. Journal of Geophysical Research Atmospheres 129(14), 2024. DOI: 10.1029/2023JD040630.
Geophysics and Geodesy
Abstract
With the advent of the digital era, especially the widespread adoption of Web 2.0 platforms, social media has emerged as a new avenue for studying urban morphological transformations. Traditionally, urban morphology focuses on interpreting the physical structures of cities, such as buildings, streets, and public spaces, which are tangible evidences of cities as human habitats. However, modern cities are not only collections of physical spaces but also venues for cultural and emotional exchanges. This study utilizes social media data as a tool to investigate the relationship between urban form and residents’ opinions and emotions. By comparing social media discussions from different types of urban morphology, this study reveals the potential impacts of physical environments on residents’ opinions. This research not only enriches the scope of traditional urban morphology studies but also offers new perspectives and data support for urban planning and
environmental policies.
Scientific fields: 315-02 Geophysics and Geodesy; 410-02 Construction Engineering and Architecture
Principal Investigator: Dr. Richard Lemoine Rodriguez, Julius-Maximilians-Universität Würzburg
Target system: parallel computer Fritz & GPGPU cluster Alex
Physical Chemistry
Theoretical Chemistry
Abstract
The project aids the design of new electrodes for photoelectrochemical water oxidation by studying the underlying electronic processes. It collaborates with experimental partners in Sonderforschungsbereich 1585. The electronic coupling between different molecules and nano-particles, as well as the charge transport through the particles and the interfaces will be studied using the real-time propagation approach to time-dependent density functional theory. By simulating electron dynamics in real-time and on a real-space grid, the project aims at unravelling charge-transport pathways in complex, interface-governed materials. Effort will also be devoted to use and develop exchange-correlation approximations that combine computational efficiency with a reasonable accuracy for long-range charge-transfer.
Scientific field: 327-01 Electronic Structure, Dynamics, Simulation
Principal Investigator: Prof. Dr. Stephan Kümmel, Universität Bayreuth
Target system: parallel computer Fritz
Engineering Sciences
Mechanics and Constructive Mechanical Engineering
Abstract
In this project, we focus on advancing computational fluid dynamics (CFD) by developing numerical algorithms optimized for exascale computing environments. The objective is to enhance the precision, parallel efficiency, and energy efficiency of CFD simulations. The project aims at ensuring readiness for exascale performance. A key aspect of the project is to improve energy efficiency contributing to sustainable computing practices. Using the developed algorithms, six different lighthouse cases of physical and engineering interest, ranging from aeronautical to atmospheric flows will be simulated. FAU-LSTM is the lead for lighthouse case 6, which will simulate the flow around a model merchant ship’s hull, and also involved in lighthouse case 3 devoted to topology optimisation. In addition FAU-LSTM is workpackage leader of WP4, exascale techniques, dealing with novel subgrid-scale models, in-situ analysis and uncertainty quantification, all in view of exascale applications.
Scientific fields: 404-03 Fluid Mechanics; 402-04 Acoustics
Principal Investigator: Prof. Dr. Philipp Schlatter, Friedrich-Alexander-Universität Erlangen-Nürnberg
Target system: parallel computer Fritz & GPGPU cluster Alex
Abstract
FRASCAL improves understanding of fracture in complex heterogeneous materials by developing simulation methods able to capture the multiscale nature of failure. With i) its rooting in different scientific disciplines, ii) its focus on the influence of heterogeneities on fracture at different length and time scales as well as iii) its integration of highly specialized approaches into a “holistic” concept, FRASCAL addresses a truly challenging cross-sectional topic in mechanics of materials.
Specifically, project P8 (“Fracture in Polymer Composites: Meso to Macro”) aims to study the influence of different mesoscopic parameters, including micro-structure morphology, on the macroscopic fracture properties of nano-particle reinforced polymers.
Scientific field: 402-2 Mechanics
Principal Investigator: Prof. Dr.-Ing. Julia Mergheim, Friedrich-Alexander-Universität Erlangen-Nürnberg
Target system: parallel computer Fritz
Project Report (11/2023)
Modelling and Computation of Fracture in Silica-Polymer Composites: From Meso to Macro Scale
Motivation: The mechanical properties and the fracture toughness of polymers can be enhanced by adding silica particles or other fillers. These nano- or micro-particles increase the elastic and especially the fracture properties of the material. However, the elastic properties exhibit a size-effect that cannot easily be modelled with classical continuum mechanics and homogenization approaches. The experimentally observed increase in the yield strength and the fracture properties is due to different mechanisms. Complex crack paths develop on the mesoscopic scale, accompanied by localized shear bands, particle debonding, and void growth, which are strongly influenced by the distribution of the particles. Modelling and simulation of the mesoscopic fracture behavior are thus essential for the determination of macroscopic fracture properties.
Vision and Objectives: The aim of the project is to study the influence of silica particles and other fillers on the elastic and fracture properties of polymer composites. Therefore, a multiscale simulation approach will be developed to derive the macroscopic properties of the composite, depending on the underlying mesostructures. A numerical homogenization approach to determine the elastic properties, including size effects, was developed and will be extended to determine the macroscopic fracture toughness of polymer composites based on mesoscopic crack simulations.
State of the Art: The mechanical properties and the fracture toughness of polymer composites depend strongly on their underlying mesostructure. In particle-reinforced thermosets, different mechanisms are responsible for the observed increase in fracture toughness and yield stress, e.g. localized shear bands, debonding of particles, and subsequent void growth in the matrix material. The increases in strength and fracture energy have been shown to depend on various mesoscopic characteristics, including the volume fraction and the geometric arrangement of the particles. Simulation of the fracture behavior on the mesoscale requires a flexible method that can represent crack propagation in heterogeneous materials, the emergence of new cracks, and crack bifurcations. The phase-field method for fracture offers these possibilities and can be coupled with regularized cohesive models to represent debonding. Multiscale approaches for fracture mainly use the variational multiscale method or related approaches, which will also be applied in the present project, or they are based on homogenization methods.
Work plan: An extended homogenization method to account for size effects in polymer composites has been successfully developed. This method includes interfaces or interphases of a finite thickness with their own mechanical properties around the particles on the mesoscopic scale. We showed that for materials with stiffer particles only the interphase-approach yields the desired size effect. The phase-field approach for fracture has been extended by means of different spatial adaptivity methods. Error indicators based on phase-field threshold values, the gradient of the phase-field, and configurational forces are introduced and compared in terms of accuracy and efficiency. Next, in the project, a multiscale simulation approach for fracture of polymer composites (epoxy with silica particles and cellulose-polymer composites) will be developed and applied considering the mentioned research results.
Abstract
The research project comprises the acoustics of a radial fan operated in a spiral housing. Radial fans have a wide range of applications in a wide variety of fluid engineering applications, in which the requirements for sound radiation are becoming increasingly dominant.
The goal of the present application is to investigate the multiphysical interrelationships of flow-related sound radiation of radial fans in volute casings using a combined, experimental, simulation-based approach. It is important to treat the excitation paths for the casing structure separately: There are the fluid dynamic pressure fluctuations as well as the flow induced acoustic pressure field within the casing.
The numerical simulation methods are verified and validated against experimentally obtained data. Subsequently, developed methods are used to investigate the sound generation in the fan impeller and the resulting sound radiation through the spiral housing. This is done by means of variant studies. The variants are formed by different inflow conditions to the radial impeller, which influence the flow-induced sound generation in the impeller and thus also the casing excitation and sound radiation into the far field.
The innovation of the research work results from the fact that for the first time it is possible to develop a simulation method for enclosed rotating systems, which maps the entire chain of fluid-structure-acoustic interaction. In a complementary approach with experimental investigations, a conscious separation of different mechanisms in sound generation and their complex interactions results in knowledge with a high general validity that is not yet available in literature for enclosed radial fans. The findings allow conclusions to be drawn as to which part of the machine (impeller flow, casing structure, etc.) changes the radiated sound with which influencing parameters. In the development process, this results in a specific treatment of the acoustic problem areas.
The basic-oriented investigations have a high general validity and form the basis of future design rules for noise-reduced radial fans. The coupled simulation method provides a calculation tool that allows the flow- induced sound generation and its propagation in radial impellers to be analyzed in the frequency and time domain.
Future work will focus on the use of optimization algorithms for noise reduction, which consider not only the inflow conditions to the impeller and their interaction with the leading edge of the blade, but also the material parameters and the shape of the casing structure.
Scientific fields: 404-03 Fluid Mechanics; 404-04 Hydraulic and Turbo Engines and Piston Engines; 402-04 Acoustics
Principal Investigator: apl. Prof. Dr.-Ing. habil. Stefan Becker, Friedrich-Alexander-Universität Erlangen-Nürnberg
Target system: parallel computer Fritz
Abstract
FRASCAL improves understanding of fracture in brittle heterogeneous materials by developing simulation methods able to capture the multiscale nature of failure. With i) its rooting in different scientific disciplines, ii) its focus on the influence of heterogeneities on fracture at different length and time scales as well as iii) its integration of highly specialized approaches into a “holistic” concept, FRASCAL addresses a truly challenging cross-sectional topic in mechanics of materials.
In particular, sub-project P6 (“Fracture in Thermoplastic Polymers: Discrete-to-Continuum Coupling”) provides a link between the level of atoms and the continuum with specific interest in the multiscale modelling and simulation of polymer fracture.
Scientific field: 402 Mechanics and Constructive Mechanical Engineering
Principal Investigator: PD Dr.-Ing. habil. Sebastian Pfaller, Friedrich-Alexander-Universität Erlangen-Nürnberg
Target system: parallel computer Fritz & GPGPU cluster Alex
Project Report (09/2024)
The present project complements project P6 “Fracture in Thermoplastic Polymers: Discrete-to-Continuum Coupling” of the Research Training Group GRK 2423 “Fracture across Scales – FRASCAL”.
The first focus is on further development and enhancement of the domain-decomposition multiscale “Capriccio” method, which couples a particle-based domain treated by molecular dynamics (MD) with a continuum solved by the finite element (FE) method. In contrast to classical MD procedures, the particle- based domain is subjected to non-periodic boundary conditions which enables application of non-affine deformations typically not feasible in MD. In this regard, extensive parameter studies have been performed to reveal an optimal setting of the coupling parameters both for the equilibration and deformation phases of the numerical samples. Specific attention has been paid to the performance of the particle-based domain under the non-periodic boundary conditions. These studies during equilibration have been recently published. Further activities to gain deeper understanding of the coupling and the effect of parameters on the results compare the fully coupled three-dimensional system to a simplified one-dimensional set-up. By these comparative studies, the relevant factors of influence can be separately investigated and strategies to reduce deviations introduced by the coupling can be developed. In this context, one project and one master thesis revealed important insights. Currently, two manuscripts are prepared on the findings of these studies. In summary, these activities resulted in a deep understanding of the influence of the staggered FE-MD solution scheme applied in the Capriccio method and to appropriately choose the coupling parameters at the method’s current stage. Additional, preliminary work to extend the Capriccio method to thermal coupling has been done by thoroughly investigating the time-temperature superposition in amorphous thermoplastics at large strains by means of pure MD simulations (https://doi.org/10.1016/j.mechmat.2024.104926). In parallel, the original version of the Capriccio code has been carefully revised, which resulted in a significant increase of efficiency. Meanwhile, this updated version of the Capriccio code is publicly available at Zenodo (https://doi.org/10.5281/zenodo.12606758).
The second focus is on fracture simulations of amorphous materials and comprises several sub-studies. On the one hand, numerical studies using pure MD have been performed. Here, we specified appropriate criteria for bond breakage in generic thermoplastic polymer systems (https://doi.org/10.1016/j.engfracmech.2024.110270) and investigated the influence of the average molar mass and the dispersity (https://doi.org/10.1016/j.euromechsol.2024.105379) . Such generic models may use simplified formulations for the interactions between molecules and atoms, but still cover the main characteristics of polymer materials. By our studies on the HPC cluster, we were able to obtain trends similar to those known from experiments. On the other hand, by choosing such generic polymer models at molecular level, also computationally efficient coupled FE-MD simulations are feasible. In this regard, fracture simulations using the Capriccio method are currently performed, using thermoplastic polymers and silica glass as exemplary amorphous materials. Here, only the vicinity of the crack tip is resolved at molecular level, whereas the remaining part is treated as a continuum. By this set-up, flexible application of boundary conditions enables simulations mimicking typical set-ups used in fracture mechanical applications. In addition, we performed studies to integrate domain adaptivity into the Capriccio method, such that finely resolved MD domains can move throughout the entire domain and have to be used only on parts of high loads.
Project Report (09/2023)
The first focus is on further development and enhancement of the domain-decomposition multiscale “Capriccio” method, which couples a particle-based domain treated by molecular dynamics (MD) with a continuum solved by the finite element (FE) method. In contrast to classical MD procedures, the particle- based domain is subjected to non-periodic boundary conditions which enables application of non-affine deformations typically not feasible in MD. In this regard, extensive parameter studies have been performed to reveal an optimal setting of the coupling parameters both for the equilibration and deformation phases of the numerical samples. Specific attention has been paid to the performance of the particle-based domain under the non-periodic boundary conditions. These studies during equilibration have been recently published. Further activities to gain deeper understanding of the coupling and the effect of parameters on the results compare the fully coupled three-dimensional system to a simplified one-dimensional set-up. By these comparative studies, the relevant factors of influence can be separately investigated and strategies to reduce deviations introduced by the coupling can be developed. In this context, one project thesis has been completed and a master thesis is currently in progress. Depending on the findings of the latter one, one or two manuscripts will result from these studies. Additional, preliminary work to extend the Capriccio method to thermal coupling has been done by thoroughly investigating the time-temperature superposition in amorphous thermoplastics at large strains by means of pure MD simulations. An associated manuscript is currently under review. In parallel, the original version of the Capriccio code has been carefully revised, which resulted in a significant increase of efficiency. In summary, these activities shall eventually result in a user-oriented user-oriented guideline for setting up coupled simulations together with an appropriate choice of parameters, which is an essential precondition to make the Capriccio method publicly available in an open repository.
The second focus is on fracture simulations of thermoplastic polymers and comprises several sub-projects. In the first one, numerical studies have been performed to specify appropriate criteria for bond rupture in thermoplastic polymer systems. Due to their complexity, these investigations are still ongoing. The second sub-project concentrates on double-notched numerical specimens inspired by classical fracture mechanical set-ups. Here, we were able to obtain trends of mechanical properties of polymer nanocomposites similar to those known from experiments. The related manuscript is currently in preparation. Furthermore, fracture simulations using the Capriccio method are currently prepared. Here, only the vicinity of the crack tip is resolved at molecular level, whereas the remaining part is treated as a continuum. By this set-up, flexible application of boundary conditions will become possible in order to run simulations mimicking typical set-ups used in fracture mechanical applications. In addition, we performed studies to integrate domain adaptivity into the Capriccio method, such that finely resolved MD domains can move throughout the entire domain and have to be used only on parts of high loads.
Our third focus is on accelerating the coupled MD-FE simulations by choosing generic polymer models at molecular level. Such generic models may use simplified formulations for the interactions between molecules and atoms, but still cover the main characteristics of polymer materials. By our studies on the HPC cluster, we were able to reveal trends observed in experiments when investigating the influence of the average molar mass, the dispersity, the filler particle size as well as the filler particle content on the mechanical properties of thermoplastics and their nanocomposites. In this context, two manuscripts are currently in preparation.
Publications
M. Ries, L. Laubert, P. Steinmann, and S. Pfaller: Impact of the unimodal molar mass distribution on the mechanical behavior of polymer nanocomposites below the glass transition temperature: A generic, coarse-grained molecular dynamics study. European Journal of Mechanics – A/Solids 107, 2024. DOI: 10.1016/j.euromechsol.2024.105379.
W. Zhao, P. Steinmann, and S. Pfaller: Modeling steady state rate- and temperature-dependent strain hardening behavior of glassy polymers. Mechanics of Materials 195, 2024. DOI: 10.1016/j.mechmat.2024.105044.
F. Weber, V. Dötschel, P. Steinmann, S. Pfaller, and M. Ries: Evaluating the impact of filler size and filler content on the stiffness, strength, and toughness of polymer nanocomposites using coarse-grained molecular dynamics. Engineering Fracture Mechanics 307, 2024. DOI: 10.1016/j.engfracmech.2024.110270.
E. Richter, G. Possart, P. Steinmann, S. Pfaller, and M. Ries: Revealing the percolation-agglomeration transition in polymer nanocomposites via MD-informed continuum RVEs with elastoplastic interphases. Composites Part B 281, 2024. DOI: 10.1016/j.compositesb.2024.111477.
W. Zhao, R. Xiao, P. Steinmann, and S. Pfaller: Time-temperature correlations of amorphous thermoplastics at large strains based on molecular dynamics simulations. Mechanics of Materials 190, 2024. DOI: 10.1016/j.mechmat.2024.104926.
J. Seibert, S. Pfaller, and M. Ries: Investigation of the influence of nano-sized particles on the entanglement distribution of a generic polymer nanocomposite using molecular dynamics. Mathematics and Mechanics of Solids 29, 2024. DOI: 10.1177/10812865231206547.
V. Dötschel, S. Pfaller, and M. Ries: Studying the mechanical behavior of a generic thermoplastic by means of a fast coarse-grained molecular dynamics model. Polymers and Polymer Composites 31, 2023. DOI: 10.1177/09673911231208590.
F. Weber, M. Ries, C. Bauer, C. R. Wick, and S. Pfaller: On equilibrating non-periodic molecular dynamics samples for coupled particle-continuum simulations of amorphous polymers. Forces in Mechanics 10, 2023. DOI: 10.1016/j.finmec.2022.100159.
S. Pfaller, M. Ries, W. Zhao, C. Bauer, F. Weber, and L. Laubert: CAPRICCIO – Tool to run concurrent Finite Element-Molecular Dynamics Simulations (2.0.1). Zenodo, 2024. DOI: 10.5281/zenodo.12606758.
In Review
W. Zhao, Y. Jain, F. Müller-Plathe, P. Steinmann, and S. Pfaller: Unraveling the origin of fracture in glassy polymers using coupled particle-continuum simulations.
In Preparation
F. Weber, M. Vassaux, L. Laubert, and S. Pfaller: Quantitative and chemically specific simulations of Mode I and Mode III fracture properties of oxide glasses.
Process Engineering, Technical Chemistry
Fluid Mechanics, Technical Thermodynamics and Thermal Energy Engineering
Abstract
Electrolytes conduct electric current by the movement of ions while blocking the free movement of electrons. For applications of electrolytes as working fluids, the transport of ions is important and can be subdivided into diffusion, convection, and, in the presence of an electric field, migration. The diffusion process has shown to be of special interest since it is a limiting factor for the performance of applications where large concentration gradients can build up when electro-active ions react at electrode surfaces. Therefore, exact knowledge of molecular diffusion coefficients is required for the design and optimization of efficient processes involving electrolytes.
Scientific fields: 404-01 Energy Process Engineering; 404-02 Technical Thermodynamics
Principal Investigator: Prof. Dr.-Ing. habil. Andreas Paul Fröba, Friedrich-Alexander-Universität Erlangen-Nürnberg
Target system: parallel computer Fritz & GPGPU cluster Alex
Project Report (10/2024)
The main aim of the intended research project is to gain a fundamental understanding of the diffusive mass transport in electrolyte systems. For this, DLS experiments are combined with EMD simulations to characterize the diffusive mass transport by the determination of molecular diffusion coefficients of systematically selected electrolyte systems. Here, diffusion coefficients for mixtures containing ions and molecules with varying size, structure, and charge distribution are intended to be studied over a broad temperature and composition range at macroscopic thermodynamic equilibrium. For electrolytes containing ions with a permanent electrostatic charge, special focus lies on the evaluation of the influence of intermolecular electrostatic interactions on the diffusive process. While EMD simulations are able to quantify this contribution, they strongly rely on the underlying force fields (FFs). In the further development and testing of existing FFs in this project, a special focus lies on the combination of compatible FFs for the different species of interest allowing a sound description of the interactions relevant in electrolyte mixtures. To access accurate diffusion coefficients by DLS, which also serve for the validation and development of the simulations, the technique should be further developed for the reliable measurement of diffusivities in electrolyte systems. A further aim of the proposed project is the simultaneous characterization of the mass transfer related diffusion on a molecular level and diffusion of clusters. For this, light scattering experiments will be further developed to allow simultaneously access to molecular and cluster diffusion coefficients by extending the accessible range of wave numbers for DLS experiments and by combining DLS with Raman spectroscopy. The investigation of density fluctuations at large wave numbers is necessary because the life times of such fluctuations related to the diffusion of clusters are larger than those related to molecular diffusion. In order to investigate the formation of clusters by Raman scattering, the shift and broadening of Raman lines associated with bonds that are involved in the cluster formation should be studied and compared to RDFs accessible by EMD simulations. It is a further objective to answer the question if cluster diffusion and molecular diffusion are related to each other. The achievement of the aforementioned aims should also support the intended development of a predictive model for the molecular diffusion coefficient in electrolyte systems. Here, the diffusivities measured and calculated by DLS and EMD simulations should serve as a reliable database.
Publications
C. J. Kankanamge, T. Klein, and A. P. Fröba: Influence of the Molecular Characteristics of the Solvent and the Solute Cation on the Diffusive Mass Transport in Binary Electrolyte Mixtures by Dynamic Light Scattering and Molecular Dynamics Simulations. Journal of Electrochemical Society, 2024. DOI: 10.1149/1945-7111/ad92df. accepted
C. J. Kankanamge, T. Zhan, M. Piszko, T. Klein, and A. P. Fröba: Diffusion coefficients in binary electrolyte mixtures by dynamic light scattering and molecular dynamics simulations. Electrochimica Acta 462, 2023. DOI: 10.1016/j.electacta.2023.142637.
Materials Science
Abstract
Phase diagrams have revolutionized materials development by providing the conditions for phase stabilities and transformations, and thereby a thorough thermodynamic understanding of materials design. However, the majority of today’s phase diagrams are based on scarce experimental input and often rely on daring extrapolations. Every multicomponent phase diagram relies on a fragile set of phase stabilities as very recent studies show.
Within this project, materials design is raised to the next level by providing a highly accurate first principles thermodynamic database. First principles, alias ab initio, approaches do not require any experimental input and can operate where no experiment is able to reach. However, they have been limited to zero-Kelvin or low-temperature approximations which are not representative of phase diagrams.
Utilizing our unique expertise in high-accuracy finite-temperature ab initio simulations, we have developed an extremely efficient procedure including novel methods accelerated by machine learning potentials. This procedure facilitates a highly efficient determination of Gibbs free energies, thereby allowing convergence also of properties depending on the second-order derivatives, such as the heat capacity and thermal expansion.
With these tools, we have now reached a stage where a large ab initio thermodynamic database will be computed for elements across the periodic table. The main focus will be on phase stabilities of various phases, including dynamically unstable ones. Having acquired such a database, the phase stabilities can be put into practice by re-parametrizing binary phase diagrams and studying the implications on multicomponent phase diagrams.
Scientific field: 406 Materials Science
Principal Investigator: Prof. Dr. Blazej Grabowski, University of Stuttgart
Target system: parallel computer Fritz
Project Report (01/2024)
1) Thermodynamic properties on the homologous temperature scale from direct upsampling: Understanding electron-vibration coupling and thermal vacancies in bcc refractory metals
In this work, we present thermodynamic properties (heat capacity, thermal expansion, bulk modulus; see example figure below) of four bcc refractory elements (V, Ta, Mo and W) up to melting point to full density-functional-theory (DFT) accuracy (including anharmonic interactions) within the PBE exchange-correlation functional. The results are also analyzed on a self-consistently determined homologous temperature scale, and we get remarkable agreement to experimental data from literature. Such an accuracy to high-temperature experimental data has never been achieved before for refractory systems, and it exemplifies the strength of the recently proposed methodology (direct upsampling) and the possibilities for developing ab initio thermodynamic databases.
Additionally, we have calculated vacancy formation Gibbs energies, and included the effect of vacancies on bulk thermodynamic properties. We also formulate and individually calculate, for the first time, the coupling between thermal vibrations and electronic excitations—a crucial concept that refines our understanding of free energy contributions.
2) Machine-learning-accelerated ab initio simulations of dynamically stabilized phases
Imagine a top that becomes stabilized in an up-right position by spinning on a table. Similarly, in materials physics, certain phases have their atoms vibrating around the static lattice positions to achieve a stabilization at elevated temperatures (see the figure below). In this study, we develop an advanced method accelerated by machine-learning potentials to compute thermal properties of such dynamically stabilized phases with quantum-mechanical accuracy. We apply it to the bcc phase in the prototype systems Ti, Zr, and Hf, with a particular focus on the solid phase transition from hcp to bcc. Our results resolve a long-standing discrepancy at 0 K between experimental and theoretical properties, providing valuable insights for both experimental studies and engineering applications.
3) Performance and limits of finite temperature DFT for SiO2
The direct upsampling approach is able to accurately predict the thermodynamic properties of materials at the full accuracy level of density-functional theory (DFT), which includes contributions of higher order than the harmonic approximation. Our approach has been applied to face-centered cubic (fcc), body-centered cubic (bcc), and hexagonal closed-pack (hcp) systems so far. The investigated materials include refractories, as well as dynamically unstable systems. However, this approach has been applied only to unary systems. Here we apply it also to the binary SiO2 system, specifically to the crystalline polymorphs. The DFT level accuracy is achieved for a series of exchange-correlation functionals. In particular, the flat energy-volume curve, which correlates with the low bulk modulus, makes the description of the thermodynamic properties very challenging. The theoretical calculation of the temperature dependent volume expansion agrees well with the experiment, but only when an appropriate exchange-correlation functional is used. The phase stabilities in comparison with CALPHAD show the results of the current exchange correlation functionals, their predictions and limitations, and call for further development of the functionals for finite temperature free-energy calculations.
Publications
A. Forslund, J. H. Jung, P. Srinivasan, and B. Grabowski: Thermodynamic properties on the homologous temperature scale from direct upsampling: Understanding electron-vibration coupling and thermal vacancies in bcc refractory metals. Physical Review B 107, 2023. DOI: 10.1103/PhysRevB.107.174309.
J. H. Jung, A. Forslund, P. Srinivasan, and B. Grabowski: Dynamically stabilized phases with full ab initio accuracy: Thermodynamics of Ti, Zr, Hf with a focus on the hcp-bcc transition. Physical Review B 108, 2023. DOI: 10.1103/PhysRevB.108.184107.
Systems Engineering
Abstract
Several studies have been conducted to resolve the flow over square riblets using numerical solvers based on second-order methods, overlapping methods and immersed boundary methods; yet, there is no study which systematically compares the effectiveness of different approaches in resolving the flow and their computational performance. In this study, we compare the accuracy and performance of high-order methods for resolving roughness due to square riblet in a turbulent channel flow using four different approaches, AMR, conformal, IBM, and overlapping at Rebulk=10000. Further, we expand this studies to simulation of heterogeneous roughness in pipe flows using Nek5000, a spectral code for a better accuracy at Rebulk=11700.
Scientific field: 404-03 Microsystems
Principal Investigator: Venkatesh Pulletikurthi, Ph.D., Friedrich-Alexander-Universität Erlangen-Nürnberg
Target system: parallel computer Fritz
Abstract
Innerhalb der zerstörungsfreien Werkstoffprüfung ist die Computertomographie (CT) der Goldstandard, um Bauteile schnell und präzise zu vermessen und auf Fehler zu analysieren. Ein typischer Inspektionsablauf ist in der folgenden Abbildung dargestellt. Die bislang etablierte Rekonstruktions-Software kämpft regelmäßig mit Problemen, bei denen das 3D- Modell nicht mit dem realen Objekt übereinstimmt. Diese treten besonders bei Verbundmaterialien aus Kunststoff und Metall auf und machen die Qualitätssicherung schwierig und zeitaufwendig. Zugleich scheitert bestehende Software an Spezialverfahren wie Roboter-CT oder der sogenannten Laminographie, die beispielsweise in der immer wichtiger werdenden Batteriezellenentwicklung angewandt wird. Wir entwickeln aktuell die neuartige Quanten-Rekonstruktionstechnik (QRT), die den Rekonstruktionsschritt in der Industrie-CT revolutioniert. Mit dieser neuen Technik wird die Ausbreitung der einzelnen Röntgenphotonen (der Quanten) simuliert und anschließend invertiert. Mithilfe eines iterativen Optimierungsalgorithmus wird so eine Rekonstruktion des Objektes erstellt.
Scientific fields: 409-05 Interactive and Intelligent Systems, Image and Language Processing, Computer Graphics and Visualisation; 407-02 Measurement Systems
Principal Investigator: Dr.-Ing. Darius Rückert, Friedrich-Alexander-Universität Erlangen-Nürnberg
Target system: GPGPU cluster Alex
Electrical Engineering and Information Technology
Abstract
The project aims to investigate the potential of hyperparameter optimization in Large Language Models (LLMs) by using substantially smaller models in the optimization process. While, in general, it is not viable to transfer hyperparameters across model size, a recently introduced re-parametrization of the network, the maximal update parametrization (Yang et al., 2021), enables unchanged transfer. However, it has not yet been examined whether transferability holds if the training dataset changes, for example, if the complexity of the training data is reduced. If confirmed, it would open an avenue for a significant reduction of computational resources and energy consumption in LLM training and, in this way, increase the sustainability of LLM design and application.
Scientific field: 408 Electrical Engineering and Information Technology
Principal Investigator: Prof. Dr. Emanuël Habets, Friedrich-Alexander-Universität Erlangen-Nürnberg
Target system: GPGPU cluster Alex
Abstract
Recent text-to-speech (TTS) synthesis systems are capable of synthesizing speech of high perceptual quality. Until a few years ago, these systems were inherently mono-lingual, i.e., they only could synthesize text in a single fixed language. Enabling such systems to synthesize text in multiple languages requires modifications to the acoustic model of the TTS pipeline, i.e., the module that converts text inputs to speech features. Previous work on multi-lingual neural TTS focused on extending autoregressive models such as Tacotron 2 using language embeddings and techniques like domain-adversarial training. Later, multi-lingual extensions were also applied to parallel architectures such as FastSpeech. Recently, Lux et al. proposed using articulatory features as input to a parallel TTS system for improving multi-lingual information sharing. In this project, we want to extend the parallel TTS architecture ForwardTacotron to enable multi-lingual speech synthesis. We perform various experiments to analyze the design of our model extension.
Scientific field: 408 Electrical Engineering and Information Technology
Principal Investigator: Prof. Dr. Emanuël Habets, Friedrich-Alexander-Universität Erlangen-Nürnberg
Target system: GPGPU cluster Alex
Abstract
Effective communication is crucial in dynamic settings like teleconferences, online classes, and virtual assistance. However, background noise, along with acoustic challenges such as reverberation and echo, often reduces clarity. Our project aims to overcome reverberation issues in real-time speech communication. Utilizing deep neural networks, we focus on eliminating late reflections from speech signals while retaining early reflections, which enhance speech intelligibility.
At the heart of our research is the development of a mask-based method for speech signal dereverberation. Our goal is to develop an effective and efficient single-channel dereverberation model tailored for indoor environments, removing undesirable late reflections. We are exploring low-complexity models suitable for real-time applications and aim to extend our research to multi-channel dereverberation.
This research not only contributes to the technical domain of speech and acoustic signal processing but also promises substantial improvements in everyday communication scenarios.
Scientific field: 408 Electrical Engineering and Information Technology
Principal Investigator: Prof. Dr. Emanuël Habets, Friedrich-Alexander-Universität Erlangen-Nürnberg
Target system: GPGPU cluster Alex
Abstract
Knowing the room geometry may benefit many audio applications, including sound reproduction, audio rendering, acoustic scene analysis, and sound source localization. Room geometry inference deals with the problem of reflector localization based on a set of room impulse responses. Motivated by the increasing popularity of commercially available soundbars and smart speakers, our aim is to infer the room geometry using available room impulse responses from these types of devices. To achieve this goal, our aim is to develop data-driven models that are robust to noise and to infer the room geometry faster and more accurately compared to classical methods.
Scientific field: 408 Electrical Engineering and Information Technology
Principal Investigator: Prof. Dr. Emanuël Habets, Friedrich-Alexander-Universität Erlangen-Nürnberg
Target system: GPGPU cluster Alex
Abstract
This project aims to accelerate training and convergence of large language models (LLMs) using novel optimizers such as Sophia and Lion. We train 3-billion parameter models, focusing on efficient optimizer configurations through an ablation study. Our approach evaluates training speed and quality, aiming to minimize the required computing resources. The goal is to identify the most effective optimizer, significantly reducing computational resources and energy consumption in LLM training, thereby offering a sustainable path for AI research.
Scientific field: 408 Electrical Engineering and Information Technology
Principal Investigator: Prof. Dr. Emanuël Habets, Friedrich-Alexander-Universität Erlangen-Nürnberg
Target system: GPGPU cluster Alex & parallel computer Fritz
Abstract
In today’s fast-paced world, clear and effective communication is crucial, especially in real-time scenarios like teleconferences, online classes, and virtual assistance. However, ambient noises and other acoustic factors often hinder this communication. Recent advances in deep learning-based noise suppression have helped in addressing this to a major extent; however, for a variety of cases, the distortion caused by the processing becomes detrimental to the listening experience. Our project addresses this challenge by focusing on generative rather than discriminative learning to design real-time speech enhancement solutions. Leveraging the power of generative modeling, the approach aims to pose the problem of speech enhancement as a resynthesis task rather than simply reducing the noise(s).
The core of our research lies in investigating generative approaches to speech enhancement. The aim is to resynthesize only the input speech signal at the output, thereby eliminating the other acoustic disturbances. The scope of the research is to investigate 1) having the generative model as a post- processor to a noise reduction model and 2) having the noise reduction model perform joint noise reduction and speech resynthesis. This research advances the technical field of speech and acoustic signal processing and offers tangible benefits for everyday communication, especially in challenging scenarios.
Scientific field: 408 Electrical Engineering and Information Technology
Principal Investigator: Prof. Dr. Emanuël Habets, Friedrich-Alexander-Universität Erlangen-Nürnberg
Target system: GPGPU cluster Alex
Abstract
In today’s fast-paced world, clear and effective communication is crucial, especially in real-time scenarios like teleconferences, online classes, and virtual assistance. However, this communication is often hindered by the presence of multiple speakers and background noises, which can lead to miscommunication and frustration. Our project addresses this challenge by focusing on the extraction of a target speaker’s voice from complex audio environments in real time. Leveraging the power of deep neural networks, our approach uses an enrolment recording of the target speaker. This enrolment recording serves as a reference, enabling our system to effectively identify and isolate the target speaker’s voice from a mixture of sounds.
The core of our research lies in developing an advanced algorithm that not only distinguishes the target speaker from others but also actively suppresses background noise, resulting in a clearer and more intelligible audio output. This technology holds immense potential for improving the quality and efficiency of various real-time communication applications, from virtual meetings to voice-controlled interfaces. By providing a solution that allows for clear transmission of a specific speaker’s voice, our project aims to facilitate smoother, more effective communication in diverse and noisy environments, making it an essential tool for both professional and personal settings. This research not only advances the technical field of speech and acoustic signal processing but also offers tangible benefits for everyday communication.
Scientific field: 408 Electrical Engineering and Information Technology
Principal Investigator: Prof. Dr. Emanuël Habets, Friedrich-Alexander-Universität Erlangen-Nürnberg
Target system: GPGPU cluster Alex
Abstract
In a scenario with multiple active sound sources, noise, and reverberation, the task of extracting and separating sources is known to be challenging. Using several spatially distributed microphones, the task can be formulated as a multiple-input multiple-output problem. Our research focuses on separating speech sources in predefined spatial regions, for example, related to the seats in a car or the seats in a conference room. We employ deep neural networks for the separation process.
Scientific field: 408 Electrical Engineering and Information Technology
Principal Investigator: Prof. Dr. Emanuël Habets, Friedrich-Alexander-Universität Erlangen-Nürnberg
Target system: parallel computer Fritz
Computer Science
Abstract
Generative large language models (LLM) have reshaped the area of natural language processing over the past years, enabling new technical solutions and applications. Recently, special interest can be observed in developing smaller, efficient language models that are suitable for local or even on-device deployment on mobile devices such as laptops or mobile phones, which significantly improves privacy aspects and response time. Published open-source models have unfortunately been trained with strong focus on English only. This project aims at understanding and developing strategies to train efficient multilingual models suitable for local and on-device deployment. More precisely, the targeted model should also support German conversations and show strong reasoning capabilities. The latter feature is important in many application scenarios of LLMs and is achieved by including code-related training data in addition to text-only data. The research and developments in this project cover all aspects of an LLM’s generation life cycle. It covers data pre-processing and filtering to obtain high-quality training data, architectural design for efficient model inference, training of actual models, quantization of model parameters, and the evaluation of different model variants on established benchmark tasks.
Scientific field: 409-05 Interactive and Intelligent Systems, Image and Language Processing, Computer Graphics and Visualisation
Principal Investigator: Prof. Dr. Emanuël Habets, Friedrich-Alexander-Universität Erlangen-Nürnberg
Target system: GPGPU cluster Alex & parallel computer Fritz
Abstract
Recent studies show extreme interest in generative models, such as LLM and Diffusion models. For auto- regressive generation, Reinforcement Learning Human Feedback(RLHF) plays a vital role in the LLM calibration, which offers a promising direction to enhance the performance and adaptability of intelligent systems. At the same time, it also improves the alignment of their objectives with human values. As RLHF shows the capability to optimize transformer-based language models, the potential of RLHF to guide other transformer-based generative models is still underexplored.
In this research proposal, we want to examine the possibility of applying RLHF to MeshGPT, a leading-edge GPT-based model for 3D mesh generation. The project will leverage the synergy between the Text-to-Text Transfer Transformer (T5) for processing natural language inputs and apply RLHF for aligning these language inputs with geometric outputs. The GPT-based model will be ;ine-tuned so that the model’s outputs are aligned with human expectations. The key challenge of the project is how to make the generation more controllable by incorporating natural languages, where the application of reinforcement learning techniques play the key role, speci;ically RLHF and Decoupled Policy Optimization (DPO), to re;ine the model’s response to user feedback iteratively. The outcome will be a user-driven, adaptive tool that improves its precision and transforms how professionals create 3D models using simple descriptive language prompts.
Scientific field: 409-05 Interactive and Intelligent Systems, Image and Language Processing, Computer Graphics and Visualisation
Principal Investigator: Prof. Dr. Matthias Schubert, Ludwig-Maximilians-Universität München
Target system: GPGPU cluster Alex
Abstract
This project outlines a pioneering research endeavor aimed at integrating visual and linguistic comprehension within intelligent systems to enable continuous reasoning. We emphasize the pivotal role of understanding the visual environment in the pursuit of Artificial General Intelligence (AGI). Our investigation reveals the reliance of contemporary systems on natural language processing for reasoning, underscoring the need to enhance these capabilities with robust visual reasoning. We propose recalibrating processing pipelines across visual and linguistic domains to redefine the frontiers of intelligent systems. Our interdisciplinary approach spans object detection, real-time video processing, and their integration with language models to cultivate intelligent systems adept at navigating and engaging with the world in real-time. Through this pursuit, we anticipate transformative impacts on various domains, advancing the frontiers of multimodal intelligence.
Scientific field: 409-05 Interactive and Intelligent Systems, Image and Language Processing, Computer Graphics and Visualisation
Principal Investigator: Prof. Dr. Thomas Seidl, Ludwig-Maximilians-Universität München
Target system: GPGPU cluster Alex
Abstract
In light of recent forward leaps in the capability of multimodal AI, the concern for the reliability of these models has steadily grown. We aim to advance the idea of selective prediction to improve and develop new, especially multimodal, models. In particular, one objective is to benchmark existing models and improve their capability by investigating novel approaches that improve the inherent trade-off between coverage (portion of questions answered) and risk (error on that portion). We will evaluate our novel approaches on a set of diverse multimodal tasks to understand their generalizability.
Scientific field: 409-05 Interactive and Intelligent Systems, Image and Language Processing, Computer Graphics and Visualisation
Principal Investigator: Prof. Dr. Marcus Rohrbach, Technical University of Darmstadt
Target system: GPGPU cluster Alex
Abstract
Language models rely on pre-trained tokenizers, which limit their ability to comprehend languages outside their initial training scope. To address this, tokenizers rely on byte-fallback or byte-tokenization, where input text is broken down to its bytes. This, however, is very inefficient and awkward. Particularly for non-Latin scripts, which often consist of multi-byte characters, this
method leads to a decomposition into several bytes (i.e., sequence lengths increase) that may appear entirely unrelated, complicating the model’s ability to accurately interpret and generate meaningful language constructs. We propose to rely on bitmaps of GNU Unifont that encodes almost the entire Unicode set into 16 by 16 pixels as an input to train networks that equitably model any input language to address the above mentioned shortcomings. A core advantage of our approach is that we do not store and update the input and output embeddings of our language model.
We want to user the NHR GPU resources to comprehensively train and benchmark Byte, Myte and Unifont language models. To that end, we will train medium-size language models (350M to 1BN
parameters) on textual data from 100+ languages. We will then evaluate our models both intrinsically, that is the ability to model language on with-held text, as well as extrinsically, in various
downstream tasks like sequence classification or question answering, in a highly multilingual and cross-lingual context. Training of c. 3 variants of multilingual language models (Byte-Tokenization, Myte-Tokenization, and our approach) from scratch is estimated to take 4 days per model on a 8 GPU machine (or 768 GPU hours per model). Subsequent downstream evaluations should total 400 GPU hours (one run requires c. 10 GPU hours, 40 runs seem like a realistic estimate).
Scientific field: 409-08 Massively Parallel and Data-Intensive Systems
Principal Investigator: Prof. Dr. Goran Glavaš, Julius-Maximilians-Universität Würzburg
Target system: GPGPU cluster Alex
Abstract
Future artificial intelligence (AI) assistive robots will need to autonomously accomplish household tasks and naturally interact with humans. Research in AI and robotics has accomplished significant results in mobile robot navigation and in fixed base manipulation, but is still limited to specific installations and specialized to single tasks. The embodiment hypothesis suggests that intelligent behavior may be acquired by the continual purposeful interaction of an agent with an environment and the induced sensorimotor experience. Motivated by this paradigm, we propose to advance beyond the current ad-hoc solutions by conducting research in our project, that is dedicated to developing methods for robot learning of mobile manipulation for intelligent assistance.
Scientific field: 409-05 Interactive and Intelligent Systems, Image and Language Processing, Computer Graphics and Visualisation
Principal Investigator: Prof.‘in Georgia Chalvatzaki, Ph.D., Technical University of Darmstadt
Target system: GPGPU cluster Alex
Abstract
Die Nephropathologie ist essentiell für die Diagnose von Nierenkrankheiten und ein äußerst wichtiges Tool für experimentelle, präklinische Nierenstudien. Fortschritte in der digitalen Pathologie, d.h. die Digitalisierung von histologischen Schnitten, und die Anwendung von künstlicher Intelligenz, insbesondere Deep Learning, haben hier neue Forschungsperspektiven eröffnet mit dem Potenzial die Gewebediagnostik in eine quantitative “computergestützte” Pathologie zu transformieren. Unser Ziel ist die Entwicklung und Anwendung von Deep Learning sowohl in der klinischen als auch in der experimentellen Nephropathologie. Wir haben eine hochmoderne virtuelle Pathologieplattform für Hochdurchsatz von Hellfeld-, Fluoreszenz- und multispektraler Bildgebung sowie eine zentralisierte, Server-basierte Speicherung und Analyse digitalisierter histologischer Präparate eingerichtet. Diese Plattform wird durch unsere sich in hohem Maße ergänzenden, interdisziplinären Fachkenntnisse unterstützt und die Entwicklung der digitalen Pathologie erleichtern. Unser Ziel ist es, Deep Learning Ansätze zu entwickeln, um verschiedene Nierenkompartimente automatisiert zu segmentieren und zu klassifizieren, was eine Unterscheidung verschiedener Tierarten, verschiedener Krankheitsmodelle und menschlicher Nierenerkrankungen ermöglicht. Wir werden uns dabei auch verschiedenen Herausforderungen stellen, die in der digitalen Pathologie vorherrschen, z.B. des Mangels manuell annotierter Daten. Darüber hinaus werden wir umfassende Analysen in Form von Pathomics durchführen, um unser Verständnis der Histopathologie der Niere zu vertiefen. Dies erfolgt durch eine groß angelegte Extraktion quantitativer Bildmerkmale, die bisher unerkannte morphologische Merkmale in jedem Nierenkompartiment für verschiedene Bereiche erkennen könnten. Als Proof-of-Concept werden wir unsere trainierten Netze nutzen, um Pathomics Daten aus unseren Experimente zu generieren, in denen wir die Rolle der Desmosomen als Biomarker für Nierenerkrankungen untersuchen. Darüber hinaus werden wir unsere Expertise in der Nephropathologie und Bildanalyse für das gesamte Konsortium zur Verfügung stellen, wodurch sich die Vergleichbarkeit der im Konsortium generierten Daten stark verbessert und gleichzeitig die Datensätze für unsere Deep Learning Ansätze wesentlich erweitert werden. Zusammenfassend sind wir bestrebt, Ansätze für Deep Learning und Pathomics zu entwickeln und anzuwenden, um den Übergang zu einen innovativen quantitativen Pathologiediagnostik bzw. bis hin zu einer personalisierten präzisions Pathologie zu erleichtern.
Scientific field: 409-05 Interactive and Intelligent Systems, Image and Language Processing, Computer Graphics and Visualisation
Principal Investigator: Prof. Dr.-Ing. Dorit Merhof, University of Regensburg
Target system: GPGPU cluster Alex
Abstract
This project explores optimizing pretrained Large Language Models (LLMs) on medium-sized GPU clusters, focusing on energy efficiency and domain-specific performance. Key phases include: analyzing LLMs’ power consumption to promote sustainable computing; developing benchmarks to assess LLMs in specific domains; training baseline models on GPU clusters balancing performance with resource use; enhancing dataset quality using LLMs; and improving information retrieval through LLM-supervised fine-tuning. The goal is to achieve energy-efficient, domain-tailored LLM applications.
Scientific field: 409-05 Interactive and Intelligent Systems, Image and Language Processing, Computer Graphics and Visualisation
Principal Investigator: Prof. Dr.-Ing. habil. Andreas Maier, Friedrich-Alexander-Universität Erlangen-Nürnberg
Target system: GPGPU cluster Alex
Abstract
Abstract reasoning is a fundamental aspect of human intelligence. It allows us to solve new, unfamiliar problems without the need for prior knowledge (Gilead et al. 2014). Developing machines that can reason this way (an ability closely related to the concept of fluid intelligence (Cattel 1963)), has been a long-standing goal in the field of artificial intelligence (Barrett et al. 2018). However, most modern AI systems (including large language models) do still lack general abstract reasoning capabilities (Marcus 2018, Gendron et al. 2023). In 2019, François Chollet introduced the Abstraction and Reasoning Corpus (ARC), a collection of visual puzzles designed to evaluate human-like general fluid intelligence in AI systems (Chollet 2019). While humans can robustly solve around 80% of ARC tasks, current algorithms markedly underperform with a maximum success rate of 31% (Johnson et al. 2021). This disparity underscores the necessity for continued development in the field of automated abstract reasoning. Various challenges have been created to motivate developers to solve ARC. This project intends to tackle the ARC benchmark by leveraging insights from recent findings on systematic generalization. For this, we will exploit the compositional nature of ARC tasks. The aim is to develop an algorithm capable of systematic generalization, extrapolating from specific examples to novel test scenarios. This approach aims not only to enhance the performance of AI systems on the ARC benchmark, but also to contribute to the broader understanding of abstract reasoning in artificial intelligence.
Scientific field: 409-05 Interactive and Intelligent Systems, Image and Language Processing, Computer Graphics and Visualisation
Principal Investigator: Prof. Dr. Barbara Plank, Ludwig-Maximilians-Universität München
Target system: GPGPU cluster Alex
Abstract
In the realm of multimodal AI, comprehending lengthy videos presents a formidable challenge, given the rising demand for automated processing of such content. Videos, rich in both text and images posing difficulties for existing AI models like the Transformer. This highlights the necessity for specialized multimodal foundation models tailored to long video understanding. Our research employs visual encoders and pretrained large language model, such as LLaMA, to address this challenge.
Scientific field: 409-05 Interactive and Intelligent Systems, Image and Language Processing, Computer Graphics and Visualisation
Principal Investigator: Prof. Dr. Volker Tresp, Ludwig-Maximilians-Universität München
Target system: GPGPU cluster Alex
Abstract
Diffusion imaging (DI) has rapidly become one of the most important non-invasive tools for clinical brain research in recent years. However, long measurement times due to the required number of gradient directions recorded lead to a rare use of DI in clinical practice. To overcome this problem, recent methods have shown the power of machine learning and in particular deep learning (DL), which is capable of very accurately describing and reconstructing the underlying complex functions of tissue while requiring only a few gradient directions. The necessary measurement time can be greatly reduced in this way. However, for optimal applicability of the DL in clinical DI, four major hurdles were identified, which should be overcome within the framework of this project. The biggest hurdle is the large variance between data from different MRI systems. First, existing methods for aligning different systems are compared and then an optimal method for harmonizing the MRI signals is developed. Another problem is that ground truth (GT) data is difficult to obtain, which makes training DL methods more difficult. To solve this problem, a framework is developed that determines important diffusion characteristics and statistics and uses this information to synthesize individual diffusion data or a complete data set. The data created in this way and their corresponding GT can then be used during the training of the DL models. Furthermore, as part of this project, the complex signals that are rarely used in regular methods and which arise when an MRI sequence is acquired are used with the help of novel DL methods The reconstruction was included because previous studies have shown that the complex additional information contains important clues about the underlying tissue. Finally, the focus is on the angular dependence of the diffusion signals. Previous DL methods are currently not able to incorporate this spherical information into the processing. Using new methods within the DL, this neighborhood information can be included both within a voxel and between voxels to ensure optimal reconstruction. To evaluate all the methods described, MRI data will be acquired at various locations during the first half of the project, which will provide the required data Phase data, a high number of gradient directions, and high resolution. The aim of the results obtained through this project and the methods developed is to be able to significantly reduce the measurement times for diffusion sequences in clinical studies while maintaining the same accuracy.
Scientific field: 409-05 Interactive and Intelligent Systems, Image and Language Processing, Computer Graphics and Visualisation
Principal Investigator: Prof. Dr.-Ing. Dorit Merhof, University of Regensburg
Target system: GPGPU cluster Alex
Abstract
Goal of the project is to enhance neural image-based rendering approaches for the online generation of novel views while the scene is still being captured. To this end, we will develop and integrate a novel differential rendering method that fulfills real-time requirements and thus, will be highly beneficial for the visualization quality by refining depth maps and camera poses.
By comparing multiple rendered frames with actual sensor data or neural depth estimations, we aim to refine depth information in real-time, resulting in more accurate and precise depth maps. These improved depth maps cannot not only benefit image-based rendering, leading to more realistic and visually appealing images, but also other down-stream tasks within the context of 3D reconstruction.
We plan to leverage these enhanced depth maps as an initialization step for scene-global 3D geometry extraction. The anticipated outcomes of this project include high-quality depth maps, improved image- based rendering, and efficient 3D reconstruction, thus being useful for various applications.
Scientific field: 409-05 Interactive and Intelligent Systems, Image and Language Processing, Computer Graphics and Visualisation
Principal Investigator: Prof. Dr.-Ing. Marc Stamminger, Friedrich-Alexander-Universität Erlangen-Nürnberg
Target system: GPGPU cluster Alex
Abstract
Multimodal models have demonstrated remarkable capabilities in various natural language understanding and computer vision tasks. Previous approaches typically employed separate vision encoders to extract image features and text encoders for multimodal understanding and reasoning. In this project, we propose a novel approach that utilizes a decoder-only architecture for direct alignment of pixel-level images and text in a generative framework. Unlike previous works that relied on vision encoders, our method streamlines the process, improving efficiency and effectiveness in multimodal tasks, without incorporating additional large vision encoders.
Our research focuses on developing a state-of-the-art multimodal foundation model by leveraging a decoder-only architecture. This approach allows us to bridge the gap between textual and visual information more directly, eliminating the need for separate vision encoders. By aligning text and images at the pixel level, we aim to enhance the model’s ability to understand and generate multimodal content.
The proposed model will be pretrained on a large-scale multimodal dataset, capturing rich and diverse textual and visual information. We will investigate novel techniques for aligning image and text representations during pretraining, enabling the model to learn meaningful cross-modal relationships. This will result in a powerful multimodal foundation that can be fine-tuned for various downstream tasks.
Our project’s primary goal is to demonstrate the superiority of our decoder-only architecture in handling multimodal data. We will conduct extensive experiments and evaluations on a range of tasks, such as image captioning, visual question answering, and multimodal sentiment analysis, to showcase the model’s improved performance over existing methods.
Scientific field: 409-05 Interactive and Intelligent Systems, Image and Language Processing, Computer Graphics and Visualisation
Principal Investigator: Prof. Dr. Volker Tresp, Ludwig-Maximilians-Universität München
Target system: GPGPU cluster Alex
Abstract
Developing autonomous driving and advanced driver-assistance systems is getting more important for the automotive industry. In addition to developing these systems, it is also necessary to guarantee their safety, which may require covering billions of driven kilometers. Virtual testing environments can pose solutions if they reach the necessary realism. This project tries to achieve this by training neural networks on real sensor data and transferring the trained systems to the virtual driving simulation.
Scientific field: 409-05 Interactive and Intelligent Systems, Image and Language Processing, Computer Graphics and Visualisation
Principal Investigator: Prof. Dr.-Ing. Marc Stamminger, Friedrich-Alexander-Universität Erlangen-Nürnberg
Target system: GPGPU cluster Alex
Abstract
We aim to transfer concepts from inverse rendering to radar simulation and reconstruction, thereby bridging the gap between recent research from the fields of computer graphics and millimeter-wave multiple-input-multiple-output (MIMO) imaging radar. We leverage gradient descent based optimization to match the scene parameters of a radar simulation to observations of a real radar and derive shape or material properties to improve simulation and reconstruction fidelity. Due to the computationally extensive requirements of this technique, it is well suited for acceleration on the NHR.
Scientific field: 409-05 Interactive and Intelligent Systems, Image and Language Processing, Computer Graphics and Visualisation
Principal Investigator: Prof. Dr.-Ing. Marc Stamminger, Friedrich-Alexander-Universität Erlangen-Nürnberg
Target system: GPGPU cluster Alex
Abstract
Neural radiance fields promise 3D reconstructions with higher photorealism. However, training is memory intensive. Moreover, rendering the resulting models in real-time is challenging. Starting from an initial available implementation, we aim to improve algorithms and hyper-parameters to increase achievable quality. At the same time, we want to optimize the representation format of the 3D information to allow for efficient real-time rendering of the obtained model.
Scientific field: 409-05 Interactive and Intelligent Systems, Image and Language Processing, Computer Graphics and Visualisation
Principal Investigator: Dr.-Ing. Joachim Keinert, Friedrich-Alexander-Universität Erlangen-Nürnberg
Target system: GPGPU cluster Alex
Abstract
Speech enhancement seeks to recover clean speech from audio recordings affected by different sources of distortions, such as environmental noise. Diffusion models, a subset of generative models, learn a prior distribution over clean speech data, capturing inherent spectral and temporal structures. This prior knowledge enables enhancing degraded speech into natural clean speech estimates. However, enhancing with diffusion models during inference is computationally expensive, therefore we aim at reducing the computational costs of diffusion models by reducing the architecture footprint and modifying the diffusion process itself.
Scientific field: 409 Computer Science
Principal Investigator: Prof. Dr.-Ing. Timo Gerkmann, Universität Hamburg
Target system: GPGPU cluster Alex
Abstract
The goal of this WP is to reconstruct the lost scents and odours of the past through their visual traces in art. By means of selected objects, motifs and iconographies, we will first analyse what meaning and design smell had in 17th–20th century works of art. At the same time, objects strongly defined by smell are to be automatically recognized via computer vision in large-scale European digital collections and the appearance and patterns of their use analysed. We thus combine a classical approach based on corpus formation and qualitative interpretation with a data-driven approach based on ‘distant viewing’. By doing so, we explore and develop new uses of mixed methods and computing in the fields of digital art history and computer vision. Finally, we build an interactive notebook-based demonstrator to show how images can be retrieved by means of their olfactory references.
Scientific field: 409-05 Interactive and Intelligent Systems, Image and Language Processing, Computer Graphics and Visualisation
Principal Investigator: Dr.-Ing. Vincent Christlein, Friedrich-Alexander-Universität Erlangen-Nürnberg
Target system: GPGPU cluster Alex
Abstract
We are planning to develop and evaluate approaches for fine-tuning large language models with the goal of combining different learning objectives i.e. preference learning and language modeling.
Scientific field: 409-05 Interactive and Intelligent Systems, Image and Language Processing, Computer Graphics and Visualisation
Principal Investigator: Dr. René Jäkel, Technische Universität Dresden
Target system: GPGPU cluster Alex
Abstract
This project will extend the current limits of Deep RL to improve its practicality in realistic high- dimensional problems. Our contributions will be a step forward in unleashing the full potential of Deep RL by making it more efficient and easier to use in real-world environments. We will achieve our goal by addressing the following fundamental problems of Deep RL: Computational and data requirements, human supervision and tuning, and task overfitting. Although agents trained with Deep RL perform excellently, they have a strong tendency to overfit the available data.
In this project, we will show that these seemingly different problems have similarities and common causes, and we will address them by introducing a new unifying view that will guide our research for more efficient and practical Deep RL methods that can be used in everyday applications.
We will demonstrate the critical importance of our methodological advances in learning locomotion skills in a leg-operated wheelchair. In this application, the wheelchair needs to move robustly and safely at different speeds, avoid obstacles and adapt to different terrains. In addition, the gait pattern should be adapted to the needs and preferences of the human user. We will demonstrate the importance of our algorithmic solutions in a realistic problem where multiple skills are required, such as navigating to a destination, walking up stairs and avoiding obstacles along the way.
The TriFORCE team is made up of outstanding scientists, an advisory board with renowned researchers, female leaders from academia and partners from industry.
Scientific field: 409-05 Interactive and Intelligent Systems, Image and Language Processing, Computer Graphics and Visualisation
Principal Investigator: Prof. Dr. Carlo D’Eramo, Julius-Maximilians-Universität Würzburg
Target system: GPGPU cluster Alex
Abstract
A major obstacle to the widespread use of ultrahigh-field magnetic resonance imaging (UHF-MRT) is the complex distribution of electromagnetic waves in the patient’s thorax, which has a very negative impact on image quality and also carries the risk of undesirable overheating of the tissue. Conventional computational methods for calculating the 3D field distribution require several days and thus cannot be used on patients. Therefore, a new approach is developed in the present research project: Physics Informed Neural Networks (PINN) will be used to train neural networks based on Maxwell’s equations, which will allow to calculate the energy deposition in the body within a few minutes. Together with the German Center for Heart Failure, PINNs, which have so far only been described for two-dimensional applications, will be further developed for the three-dimensional case.
Scientific field: 409 Computer Science
Principal Investigator: Dr.-Ing. Anna Krause, Julius-Maximilians-Universität Würzburg
Target system: GPGPU cluster Alex
Project Report (09/2024)
A major obstacle to the widespread use of ultrahigh-field magnetic resonance imaging (UHF-MRT) is the complex distribution of electromagnetic waves in the patient’s thorax, which has a very negative impact on image quality and also carries the risk of undesirable overheating of the tissue. Conventional computational methods for calculating the 3D field distribution require several days and thus cannot be used on patients. Therefore, a new approach is developed in the present research project: Physics Informed Neural Networks (PINN) will be used to train neural networks based on Maxwell’s equations, which will allow to calculate the energy deposition in the body within a few minutes. Together with the German Center for Heart Failure, PINNs, which have so far only been described for two-dimensional applications, will be further developed for the three-dimensional case.
Abstract
This project aims to identify existing shortcomings in the German NLP/LLM landscape, by training a large German language model from scratch in a controlled setting, finetuning it on several different domains, and finally evaluating its performance on domainspecific, but also broad and general downstream tasks. We will make the models, code, results and findings public and accessible for the German NLP community.
Scientific field: 409 Computer Science
Principal Investigator: Prof. Dr. Andreas Hotho, Julius-Maximilians-Universität Würzburg
Target system: GPGPU cluster Alex
Project Report (03/2024)
The main motivation of this test project was to create a benchmark for German language models, as German is a language that has been relatively underexplored/-evaluated in NLP. Quite a few German resources and multi-lingual models do exist, but they traditionally have neither been explicitly trained on German texts, nor been evaluated on German tasks. Hence we assemble a broad Natural Language Understanding benchmark suite consisting of 29 tasks for German and consequently evaluate 10 German-capable models on them in order to create a better understanding of the current state of German LLMs. In our comprehensive evaluation we find that encoder models are a good choice for most tasks, but also that the largest encoder model does not necessarily perform best for all tasks. We make our benchmark suite and a leaderboard publicly available and encourage the community to contribute new tasks and evaluate more models on it.
Publications
J. Pfister and A. Hotho: SuperGLEBer: German Language Understanding Evaluation Benchmark. Proceedings of the 2024 Conference of the North American Chapter of the Association for Computational Linguistics: Human Language Technologies (Volume 1: Long Papers), 2024. DOI: 10.18653/v1/2024.naacl-long.438.
Abstract
Sub-project A01 of EmpkinS explores a novel, multimodal sensor concept for high-precision, non-contact detection of the envelope of the human body and the velocity vector of each point on this envelope. For this purpose, a micro-doppler aperture synthesis radar is combined with an optical depth camera and the strengths of the sensors are combined. The body envelope and its movements are detected with outstanding precision and at a high measuring rate. With these properties, the system is a central basis for the research programme of the CRC EmpkinS. To be able to detect a human body and capture its pose in the radar and the depth camera, a large variety computer vision algorithms is required, ranging from simple Region-of-Interest detection of the human in an image, to segmentation, 3D reconstruction, and human pose estimation algorithms. In terms of quality, Deep Learning approaches have proven outstanding performance with respect to traditional optimization-based approaches. Therefore, this project aims at leveraging Deep Learning approaches, which operate on the previously-mentioned sensor data such as RGB ((depth-)camera), RGB-D (depth-camera) and radar data.
Scientific field: 409 Computer Science
Principal Investigator: Prof. Dr.-Ing. Marc Stamminger, Friedrich-Alexander-Universität Erlangen-Nürnberg
Target system: GPGPU cluster Alex
Abstract
The digiOnko project (https://www.digionko-bayern.de/) aims at improving the screening, early detection, diagnosis, treatment and aftercare for women with breast cancer. One aspect of this project is the use of machine learning methods in digital pathology. Deep learning methods will be used to analyze digital Whole Slide Images (WSI), in particular to automatically calculate scores and improve treatment decisions.
Scientific field: 409-05 Materials Engineering
Principal Investigator: Prof. Dr.-Ing. habil. Andreas Maier, Friedrich-Alexander-Universität Erlangen-Nürnberg
Target system: GPGPU cluster Alex
Project Report (09/2024)
This sub-project, focuses on research of the HER2 breast cancer subtype using deep learning methods. Multiple works were already performed with the aid of NHR resources. A method to generate synthetic data using un-annotated data was developed, reducing the need for human annotations.
A work to analyze and adapt generative AI for the task of medical image segmentation was performed, resulting in better use of such methods for the task of medical image segmentation.
Finally, a fully automatic segmentation pipeline for HER2 tissue was developed, allowing to automatically score patients and also establishing opportunities for further research on HER2 tissue distributions.
Abstract
Today, it is possible to capture the 3D-Geometry of real-world objects and rooms in high quality by using modern sensors and algorithms for 3D reconstruction. These virtual models open the door for many applications, e.g., floor plans can be generated, and distances measured virtually. However, for virtual walkthroughs, the resulting models are usually not sufficient yet to achieve high-quality renderings from arbitrary views. With 360°-photographs (panoramas) it possible that a user explores a scene in photo- realistic quality, but only by looking around, and not moving freely. Image-based rendering is a well- researched approach to lift this restriction: photographs of the scene are reprojected to novel views, based on a coarse 3D model of the world. Yet, image-based rendering often suffers from artifacts, and most often does not deliver photo-realistic quality.
In the project we research novel approaches based on deep neural networks that improve the quality of the images generated by image-based rendering and that can remove or reduce typical artifacts. Focus of the project is in the first phase the generation of panoramas from a set of nearby inside-out take images that exploit such techniques to generate artifact-free panoramas from few input images. Later on, we will focus on approaches that allow the free movement of a user in real-time, making it possible to walk through a scene virtually.
The work in the project is centered around data obtained by the 3D-indoor-scanning systems of the industrial partner NavVis (Munich), who can deliver high-quality 3D point clouds and registered input images. We will thus focus on novel neural rendering techniques that as point clouds as proxy geometry, on the generation of panoramic images, and the research of possibilities to achieve free-viewpoint video, based on this data, in real-time.
Scientific field: 409-05 Interactive and Intelligent Systems, Image and Language Processing, Computer Graphics and Visualisation
Principal Investigator: Prof. Dr.-Ing. Marc Stamminger, Friedrich-Alexander-Universität Erlangen-Nürnberg
Target system: GPGPU cluster Alex
Abstract
How do we best represent objects and their variations for inverse rendering? Can a combination of classical and novel techniques increase photorealism whilst retaining a low dimensional and interpretable representation? And given such object models: How do we efficiently infer the scene graph from visual input based on object-agnostic and object-specific processing? And ultimately can we explain the extreme generalization capabilities of the human visual system with an inverse rendering account?
Scientific field: 409-05 Interactive and Intelligent Systems, Image and Language Processing, Computer Graphics and Visualisation
Principal Investigator: Prof. Dr. Bernhard Egger, Friedrich-Alexander-Universität Erlangen-Nürnberg
Target system: GPGPU cluster Alex
Project Report (07/2024)
We reached our main goals according to the BMBF proposal and made some corrections to align the project with the current developments in the research community. In particular diffusion based methods for 3D reconstruction became very popular and we include those in our approaches.
One result is a publication “Generalizable 3D Scene Reconstruction via Divide and Conquer from a Single View”. This particularly covers scaling towards the scene, multiple objects and also the inclusion of depth estimation as proposed in the project. We focused on developing a hybrid reconstruction model that combines the strengths of explicit 3D representations with the capabilities of implicit neural representations.
Furthermore, we aimed to merging of Gaussian Process Morphable Models (GPMMs) and implicit models. First, a suitable framework was sought to implement this; it turned out that Neural Kernel Fields (NKFs) are particularly well suited for this. NKFs are methodologically similar to Gaussian Process Regression, and therefore already have a probabilistic interpretation. NKFs use the so-called Neural Spline (NS) kernel; this can be augmented relatively easily by GPMMs. This allows implicit and explicit models to be combined. However, it turned out that NKFs still have problems representing detailed surfaces, such as faces. For this reason, a new kernel was and is being worked on that should represent these details better. The kernel is based on a spectral representation, whereby the Fourier coefficients are learned. This kernel, in comparison to NS, is adaptive (has parameters); its spectrum can be manipulated in a targeted manner. This makes it particularly attractive for very noisy data.
Additionally t was originally proposed that we will use extreme stimuli such as Mooney images to study feedforward processing because a model of the human brain or human in the loop is required. We adapted our hypothesis for our behavioral experiment and instead tried to determine whether our brain reconstructs faces from impaired stimuli using the same mechanism as the statistical face model. Our goal is to design more behavioral experiments that can further extrapolate the behavioral information.
Publications
A. Dogaru, M. Özer, and B. Egger: Generalizable 3D Scene Reconstruction via Divide and Conquer from a Single View. arXiv, 2024. DOI: 10.48550/arXiv.2404.03421.
M. Özer, M. Weiherer, M. Hundhausen, and B. Egger: Exploring Multi-modal Neural Scene Representations With Applications on Thermal Imaging. arXiv, 2024. DOI: 10.48550/arXiv.2403.11865.
M. Weiherer, F. Klein, and B. Egger: Approximating Intersections and Differences Between Linear Statistical Shape Models Using Markov Chain Monte Carlo. IEEE/CVF Winter Conference on Applications of Computer Vision (WACV), 2024. DOI: 10.1109/WACV57701.2024.00624.
M. Choithwani, S. Almeida, and B. Egger: PoseBias: On Dataset Bias and Task Difficulty—Is there an Optimal Camera Position for Facial Image Analysis?. IEEE/CVF International Conference on Computer Vision Workshops (ICCVW), 2023. DOI: 10.1109/ICCVW60793.2023.00334.
Abstract
The IDP “Measuring and Modelling Mountain glaciers and ice caps in a Changing ClimAte (M³OCCA)” aims to combine cutting edge technologies with climate research. We will develop future technologies and transfer knowledge from other disciplines into climate and glacier research. Within the doctoral project “Machine Learning on Radargrams”, we aim at using and modifying machine learning techniques from medical imaging as well as natural language processing and apply those to glaciological radargrams to extract information on ice thickness and internal structures of ice bodies.
Scientific field: 409-05 Interactive and Intelligent Systems, Image and Language Processing, Computer Graphics and Visualisation
Principal Investigator: Dr.-Ing. Vincent Christlein, Friedrich-Alexander-Universität Erlangen-Nürnberg
Target system: GPGPU cluster Alex
Project Report (10/2023)
So far, several cutting-edge machine learning models have been adapted to radar imagery and have been shown to exceed prior works. A comparison study is being performed to identify the best working approach. Moreover, a model that accounts for uncertainty is being developed, and learning strategies that do need fewer human annotations are considered.
Publications
F. Wu, N. Gourmelon, T. Seehaus, J. Zhang, M. Braun, A. Maier, and V. Christlein: AMD-HookNet for Glacier Front Segmentation. IEEE Transactions on Geoscience and Remote Sensing 61, 2023. DOI: 10.1109/TGRS.2023.3245419.
F. Wu, N. Gourmelon, T. Seehaus, J. Zhang, M. Braun, A. Maier, and V. Christlein: Contextual HookFormer for Glacier Calving Front Segmentation. IEEE Transactions on Geoscience and Remote Sensing 62, 2024. DOI: 10.1109/TGRS.2024.3368215.
O. Herrmann, N. Gourmelon, T. Seehaus, A. Maier, J. Fürst, M. Braun, and V. Christlein: Out-of-the-box Calving Front Detection Method Using Deep Learning. The Cryosphere 17(11), 2023. DOI: 10.5194/tc-17-4957-2023.
Conferences
N. Gourmelon, J. Klink, T. Seehaus, M. Braun, A. Maier, and V. Christlein: Conditional Random Fields for Improving Deep Learning-based Glacier Calving Front Delineations. International Geoscience and Remote Sensing Symposium 2023 (Pasadena, California, July 16–21, 2023). DOI: 10.1109/IGARSS52108.2023.10282915.
N. Gourmelon, T. Seehaus, M. Braun, A. Maier, and V. Christlein: Comparability of Deep Learning Techniques for Calving Front Segmentation in SAR Imagery. European Geoscience Union General Assembly 2023 (Vienna, April 23–28, 2023). DOI: 10.5194/egusphere-egu23-5069.
Construction Engineering and Architecture
Abstract
For many centuries, scientific discovery relied on performing experiments and the subsequent deduction of new theoretical models. The advent of powerful computers, coupled with new and ever more efficient numerical algorithms, makes it possible to simulate complex systems with increasing realism, and to automatize even model discovery using artificial intelligence (AI) technologies. Computational Fluid DynFor many centuries, scientific discovery relied on performing experiments and the subsequent deduction of new theoretical models. The advent of powerful computers, coupled with new and ever more efficient numerical algorithms, makes it possible to simulate complex systems with increasing realism, and to automatize even model discovery using AI technologies. Computational Fluid Dynamics (CFD) is one of the most prominent areas that clearly requires, and even motivate exascale computing to be part of the engineering and academic workflows. Given the physical scaling and the availability of highly efficient simulation codes, CFD has the potential of reaching exascale performance, as one of the few application areas. This center will implement exascale ready workflows for addressing relevant challenges for future exascale systems, including those procured by EuroHPC. The significant improvement in energy efficiency will be facilitated through efficient exploitation of accelerated hardware architectures (GPUs) and novel adaptive mixed-precision calculations. Emphasis is furthermore given to new or improved algorithms that are needed to exploit upcoming exascale architectures. The efforts of the center are driven by a collection of five different lighthouse cases of physical and engineering interest, ranging from aeronautical to atmospheric flows, with the goal of reaching TRL 4 and even 5 for selected cases. All development is done in five European HPC codes which span the entire spectrum of CFD applications, including compressible, incompressible and multiphase flows.
Scientific field: 410-06 Geotechnics, Hydraulic Engineering
Principal Investigator: Prof. Dr.-Ing. Harald Köstler, Friedrich-Alexander-Universität Erlangen-Nürnberg
Target system: GPGPU cluster Alex
Completed NHR compute time projects on Alex and Fritz at NHR@FAU
Humanities and Social Sciences
Humanities
Abstract
The design of proteins with tailored functions will tackle many societal challenges. Traditionally, the protein design process relied on searching the global minima of multidimensional energy functions, a process that required significant computational times for each run. Recent advances in NLP have produced protein language models capable of generating fit protein sequences within seconds. However, these current models lack control over the design process, thus preventing user-defined design. The proposal described here will train a joint model, ProtCTRL, to capture the sequence-function relationships. ProtCTRL will be capable of generating sequences upon a user prompt, ultimately enabling tailored protein design. The model will be publicly released.
Scientific fields: 201-07 Bioinformatics and Theoretical Biology; 104-04 Applied Linguistics, Experimental Linguistics, Computational Linguistics
Principal Investigator: Dr. Noelia Ferruz, Universität Bayreuth
Target system: GPGPU cluster Alex
Project Report (06/2024)
The design of proteins with tailored functions could have the potential of transforming biomedicine and a myriad of industrial processes but it has proven remarkably challenging. The advent of artificial intelligence (AI) methods capable of learning patterns from sequence-data alone in an unsupervised fashion has, however, revolutionized the field in the last two years. Protein language models (pLMs) are capable of generating sequences that resemble the properties and functions of natural proteins while being completely novel.
In this project, we have pioneered the training of pLMs and experimentally validated their performance. First, we trained ProtGPT2, capable of generating sequences in unexplored sequences of the protein space. Then, we trained ZymCTRL, a conditional model capable of generating artificial enzyme sequences for user-defined catalytic reactions. We specifically generated carbonic anhydrases and lactate dehydrogenases and showed that they are well-folded and present activities in line with the natural counterparts, despite being completely novel. Lastly, we have trained REXzyme, a conditional model that allows the design of enzyme sequences for user-defined chemical reactions, even those that are new-to-Nature. We are currently evaluating experimentally its performance. The follow-up of this project will focus on the training of a general-purpose model for all protein design tasks.
This project is still ungoing under the name: ATHENA.
Publications
N. Ferruz and B. Höcker: Controllable protein design with language models. Nature Machine Intelligence 4, 2022. DOI: 10.1038/s42256-022-00499-z.
N. Ferruz, S. Schmidt, and B. Höcker: ProtGPT2 is a deep unsupervised language model for protein design. Nat Commun 13, 2022. DOI: 10.1038/s41467-022-32007-7.
Abstract
This NHR project is tied to an existing DFG/AHRC-funded joint project by FAU Erlangen-Nürnberg and the University of Oxford studying the mechanisms used for disinformation, in particular viewpoint manipulation, by Russian state-sponsored media. The purpose of this sub-project is to create a dataset fully annotated with pose information of the English-language programs found on the now-banned YouTube presence of RT (Russia Today) both for the purpose of our own analysis and to create an annotated open dataset.
Scientific field: 104 Linguistics
Principal Investigator: Prof. Dr. Peter Uhrig, Friedrich-Alexander-Universität Erlangen-Nürnberg
Target systems: parallel computer Fritz & GPGPU cluster Alex
Project Report (04/2024)
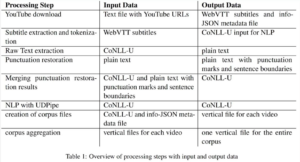
We have produced an automatically-annotated dataset for the collaborative project “World Futures: Multimodal Viewpoint Construction by Russian International Media”, which is funded by DFG and AHRC and is run jointly by the Universities of Oxford and Erlangen-Nürnberg. The raw data is Russian propaganda mainly taken from RT (formerly Russia Today) via YouTube, which was downloaded before it was banned in early 2022, including the subtitles generated automatically by YouTube, which are reasonably good transcripts of the audio channel.
We developed a pipeline to download and process the videos, subtitles and metadata to form a multimodal corpus, which is described in more detail Dykes/Wilson/Uhrig (2023). A short summary of the process is given in Table 1, which is taken from the paper:
On the downloaded videos, we deployed an Active Speaker Detection pipeline (ASD) to detect the host for each given scene. For ASD we used TalkNet (Tao et al. 2021), which combines visual features extracted by a convolutional neural network from the input video and audio features obtained by another convolutional network from the Mel-frequency cepstrum via a cross-attention mechanism. For the host detection task, we used biometric clustering. We split each video into scenes, tracked persons on each individual scene, and calculated the mean face vector representation for each tracked person. After that we used the HDBSCAN clustering algorithm in order to cluster the obtained vector representations. For each scene we assigned a cluster to every tracked person and calculated a centroid of each cluster and ran the DBSCAN clustering algorithm on these centroids.
To find instances when the hands of an active speaker are visible, we deploy OpenPose (Cao et al. 2018) to annotate body pose, which means that a set of so-called keypoints for every person detected on screen is created, and the results are stored as coordinates in a data structure that can then be processed further. (Uhrig et al. 2023: 2)
The output of these tools was used in two different settings. The first is via the corpus manager CQPweb, which was improved with plugins and visualizations to allow for video playback directly in the corpus interface. We can now query not only for linguistic features but also find specific people (in our case the host of the show, Sophieco Shevardnadze) and query whether we see at least one of their wrists either directly on the word or within a two-second time window around the word.
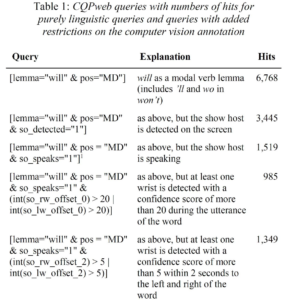
Table 2 shows how the number of hits can be massively reduced through these methods, saving large amounts of time of the human annotators, who would otherwise have to watch all video snippets and decide which ones to discard based on the speaker or the visibility of the hands.
We have created a Python package described in Burenko/Wilson/Uhrig (in prep.) which creates ELAN files with user-specified tiers and time series including annotation for hand movements, hand positions, hand movement directions and eyebrow movements. It is developed in a modular way, which allows third-party developers to contribute their own modules to the software, so that further developments in the automatic annotation of multimodal communication can be leveraged.
Examples can be seen at http://go.redhenlab.org/pgu/0135/ and at http://go.redhenlab.org/pgu/0136/. In a collaboration with researchers from the Netherlands, we also trained models for gesture segmentation (Ghaleb et al. 2024):
We have proposed a novel framework that emphasizes the structured and sequential nature of gestures, focusing on co-speech gestures in naturalistic, conversational data. Our framework reframes the gesture detection task as a multi-phased sequence labeling problem, exploiting a theoretically motivated labeling scheme that distinguishes between the preparation, stroke, and retraction phases of gesture units. Our model processes sequences of skeletal movements represented as spatio-temporal graphs over time windows, uses Transformer encoders to learn contextual embeddings, and leverages Conditional Random Fields to perform sequence labeling. In our experiments, we compare our proposed model to strong baselines. The results show that sequence labeling methods outperform classification approaches on gesture stroke detection and that the presence of the Transformer encoder improves gesture unit detection for all models, but especially for sequential prediction variants. Finally, we observed that all models are better at detecting the gesture stroke than its boundaries. Overall, our results and analyses highlight our framework’s capacity to capture the fine-grained dynamics of co-speech gesture phases. (Ghaleb et al. 2024: 4014).
This project is still ungoing under the name MultiGest24.
Publications
A. Wilson, I. Pavlova, E. Payne, I. Burenko, and P. Uhrig: World Futures through RT’s Eyes: Multimodal Dataset and Methodology. Frontiers in Communication. Section: Multimodality of Communication 9, 2024. DOI: 10.3389/fcomm.2024.1356702.
E. Ghaleb, I. Burenko, M. Rasenberg, W. Pouw, P. Uhrig, J. Holler, I. Toni, A. Özyürek, and R. Fernández: Co-Speech Gesture Detection through Multi-phase Sequence Labeling. IEEE/CVF Winter Conference on Applications of Computer Vision (WACV), 2024. DOI: 10.1109/WACV57701.2024.00396.
P. Uhrig, E. Payne, I. Pavlova, I. Burenko, N. Dykes, M. Baltazani E. Burrows, S. Hale, P. Torr, and A. Wilson: Studying time conceptualisation via speech, prosody, and hand gesture: Interweaving manual and computational methods of analysis. In W. Pouw, J. Trujillo, H. R. Bosker, L. Drijvers, M. Hoetjes, J. Holler, et al. (Eds.), Gesture and Speech in Interaction (GeSpIn) Conference. 2024. DOI: 10.17617/2.3527220.
N. Dykes, A. Wilson, and P. Uhrig: A Pipeline for the Creation of Multimodal Corpora from YouTube Videos. Proceedings of Linguistic Insights from and for Multimodal Language Processing (LIMO 2023) at KONVENS, Ingolstadt. ACL Anthology. 2024.
Life Sciences
Biology
Abstract
The understanding of the molecular details behind the regulatory mechanisms of the AMPA-type glutamate receptor (AMPAR) is of high importance. The process of cation conduction in the AMPAR ion channel offers several points of action for modulation. The divers AMPAR family exhibits in general a huge plethora of different regulations und modulations of their activity. Some examples for those effects are stabilization of the open or closed state, slow down or facilitate desensitization, influence on the conductance for calcium ions, prevent or allow the ability to be blocked by polyamines and so on. Many of these effects are mediated by auxiliary proteins which bind to the core-receptor. The atomistic details behind these interactions are not fully understood. Thus, MD Simulation represent a versatile method to investigate questions like these. In this project, we apply all-atom MD Simulations to Cryo-EM structures of the AMPAR with bound auxiliary proteins to reveal the mechanisms by which the cation gating and conduction is modulated. Our focus will lie on a subunit composition which is thought to represent a stabilized open state and a different subunit composition which has an influence on the polyamine block. We aim to elucidate molecular interactions which are crucial for AMPAR regulation.
Scientific field: 201-02 Biophysics
Principal Investigator: Prof. Dr. Andrew Plested, Humboldt-Universität zu Berlin
Target system: parallel computer Fritz
Project Report not yet available
Abstract
GPCRSIM uses classical (force-field) molecular dynamics simulations to determine binding sites, binding free energies and activation/deactivation free-energy profiles for predominantly class A G protein coupled receptors. In order to be able to observe rare events such as binding or activation, metadynamics enhanced sampling simulations employ standard optimized simulation profiles that have been developed in predecessor projects. Multiple-walker simulations ensure high parallel performance in metadynamics simulations that use standardized collective variables and funnel constraints to restrict the conformational space in the extracellular medium.
Scientific field: 201-02 Biophysics
Principal Investigator: Prof. Dr. Tim Clark, Friedrich-Alexander-Universität Erlangen-Nürnberg
Target systems: parallel computer Fritz & GPGPU cluster Alex
Project Report not yet available
Publications
J. C. Calderón, E. Plut, M. Keller, C. Cabrele, O. Reiser, F. L. Gervasio, and T. Clark: Extended Metadynamics Protocol for Binding/Unbinding Free Energies of Peptide Ligands to Class A G-Protein-Coupled Receptors. Journal of Chemical Information and Modeling 64(1), 2023. DOI: 10.1021/acs.jcim.3c01574.
Abstract
The function of immune receptors expressed on the surface of a variety of cells is typically characterized by the sensing of an external signal, followed by signal modulation and transmission into the cell. All of these steps are directly or indirectly affected by the composition, structure, and characteristics of the plasma membrane that forms the signal transmission barrier, shapes the sites for both extracellular sensing and intracellular signaling cascades. In immune cells, these two are connected by immune receptors that bridge the membrane.
Immune receptors were repeatedly reported to be localized in specific membrane domains, so called immune domains, that involve mutual interactions between the receptors, co-receptors and the lipid environment. Clearly, immune cell activation depends on and may affect this domain formation. In this project we envisage to characterize the architecture and interaction dynamics of these immune domains, and their coupling to receptor signaling.
To investigate this mutual interplay and decipher the driving forces underlying immune domain formation, we will use both coarse-grained and atomistic molecular dynamics (MD) simulations of receptors and their environment in membranes of increasing complexity, from symmetric simplified model membranes to asymmetric membranes with a composition close to the plasma membrane. We expect the simulations to yield a fingerprinting of the lipid nanometer environment of immune receptors depending on the sequence as well as on the orientation of their transmembrane (TM) region within the membrane. The composition of the immune domain composition may also influence membrane shape such as curvature, which is as well an essential factor for the efficiency of binding of large ligands or of association and clustering of receptors. The mutual influence of the composition of immune domains and signaling-required receptor assemblies on the membrane shape will be screened employing coarse-grained MD simulations of a recently developed bicelle setup which is particularly suitable to study protein- and lipid-induced membrane reshaping processes in an unbiased manner.
In the long run, combining in silico molecular scale insights of immune domain composition, structure, and dynamics with results from immune cell activation, receptor localization and membrane phase in superresolution microscopy, and proteo-lipidomics, as provided by a collaborative network of experimental labs, will yield a comprehensive view on immune cell activation and its modulating key factors.
Scientific field: 201-02 Biophysics
Principal Investigator: Prof. Dr. Rainer Böckmann, Friedrich-Alexander-Universität Erlangen-Nürnberg
Target system: GPGPU cluster Alex
Project Report not yet available
Abstract
Lipid nanoparticles (LNPs) are very successfully employed as novel transport vehicles for mRNA vaccines. A major gap in our understanding and thus obstacle for future developments of nanoparticle-mRNA drugs, however, is the lack of a molecular picture and molecular insight into LNPs. In this project we aim to provide unique insight at the atomistic scale into the structure and mechanisms of these carriers.
Scientific field: 201-02 Biophysics
University: Friedrich-Alexander-Universität Erlangen-Nürnberg
Target system: GPGPU cluster Alex
Abstract
The design of new functional proteins can tackle many of the problems humankind is facing today but so far has proven very challenging. Analogies between protein sequences and human languages have been long noted and a summary of their most prominent similarities is described. Given the tremendous success of Natural Language Processing (NLP) methods in recent years, its application to protein research opens a fresh perspective, shifting from the current energy-function centered paradigm to an unsupervised learning approach based entirely on sequences. To explore this opportunity further we have pre-trained a generative language model on the entire protein sequence space. We find that our language model, ProtGPT2, effectively speaks the protein language and can generate de-novo sequences with natural properties in a matter of seconds.
Scientific field: 201-02 Biophysics
University: Universität Bayreuth
Target system: GPGPU cluster Alex
Scientific field: 201-02 Biophysics
University: Leipzig University
Target system: parallel computer Fritz
Medicine
Abstract
The classification of chest x-rays for radiologists is a time-consuming task. Recently, neural networks have been shown to be quite useful for a first assessment of chest x-rays. For this, a large amount of annotated data is necessary. Even though there are strong efforts to anonymize the patient identity, it still may be possible to identify a patient. Thus, in this work, we will investigate the generation of synthetic chest x- rays using latent diffusion models. This way, we will be able to generate large amount of data necessary to train chest x-ray classification networks while preventing leakage of patient identity.
Scientific field: 205-01 Epidemiology, Medical Biometry, Medical Informatics
Principal Investigator: Prof. Dr.-Ing. habil. Andreas Maier, Friedrich-Alexander-Universität Erlangen-Nürnberg
Target system: parallel computer Fritz
Project Report (01/2024)
The project aimed to harness the power of advanced neural networks for the classification of MRI images in psoriatic arthritis (PsA), seronegative rheumatoid arthritis (RA), and seropositive RA. The scientific objectives were centered around the development and application of ResNet neural networks and a novel neural network for the differentiation of inflammatory arthritis types based on MRI scans and 3D articular bone shapes.
The A100 HPC Cluster provided the computational backbone for this project, enabling the training of complex ResNet models and the novel neural network on large datasets. The first part of the project used ResNet neural networks to analyze MRI scans from 649 patients, encompassing various sequences such as T1 coronal, T2 coronal, and fat-suppressed contrast-enhanced sequences. The models were tasked with distinguishing between seropositive RA, seronegative RA, and PsA based on these scans.
In the second part, a novel neural network was trained on 3D shapes of hand joints derived from high-resolution peripheral-computed-tomography (HR-pQCT) scans of 617 patients. This innovative approach focused on identifying joint shape patterns indicative of RA, PsA, or healthy controls (HC).
Achievements and Results
Classification Performance: The ResNet models achieved AUROCs of 75%, 74%, and 67% for seropositive RA vs PsA, seronegative RA vs PsA, and seropositive vs seronegative RA classifications, respectively. The novel neural network differentiated hand bone shapes with AUROCs of 82% for HC, 75% for RA, and 68% for PsA, highlighting its potential for clinical application.
Insights into Disease Patterns: The project revealed that all MRI sequences were relevant for classification, but the exclusion of contrast agent–based sequences only marginally affected performance. Additionally, psoriasis patients without clinical arthritis were often classified as PsA by the neural networks, suggesting early PsA-like MRI patterns in psoriatic disease.
Heat Maps for Clinical Application: The novel network provided heat maps identifying critical anatomical regions prone to arthritis-related changes, offering valuable insights for clinical assessments and potential early detection of disease.
The A100 HPC Cluster proved to be an invaluable resource, providing the necessary computational power to process large imaging datasets and complex neural network architectures. The computational efficiency and capacity of the cluster were sufficient to meet the project’s objectives, enabling rapid training and iteration of the models.
A significant highlight of the project was the development of the novel neural network capable of analyzing 3D joint shapes, a pioneering approach in the field of rheumatology. This advancement opens new avenues for the non-invasive and early detection of inflammatory arthritis, potentially improving patient outcomes through earlier intervention.
Publications
L. Folle, S. Bayat, A. Kleyer, F. Fagni, L. A Kapsner, M. Schlereth, T. Meinderink, K. Breininger, K. Tascilar, G. Krönke, M. Uder, M. Sticherling, S. Bickelhaupt, G. Schett, A. Maier, F. Roemer, and D. Simon: Advanced neural networks for classification of MRI in psoriatic arthritis, seronegative, and seropositive rheumatoid arthritis. Rheumatology 61(12), 2022. DOI: 10.1093/rheumatology/keac197
L. Folle, D. Simon, K. Tascilar, G. Krönke, A.-M. Liphardt, A. Maier, G. Schett, and A. Kleyer: Deep Learning-Based Classification of Inflammatory Arthritis by Identification of Joint Shape Patterns—How Neural Networks Can Tell Us Where to “Deep Dive”. Clinically. Front. Med. 9, 2022. DOI: 10.3389/fmed.2022.850552
L. Folle, P. Fenzl, F. Fagni, M. Thies, V. Christlein, C. Meder, D. Simon, I. Minopoulou, M. Sticherling, G. Schett, A. Maier, and A. Kleyer: DeepNAPSI multi‑reader nail psoriasis prediction using deep learning. Front. Med. 9, 2022. DOI: 10.3389/fmed.2022.850552
The goal of this project is to develop an unobtrusive wearable technology for long-term digestion monitoring, termed GastroDigitalShirt. We investigate low-amplitude bowel sounds (BS) as indicators of digestive disorders, including chronic inflammatory bowel diseases (IBD). We analyze methods to: (1) acquire BS in unconstrained environments (i.e. daily life of patients), (2) determine the benefit of source separation techniques to interpret BS sources, (3) spot BS patterns in continuous audio data streams, and (4) investigate how BS analysis can help patients and clinicians in the diagnosis and treatment of gut diseases.
Scientific fields: 205-01 Epidemiology, Medical Biometry, Medical Informatics; 407-06 Biomedical Systems Technology; 409-07 Computer Architecture and Embedded Systems
Principal Investigator: Prof. Dr. Oliver Amft, Friedrich-Alexander-Universität Erlangen-Nürnberg
Target system: GPGPU cluster Alex
Publications
A. Baronetto, L. S. Graf, S. Fischer, M. F. Neurath, and O. Amft: Segment-Based Spotting of Bowel Sounds Using Pretrained Models in Continuous Data Streams. IEEE Journal of Biomedical and Health Informatics 27(7), 2023. DOI: 10.1109/JBHI.2023.3269910.
Natural Sciences
Chemistry
Abstract
Covalent inhibitors currently experience a renaissance in medicinal chemistry due to their various advantages, including prolonged residence times, lower sensitivity against pharmacokinetic aspects, and high efficacy. Our work addresses the reaction mechanisms of cysteine protease rhodesain with covalent inhibitors. Our goal is to further improve the modelling of the underlying inhibition mechanisms so that we can suggest improved compounds. Furthermore, we aim to develop a protocol to treat enzyme-inhibitor reactions with various local minima.
Scientific field: 303-02 General Theoretical Chemistry
Principal Investigator: Prof. Dr. Bernd Engels, Julius-Maximilians-Universität Würzburg
Target system: GPGPU cluster Alex
Project Report not yet available
Abstract
Ethylene (C2H4) is a building block for the production of polymers. The employed catalysts used for enabling the polymerization process are sensitive to impurities. C2H4 is usually produced by cracking light alkanes and contains acetylene (C2H2) which deactivates the catalyst. Therefore, it is important to reduce the amount of it before starting the polymerization reaction. This important issue can be alleviated by selective hydrogenation of C2H2 to C2H4. In order to achieve high control over selectivity, we can employ binary and ternary transition-metal alloy surfaces as catalysts. However, there are few known alloys for such reactions. Applying only extensive computational search and/or trial and error experimental methods hinders the catalytic search. We will apply an AI tool based on compressed sensing, in conjunction with DFT calculations to identify descriptors for predicting catalytic activity and selectivity from a relatively small set of training DFT data.
Scientific fields: 303 Physical and Theoretical Chemistry; 307 Condensed Matter Physics
Principal Investigator: Prof. Dr. Matthias Scheffler, TU Berlin
Target system: parallel computer Fritz
Project Report not yet available
Physics
Abstract
CosmosTNG is a first-of-its-kind constrained cosmological hydrodynamical galaxy formation simulation. It is designed to explore the formation and evolution of galaxies within the large-scale structure of the high-redshift Universe. An observed patch of the sky is first used to reconstruct the density field of the early Universe, which is then evolved with a state-of-the- art structure formation code using the IllustrisTNG galaxy formation model. The outcome enables (i) a direct comparison between simulations and observations in the COSMOS field, (ii) a new tool to explore galaxy formation in constrained environments, and (iii) a probe of the physics and distribution of gas in and around galaxies – from the circumgalactic to intergalactic medium – at cosmic noon.
Scientific field: 311 Astrophysics and Astronomy
Principal Investigator: Dr. Chris Byrohl, Heidelberg University
Target system: parallel computer Fritz
Project Report (09/2024)
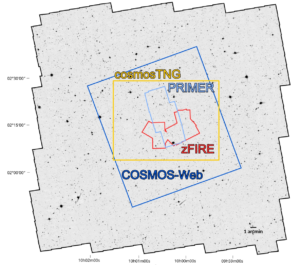
cosmosTNG is a first-of-its-kind constrained cosmological galaxy formation simulation exploring the formation processes of our Universe in its first billions of years after the Big Bang. An observed patch of the sky is first used to reconstruct the matter distribution shortly after the Big Bang, which is then evolved with the state-of-the-art structure formation code AREPO using the TNG galaxy formation model. The simulation outcomes enable a direct comparison of simulations and observations within the COSMOS field on the night sky and provide a new tool to explore galaxy formation in realistic large-scale environments, as well as a probe of the physics and distribution of gas in and around galaxies two billion years after the Big Bang. First results indicate similar outcomes to “conventional” cosmological galaxy formation simulations, albeit substantial differences for the abundance and properties of massive galaxies occur, potentially pointing at the uniqueness of the overdense COSMOS field.
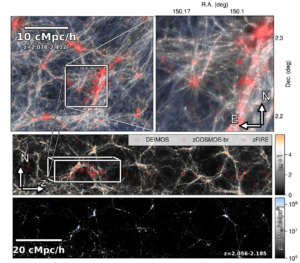
Publications
C. Byrohl, D. Nelson, B. Horowitz, K.-G. Lee, and A. Pillepich: Introducing cosmosTNG: simulating galaxy formation with constrained realizations of the COSMOS field. arXiv, 2024. DOI: 10.48550/arXiv.2409.19047.
Abstract
Many astrophysical environments, from stellar clusters to disks of active galactic nuclei, are characterized by frequent interactions between stars and stellar-mass compact objects. In particular, encounters involving three objects (three-body encounters) are frequent in dense stellar environments. Previous work on such encounters has mostly focused on understanding the orbit evolution and outcome assuming the encountering objects are point particles. However, the point-particle approximation cannot be used to study observable phenomena produced in those interactions where the size of the meeting objects and gas interactions between them become an essential role in generating electromagnetic radiation. Such phenomena include tidal disruption events of stars by black holes, which can have a significant impact on the black hole mass growth and the subsequent long-term interactions with the surrounding debris. To investigate the impact and outcomes of dynamical interactions we perform hydrodynamics simulations of various types of three-body encounters using the moving-mesh magnetohydrodynamics code AREPO.
Scientific field: 311 Astrophysics and Astronomy
Principal Investigator: Prof. Dr. Volker Springel, Ludwig-Maximilians-Universität München
Target system: parallel computer Fritz
Project Report (07/2024)
Many astrophysical environments, from stellar clusters to disks of active galactic nuclei, are characterized by frequent interactions between stars and stellar-mass compact objects. In particular, encounters involving three objects (three-body encounters) are frequent in dense stellar environments. Previous work on such encounters has mostly focused on understanding the orbit evolution and outcome assuming the encountering objects are point particles. However, the point-particle approximation cannot be used to study observable phenomena produced in those interactions where the size of the encountering objects and gas interactions between them become an essential role in generating electromagnetic radiation. Such phenomena include tidal disruption events of stars by black holes, which can have a significant impact on the black hole mass growth and the subsequent long-term interactions with the surrounding debris.
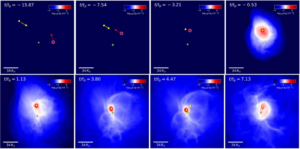
To investigate the impact and outcomes of dynamical interactions we perform hydrodynamics simulations of various types of three-body encounters using the moving-mesh magnetohydrodynamics code AREPO. We found that three-body encounters can produce varieties of outcomes, some of which are observable transients, such as stellar disruption, collisions between stars, and collisions between stars and black holes. See Figure 1 as an example.
The two decisive parameters for determining the outcomes are the binary phase angle, determining which two objects meet at the first closest approach, and the impact parameter, demarcating the boundary between violent and non-violent interactions. When the impact parameter is smaller than the binary semimajor axis, tidal disruptions and star-black hole collisions frequently occur, while the two stars mostly merge when the two stars meet first instead. In both cases, the black holes typically are surrounded by an optically and geometrically thick accretion disk and accrete at super-Eddington rates, possibly generating luminous flares. The stellar collision products either form a binary with the black hole or remain unbound to the black hole. Upon collision, the merged stars are hotter and larger than main sequence stars of the same mass at similar age. Even after recovering their thermal equilibrium state, stellar collision products, if isolated, would remain hotter and brighter than main sequence stars until becoming giants.
Project Accomplishments
The overarching goal of the proposed research program is to conduct detailed hydrodynamics calculations for multi-body encounters. Specifically, the three main objectives are 1) to identify types of outcomes, 2) estimate their observational signature, and 3) improve our current understanding based on the point-particle approximation.
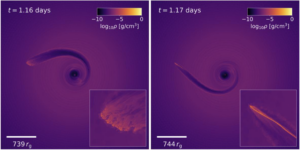
The three most significant impacts of the projects are the identification of transients produced in various types of three-body encounters and their classifications, and the examination of observational features of potentially observable transient phenomena. More specifically, we were able to identify the ranges of key parameters where observable transients can be potentially created and the dependence of outcome properties on encounter parameters. In addition, the simulations of tidal disruption events in active galactic nuclei (Ryu, McKernan, Ford et al. MNRAS 527, 8103 (2024)) was the first global simulations of tidal disruption events in gaseous environments where the initial approach of the star was successfully modelled. In that paper, we found the characteristic density above which the evolution of debris becomes qualitatively different from those in gas-free tidal disruption events, which includes the mixing of debris into the disk as depicted in Figure 2. Our semi-analytic model for debris evolution and the characteristic density can be used to place constraints on the density of active galactic nuclei disks, which otherwise is hard to achieve.
We made two significant improvements from previous work. First, the hydrodynamics scheme adopted for our simulations overcomes numerical limits of widely used methods. The hydrodynamics code AREPO adopts the moving-mesh approach which inherits advantages of the Eulerian finite-volume method and the Lagrangian smoothed particle method. This allows us to account for shocks and mixing more accurately. Second, we adopt realistic initial conditions. The initial state of the stars has been often approximated as the solution of the gravitational Poisson’s equation for polytropic gas in hydrostatic equilibrium even though those models sometime show significant discrepancies from detailed stellar evolution models. In our projects, the initial state of the stars was taken from stellar models generated using the stellar evolution code MESA.
In this project, we have published five papers published in a peer-reviewed journal (see Publications below).
The computation resources were sufficiently large to complete the proposed projects. The code speed with AREPO was much faster than that on other clusters that PI have been running simulations on, which facilitated the progress of the projects substantially.
Publications
T. Ryu, R. Perna, R. Pakmor, J.-Z. Ma, R. Farmer, and S. de Mink: Close encounters of tight binary stars with stellar-mass black holes. MNRAS 519(4), 2023. DOI: 10.1093/mnras/stad079
T. Ryu, R. Valli, R. Pakmor, R. Perna, S. de Mink, and V. Springel: Close encounters of black hole—star binaries with stellar-mass black holes. MNRAS 525(4), 2023. DOI: 10.1093/mnras/stad1943
T. Ryu, S. de Mink, R. Farmer, R. Pakmor, R. Perna, and V. Springel: Close encounters of star–black hole binaries with single stars. MNRAS 527(2) 2024. DOI: 10.1093/mnras/stad3082
T. Ryu, B. McKernan, S. Ford, M. Cantiello, M. Graham, D. Stern, and N. Leigh: In-plane tidal disruption of stars in discs of active galactic nuclei. MNRAS 527(3), 2024. DOI: 10.1093/mnras/stad3487
T. Ryu, P. Amaro Seoane, A. Taylor, and S. Ohlmann: Collisions of red giants in galactic nuclei. MNRAS 528(4), 2024. DOI: 10.1093/mnras/stae396
Abstract
The High Energy Stereoscopic System (H.E.S.S.) telescopes in Namibia observe the very-high-energy gamma-ray sky from 20 GeV to 10s of TeV. H.E.S.S. operates by observing the Cherenkov light emitted when such a gamma-ray creates a particle cascade in the atmosphere; the nature, origin and energy of the incident particle can be determined using image processing techniques. State-of-the-art deep learning methods allow for high-sensitivity image analysis at high-speed, making them an attractive analysis prospect for H.E.S.S.. With this project, we aim to develop a variety of new analysis methods for H.E.S.S. data, including improved identification of muonic events, exploration of geometric deep learning techniques, fast simulation approaches and new data augmentation strategies.
Scientific fields: 311-01 Astrophysics and Astronomy; 309-01 Particles, Nuclei and Fields
Principal Investigator: Dr. Samuel Spencer, Friedrich-Alexander-Universität Erlangen-Nürnberg
Target system: GPGPU cluster Alex
Project Report (01/2024)
Imaging Air Cherenkov Telescopes (IACTs) are fundamental to ground-based observations of the very high-energy sky. To perform ground-based gamma-ray astronomy, an effective rejection of the hadronic background is pivotal. Within the current research project, we are investigating algorithms based on state-of-the-art machine-learning techniques to improve instrument resolution and sensitivity.
We proposed a new deep-learning-based algorithm for classifying images by interpreting the detected images as sets of triggered sensors, which are then represented as graphs and analyzed using graph convolutional networks. This innovative method, particularly effective for images cleaned of night sky light, circumvents challenges associated with sparse images encountered in traditional deep learning techniques like convolutional neural networks. Our exploration of various graph network architectures has yielded promising results, showcasing improvements over existing machine-learning and deep-learning-based methods. This memory-efficient approach has promising potential to be adapted to IACT arrays with a large number of telescopes.
For precise observations using IACTs the instrument response has to be derived, requiring large simulation libraries. These simulations, characterized by computationally and memory-intensive calculations, are repeatedly performed for different observation intervals to account for variations in the optical sensitivity of the telescopes. Leveraging generative models based on deep neural networks, specifically Wasserstein Generative Adversarial Networks, we propose an efficient method for storing extensive simulation libraries in a memory-efficient manner and rapidly generating simulations. In our study, we applied this approach to a state-of-the-art IACT camera featuring over 1,500 pixels, and demonstrated the successful generation of high-quality images with high fidelity with respect to important physical parameters. Notably, the substantial increase in simulation speed, on the order of 100,000, holds promising implications for efficient and fast simulations for IACTs.
The project is still ongoing under the name: GAMMAML.
Publications
J. Glombitza, V. Joshi, B. Bruno, and S. Funk: Application of graph networks to background rejection in Imaging Air Cherenkov Telescopes. Journal of Cosmology and Astroparticle Physics 11, 2023. DOI: 10.1088/1475-7516/2023/11/008.
C. Elflein, S. Funk, and J. Glombitza: Ultra-fast generation of air shower images for Imaging Air Cherenkov Telescopes using Generative Adversarial Networks. Journal of Instrumentation 19, 2024. DOI: 10.1088/1748-0221/19/04/P04010.
Abstract
KM3NeT/ORCA is a neutrino telescope currently under construction in the Mediterranean deep sea. It detects neutrinos of all flavors produced in the atmosphere indirectly by measuring the Cherenkov light emitted when fast charged particles are produced during neutrino-nucleon interactions. One of the scientific goals of the KM3NeT Collaboration is to further constrain the least-well-known elements of the three-flavor leptonic mixing matrix. To this end, we will in particular seek to identify tau neutrino interactions in the detector volume, a task known as tau neutrino event identification. A new approach to this problem is studied in this project, where state-of-the-art Graph Neural Networks are trained to directly identify tau neutrino events in the detector data. The promising results achieved in first trainings will be further improved in this NHR project by conducting a systematic search for the optimal hyperparameters of the networks, a task known as automatic hyperparameter optimization.
Scientific field: 309-01 Nuclear and Elementary Particle Physics, Quantum Mechanics, Relativity, Fields
Principal Investigator: PD Dr. Thomas Eberl, Friedrich-Alexander-Universität Erlangen-Nürnberg
Target system: GPGPU cluster Alex
Project Report (11/2023)
Different optimization steps for training Graph Neural Networks (GNNs) on the task of tau neutrino event identification in KM3NeT/ORCA were performed. The last optimization step consisted of an automatic hyperparameter optimization (AHPO) that systematically searched through the hyperparameter space by stepwise parameter selection and subsequent automated training of the resulting GNNs. The chosen hyperparameters, various metrics that were monitored during training, and the final performance metric of the GNNs were logged. In total, about 2000 GNNs could be trained with the 46000 GPU-hours of computing time granted for this project. The data from this AHPO was used to identify the most significant hyperparameters, i.e., the hyperparameters whose values have the greatest influence on the resulting performance of the GNNs.
The most significant hyperparameter was identified to be the learning rate. The boxplot on the left shows the distribution of the value of the performance metrics over many trained GNNs for learning rates in different ranges. It indicates that learning rates between 10-3 and 10-2 result in the best-performing GNNs. Analogous investigations were done for many other hyperparameters in the GNN architecture, demonstrating which of these are essential and which are of less significance for the performance in the studied classification task. Importantly, this systematic analysis a-posteriori confirmed the general, best-guess hyperparameter choices that were made in previous KM3NeT studies, published in two dissertations and several conference proceedings, using very similar GNN architectures for classification and regression tasks. Due to a lack of corresponding resources within the international KM3NeT Collaboration, this important systematic study could not be done earlier. Another goal of the AHPO was to achieve a performance gain in classification compared to the first-step manual optimizations done earlier. However, the AHPO did not show a significant performance gain over the manual optimizations. Presumably, the reason is that the GNN architecture was already well-optimized due to its use in previous studies. Finally, using the insights, strategies, and methods developed during this project for future architectures and applications in the analysis of neutrino telescope data, more efficient, faster and more trustable optimizations will be possible.
The resources granted to this project have been sufficient to achieve its goals. The baseline target was to train between 1000-1500 different GNNs, but the resources turned out to be even enough to train about 2000 GNNs. This number of GNNs allowed us to compute a large enough sample to draw statistically relevant conclusions on the significance of most of the probed hyperparameters. The AHPO ran for most of the time in parallel on 64 GPUs, i.e., on eight computing nodes with eight GPUs per node.
Publications
The accomplishments of this project are documented in the master’s thesis of Lukas Hennig.
Abstract
High precision calculations from lattice QCD are needed nowadays in many respects. In order to obtain such results it is mandatory to have good control over all systematic effects involved in such calculations. In this study we plan to investigate the systematic effects introduced by the finite volume of the system, which is important in particular for quantities like low energy constants, pseudoscalar masses and decay constants, or the axial charge of the nucleon.
Scientific field: 309 Particles, Nuclei and Fields
Principal Investigator: PD Dr. Wolfgang Söldner, University of Regensburg
Target system: parallel computer Fritz
Project Report (01/2024)
Within the past NHR project we have made another great step forward in our large scale project of computing important matrix elements related to Beyond-the-Standard-Model (BSM) interactions (which are not accessible experimentally) and (lower) moments of the parton distribution functions. These computations are of great interest for current and upcoming experiments (like the Electron Ion Collider) with respect to nucleon structure. Utilizing the granted NHR resources we could improve on our statistical and systematic uncertainties in a particular relevant region in the parameter space that enters our fits from which we extract the final (physical) matrix elements.
Scientific field: 307-02 Theoretical Condensed Matter Physics
University: RWTH Aachen
Target system: parallel computer Fritz
Scientific field: 307-02 Theoretical Condensed Matter Physics
University: Julius-Maximilians-Universität Würzburg
Target system: parallel computer Fritz
Engineering Sciences
Thermal Engineering/ Process Engineering
Abstract
In this project, numerical studies are carried out to investigate the influence of surface modifications on the near-wall turbulence of external and internal wall-bounded flows. The surface modifications consist of streamwise aligned grooves in combination with regions of strong transverse curvature between the grooves. The aim of the surface modifications is to reduce the near-wall turbulent fluctuations that can lead to flow-induced vibrations of the adjacent structure and/or to flow-induced sound which is undesired in many applications. Recent investigations show that such surface modifications could lead to a 20 % decrease in the average wall shear stress and result in local reductions in the turbulent intensities, Reynolds stress, the temporal velocity spectrum, and the turbulent dissipation rate. The analysis within the anisotropy-invariant space revealed a local tendency towards flow relaminarization. Improvements of the surface modifications should be investigated in a numerical study using the finite volume code OpenFOAM. The size of the improved surface modifications are in the order of the viscous sublayer. Transient and scale-resolving simulations are needed to extraxt the quantities of interest, such as turbulent dissipation, anisotropy of turbulence, Reynolds stress, flow-acoustic source terms, velocity and pressure fluctuations as well as temporal spectra. Therefore a high-resolution mesh in combination with multi-node parallelized simulations are required.
Scientific fields: 404-03 Fluid Mechanics; 402-04 Acoustics
Principal Investigator: apl. Prof. Dr.-Ing. habil. Stefan Becker, Friedrich-Alexander-Universität Erlangen-Nürnberg
Target system: parallel computer Fritz
Project Report (09/2024)
When fluid, like water or air, flows along solid walls, a boundary layer develops due to the no-slip condition at the wall. If the Reynolds number is sufficiently high, the boundary layer becomes turbulent. The turbulent fluctuations in the turbulent boundary layer (TBL) can induce vibrations in the adjacent structure (wall) via fluctuating wall pressure or wall shear stress. Such an excited vibrating structure can be an efficient source of sound. However, the turbulent pressure and/or velocity fluctuations within the TBL can themselves contribute to flow-acoustic sources. In general, flow- induced sound is undesirable in several applications. One example is air-flow-induced sound emitted into aircraft cabins, train cabins or cars, which can be unpleasant for the passengers. Another example is unwanted water-flow-induced sound in applications like sonar devices mounted within the hull of a ship or hydro-acoustic linear antennas in towed streamers (towed array sonars, side-scan sonar). For sonar devices, the flow-induced sound is unfavorable for its signal-to-noise ratio and thus limits the performance of the system. One way to suppress or decrease the level of such undesirable flow- induced sound is to suppress or decrease the origin of the problem, namely the near-wall turbulence. The main focus of the project was on applications like towed streamers. With the motivation to improve its signal-to-noise ratio, the aim of the work was to design a surface modification for the device that influences the attached turbulent boundary layer to reduce the near-wall turbulence. The investigated surface modification consists of regularly spaced streamwise-aligned grooves. The regions between the grooves are covered by convex regions with strong transverse curvatures, which have small radii on the order of the viscous sublayer. Both features of the modification should lead to a flow stabilization. Parallelized, wall-resolved large-eddy simulations were carried out with the open- source software OpenFOAM to analyse the effect of the surface modification on the TBL. The results show that although the radii of the convex regions are decreased to the order of the viscous sublayer to ensure strong convex transverse curvatures, the convex regions show no tendency of the desired flow stabilizing effect. Furthermore, the pressure fluctuation profiles reveal that the surface modification is
only able to reduce the near-wall pressure fluctuations in a negligible manner. However, the present numerical study shows that the surface modification is capable to decrease the overall wall shear stress and friction factor by 33%. The longitudinal grooves are effective in reducing the near-wall turbulence. In the grooves, a tendency of local relaminarisation is observable, and the profiles reveal that the Reynolds stress and turbulent production are locally suppressed. Furthermore, the grooves show flow stabilizing tendencies also in the anisotropy-invariance map. The turbulent dissipation is reduced to zero at the walls in the grooves, and the velocity spectra show an energy reduction over several orders of magnitude in the low to moderate frequency range. Furthermore, the areas beneath the turbulent production and dissipation rate profiles indicate that the surface modification leads to an overall reduced turbulence. Thus, the surface modification of the present study with its smaller structures leads to an improved performance in comparison to the surface modification with coarser structures analysed in a previous study (Wachter, F., Metelkin, A., Jovanović, J., Praß, J., Becker, S, 2021. Numerical investigation of the effect of convex transverse curvature and concave grooves on the turbulent boundary layer along a cylinder in axial flow. Int. J. Heat Fluid Flow 92, 108855.)
The cost of energy produced by wind turbines has been undergoing a steady reduction. Wind energy supplied 15% of the electricity demand of the European Union in 2019. Since rotor blades are the determining component for both performance and loads, they are the objective of further optimizations. To obtain high efficiencies, an increased use of special aerodynamic profiles is observed possessing large areas of low-resistance, which means laminar flow is maintained. In order to design such profiles, it is necessary to include the laminar-turbulent transition in CFD simulations of wind turbine blades. Thus, the objective of the project is to carry out high-fidelity numerical simulations of the flow around a wind turbine blade at a realistic Reynolds number to get a deeper insight into this phenomenon and especially the transition process under different levels of the turbulence intensities of the approaching flow.
Scientific field: 404-03 Fluid mechanics
University: Helmut Schmidt University Hamburg
Target system: parallel computer Fritz
Scientific field: 404-03 Fluid mechanics
University: University of Bremen
Target system: GPGPU cluster Alex
Material Science and Engineering
Abstract
Single-crystal Ni-based superalloys play a key role for the efficiency of gas turbines for aviation and power generation. The optimization of these high-performance structural materials requires an understanding of the influence of the elements of the microstructure on the mechanical properties. The goal of this project is to understand dislocation mobility and the formation of local defect phases in the microstructure. The atomistic simulations involve high-throughput ab-initio calculations and large-scale molecular-dynamic simulations with machine-learning potentials based on the atomic cluster expansion.
Scientific field: 406 Materials Science
Principal Investigator: PD Dr. habil. Thomas Hammerschmidt, Ruhr-Universität Bochum
Target system: parallel computer Fritz & GPGPU cluster Alex
Project Report (06/2024)
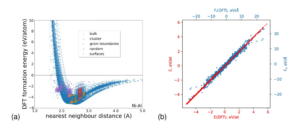
Single-crystal Ni-based superalloys play a key role for the efficiency of gas turbines for aviation and power generation. The base system of Ni-based superalloys is an fcc single-crystal with regions of L12 ordered Ni3Al (γ’ particles) embedded in a matrix of disordered Ni-Al (γ channels). This microstructure is the basis for the unusual hardening of superalloys at high temperatures that rules them one of the few materials that can withstand the extreme conditions in the hottest part of a gas turbine. Despite decades of research, there is still a lack of understanding of the complex dislocation processes at the atomistic scale that govern the plastic deformation. This can be attributed to the antipodal requirements of a sufficiently accurate description of the interatomic interactions on one hand and a sufficiently efficient method to handle large length-scales and long simulation-times on the other hand. The central activity of this project was the development of a machine-learning interatomic potential based on the atomic cluster expansion (ACE) for the Ni-Al system that captures the interatomic interaction with high accuracy at rapid execution times (Fig. 1). The construction of the ACE model for Ni-Al is based on an extensive campaign of high-throughput density-functional theory calculations for a large set of Ni, Al and Ni-Al structures that cover a broad variety of local atomic environments.
Accomplishments
The basis for the development of ACE models are density-functional theory (DFT) calculations with the VASP software package that were carried out using the projector-augmented wave method with the PBE exchange-correlation functional in the first stage of the project. The set of structures samples a large space of local atomic environments that is necessary for a stable interpolation of the DFT data by the ACE. This set of reference data will be calculated by DFT in the first stage of this project and used to parameterize ACE models for Ni, Al and Ni-Al in the second stage of the project.
The DFT calculations were running on CPUs using single nodes with 72cores which was in nearly all cases sufficient to complete the calculations in the 24 hours of queue time. The ACE parameterizations were running on A40 and A100 GPUs and partly required restarts due to time outs within 24 hours.
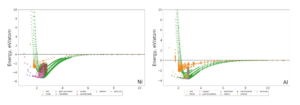
The DFT results are compiled in Fig. 1 for binary structures of Ni-Al and in Fig. 2 for unary structures of Ni and Al. All sets include a broad variety of unary or binary structures in order to achieve an extensive sampling of local atomic environments as prerequisite for constructing robust ACE models. The color coding represents structures of bulk crystal-structures of known prototypes and of random unit cells, point defects like vacancies and interstitials, grain boundaries, stacking faults, surfaces and numerous clusters of different size. The structures are considered at different unit cell scalings to map out a broad variety of bond lengths in the interatomic interactions.
The DFT results are used to parameterize the ACE models that provide an interpolation of the DFT energy landscape and calculations at much lower computational cost. In the initial step, the complexity and the range of the ACE models was optimized by performing a series of fits with different size of the ACE basis and different cutoff radius. The quality of these individual fits was compared in terms of the root-mean square error (RMSE) on the DFT reference energies as shown in Fig. 3.
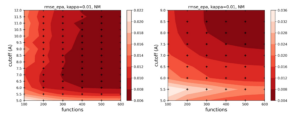
Using the identified basis-set size and cutoff, the ACE models are parameterized for the DFT reference data above. Examples of the performance of the ACE models are shown for Ni-Al in Fig. 1 and for Ni and Al in Fig. 4 and 5, respectively.
These ACE models exhibit very high predictive power with an RMSE on the energies of the order of few meV/atom over a diverse set of structures and a large range of formation energies. The development of the ACE models for the elements Ni, Al and Ni-Al is currently taken further by validations and by extending the DFT data set by active learning and by iterative improvements of the ACE parameterization. The ACE models developed in this project pave the way to investigations of the complex finite-temperature simulations of the different elements of the microstructure that are planned in future projects.
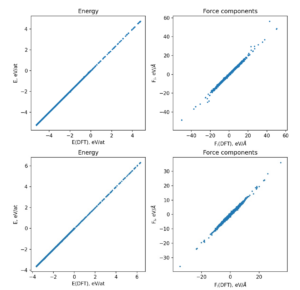
The granted HPC resources were furthermore used as requested in the proposal for DFT calculations of the thermodynamic driving force for local phase transformations in L12-Ni3Al. These calculations included different arrangements of different alloying elements superlattice intrinsic stacking faults in L12-Ni3Al (not shown in detail here).
The resources have been sufficient to reach the planned project objectives. The granted bonus resources made it possible to address also additional small side projects that came up in collaborations within the SFB/TR103 project that was the motivation of this HPC project with pre-existing review.
Particularly noteworthy seems the fact that the calculations carried out in this project formed the basis for a new proposal for an SFB/TR103 transfer-project on running gas turbines with fuels based on hydrogen. The proposal on this important backup-technology for renewable energy sources with experimental colleagues and a company for computational materials science in Sweden (ThermoCalc) was recently reviewed in a scientific colloquium and recommended for funding (final decision expected in June).
Publications
There are several publications currently written and further planned that used the granted NHR@FAU HPC resources. The acknowledgment texts will be included.
Computer Science, Electrical and System Engineering
Abstract
This project aims at exploring the potential of Convolution Neural Networks (CNNs) and Vision Transformers (ViTs) in two fundamental tasks of computer vision, i.e., handwriting analysis, object tracking and segmentation.
Handwriting document analysis aims to evaluate and recognize the handwritten manuscripts according to different intentions, such as text recognition, spotting, layout analysis, text alignment, and writer recognition. As an important issue in the first step of digitizing scanned documents, this project will focus on line segmentation and text recognition.
Object tracking and segmentation aims at continuously estimating the state of an object based on a given bounding box extracted by a simple rectangle/mask from the initial frame of a video sequence. It is widely applied in various applications such as surveillance, autonomous driving, human-computer interaction, etc. Despite the progress made so far, its main challenge lies in the limited discriminative power of the classifiers. Also, it is prone to introduce endless distractors in real-world surveillance applications. This project will investigate state-of-the-art algorithms for achieving accurately and stably object tracking and segmentation.
Scientific field: 409-05 Interactive and Intelligent Systems, Image and Language Processing, Computer Graphics and Visualisation
Principal Investigator: Fei Wu, Ph.D., Friedrich-Alexander-Universität Erlangen-Nürnberg
Target system: GPGPU cluster Alex
Project Report (11/2024)
This project focuses on handwritten document layout segmentation and glacier segmentation.
As an important issue in the first step of digitizing scanned documents, historical document layout analysis is essential for downstream tasks, e.g., optical character recognition (OCR), document categorization, and automatic transcription. Historical handwritten documents are more challenging than machine-printed documents as they are unconstrained, heterogeneous and often have complex layouts. In this project, we propose a lightweight CNN-based segmentation framework for handwriting layout analysis. Comprehensive experimental evaluation verifies the effectiveness of the proposed method.
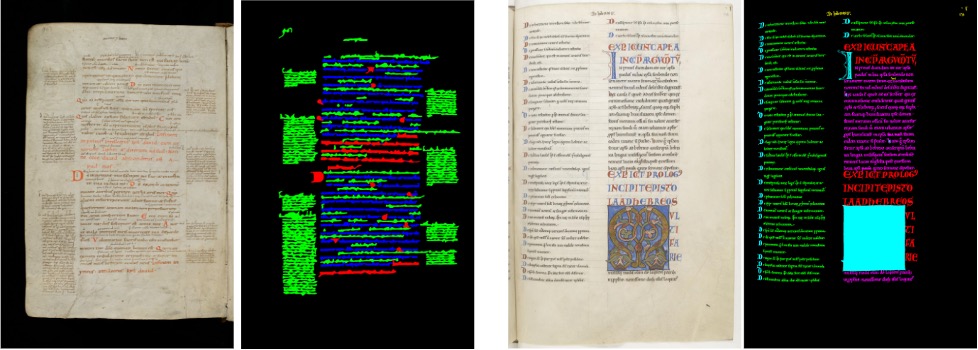
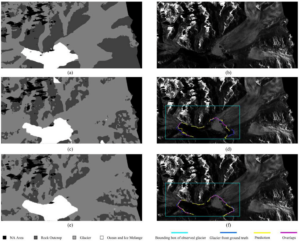
Position changes of glacier calving fronts are important indicators for evaluating the health of ice sheet outlet glaciers and changes in ice dynamics. However, manual delineation of calving fronts in remote sensing imagery is a time-consuming task, resulting in potential large costs. Deep learning-based methods have made remarkable progress in automatically segmenting and delineating glacier calving fronts from remote sensing imagery. The relatively few remote sensing images and the limited geometric changes for glacier observations both reduce the diversity of the data and exacerbate the difficulty of accurate segmentation. In this project, we describe a novel ViT-based method for detecting glacier calving fronts in synthetic aperture radar (SAR) images. We conduct an in-depth analysis and make extensive comparisons based on the challenging glacier segmentation benchmark dataset, the accordingly experimental results reveal that the proposed method achieves state-of-the-art performance.
Publications
F. Wu, N. Gourmelon, T. Seehaus, J. Zhang, M. Braun, A. Maier, and V. Christlein: Contextual HookFormer for Glacier Calving Front Segmentation. IEEE Transactions on Geoscience and Remote Sensing 62, 2024. DOI: 10.1109/TGRS.2024.3368215.
F. Wu, N. Gourmelon, T. Seehaus, J. Zhang, M. Braun, A. Maier, and V. Christlein: AMD-HookNet for Glacier Front Segmentation. IEEE Transactions on Geoscience and Remote Sensing 61, 2023. DOI: 10.1109/TGRS.2023.3245419.
F. Wu, J. Zhang, Z. Xu, A. Maier, and V. Christlein: Updating Siamese trackers using peculiar mixup. Applied Intelligence 53, 2023. DOI: 10.1007/s10489-023-04546-z.
A. Mattick, M. Mayr, M. Seuret, F. Kordon, F. Wu, and V. Christlein: Evaluating learned feature aggregators for writer retrieval. International Journal on Document Analysis and Recognition 21, 2024. DOI: 10.1007/s10032-024-00482-x
In Review
F. Wu, M. Seuret, M. Mayr, F. Kordon, J. Zöllner, S. Wind, A. Maier, and V. Christlein: Lightweight Cross-Attention-based HookNet for Historical Handwritten Document Layout Analysis. International Journal on Document Analysis and Recognition.
F. Wu, J. Zhang, D. Liu, A. Maier, and V. Christlein: Deep Learning-based Debris Flow Hazard Detection and Recognition System: A Case Study. Scientific Reports.
N. Gourmelon, K. Heidler, E. Loebel, D. Cheng, J. Klink, A. Dong, F. Wu, N. Maul, M. Koch, M. Dreier, D. Pyles, T. Seehaus, M. Braun, A. Maier, and V. Christlein: Comparison Study: Glacier Calving Front Delineation in Synthetic Aperture Radar Images With Deep Learning. IEEE Transactions on Pattern Analysis and Machine Intelligence.
M. Dreier, M. Koch, N. Gourmelon, N. Blindow, D. Steinhage, F. Wu, T. Seehaus, M. Braun, A. Maier, and V. Christlein: Ice Anatomy: A Benchmark Dataset and Methodology for Automatic Ice Boundary Extraction from Radio-Echo Sounding Data. Earth System Science Data.
Abstract
XXL-Computed Tomography (XXL-CT) is able to produce large scale volume datasets up to and above 10,0003 voxels which can relate up to many terabytes in file size and can contain multiple 10,000 of different entities of depicted objects with varying properties (shapes, densities, materials, compositions) which are hard to delineate. This project aims to develop deep learning based instance segmentation algorithms to delineate these entities for the data exploration process.
Scientific field: 409-05 Interactive and Intelligent Systems, Image and Language Processing, Computer Graphics and Visualisation
Principal Investigator: PD Dr. Thomas Wittenberg, Friedrich-Alexander-Universität Erlangen-Nürnberg
Target system: GPGPU cluster Alex
Project Report not yet available
Abstract
As a flexible and contact-free joining technology, laser beam welding has increasingly gained importance. Processing of alloys with large melting range poses a challenge due to their solidification cracking tendency. Solidification cracks form due to critical stress and strain states of the dendritic microstructure with interdendritic melt. Despite the high industrial relevance, there are only approaches addressing single aspects of the problem, metallurgically or structurally oriented. The research unit “Solidification Cracking during Laser Beam Welding—High Performance Computing for High Performance Processes” aims at developing quantitative process understanding of the mechanisms of solidification cracking and their relation to process parameters. The sub-project aims at simulating the dynamics of the melt pool with a resolution of about one micron. This is achieved using a numerical model consisting of approximately 109 computational cells that have to be computed for more than 105 time steps. The simulation will model the phase change at melting and solidification, the energy input from the laser, the expansion and contraction, and the heat and mass transport in the melt pool. Simulations of such complexity require the computational power of parallel high-performance systems and can only be realized using optimized parallel algorithms and modern software technologies. In this sub-project, the lattice Boltzmann method (LBM) will be used and extended to correctly capture the various physical effects in the melt pool. Compared to other numerical schemes, the LBM is well-suited for parallel computing and modern computer architectures that include hardware accelerators. The implementation will be based on the HPC framework waLBerla specifically designed for implementing complex multi-physics applications. Using abstraction layers and code generation concepts, software developed with waLBerla is sustainable, i.e., portable to future computer architectures. One of the significant aspects of this project lies in the validation of newly implemented models and algorithms, and in the interoperability with models from the partners in the research unit. This will be achieved via the close cooperation with neighboring sub-projects.
Scientific field: 409-08 Massively Parallel and Data-Intensive Systems
Principal Investigator: Prof. Dr.-Ing. Harald Köstler, Friedrich-Alexander-Universität Erlangen-Nürnberg
Target system: GPGPU cluster Alex
Project Report (09/2024)
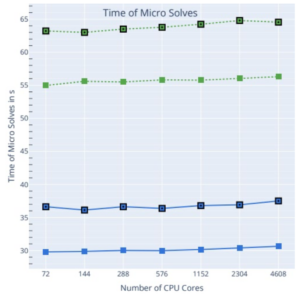
Motivation—For scientific software, especially those used for large scale-simulations, achieving good performance and efficiently using the available hardware resources is essential. It is important to regularly perform benchmarks to ensure the efficient use of hardware and software when systems are changing and the software evolves. As such we used the compute hours on Fritz to verify findings via our continuous benchmarking pipeline and weak scaling performance benchmarks and analysis.
Methods and frameworks used—For the verification of the performance findings during continuous benchmarking, for FE2TI and waLBerla, scaling runs on Fritz were necessary. FE2TI is a scalable implementation of the FE^2 method using C/C++, which is used to simulate small and large deformations in the field of solid mechanics. WaLBerla is a multiphysics HPC C++ framework for massively parallel simulations, including rigid particle dynamics, phase-field simulations, and coupled algorithms like the free-surface lattice Boltzmann method (FSLBM).
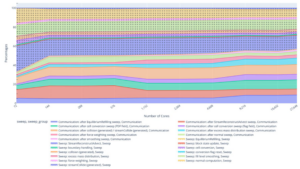
Results—For the FE2TI software, we found out in the continuous benchmarking that the fastest solution is to use an inexact solver for solving the micro problems and showed that this also holds for runs with up to 4608 CPU cores on Fritz, see the weak scaling results in Fig. 1. For waLBerla we also did weak scaling benchmarks and further performance analysis for the FSLBM implementation in waLBerla (see Fig. 2). To be specific, we looked at every part of the FSLBM algorithm and analyzed how the different parts are contributing to the total time to solution. This showed that the different parts of the algorithm do scale, but the overall algorithm shows further optimization potential which is currently investigated.
Publications
C. Alt, M. Lanser, J. Plewinski, A. Janki, A. Klawonn, H. Köstler, M. Selzer, and U. Rüde: A continuous benchmarking infrastructure for high-performance computing applications. International Journal of Parallel, Emergent and Distributed Systems, 39(4), 2004. DOI: 10.1080/17445760.2024.2360190.
J. Plewinski, C. Alt, H. Köstler, and U. Rüde: Performance Analysis of the Free Surface Lattice Boltzmann Implementation in waLBerla. Proceedings in Applied Mathematics and Mechanics (PAMM), 2024. accepted